AI Vehicles Could Improve Transportation in Rural Areas
Category: AI insights
Published date: 21.07.2023
Read time: 6 min
Current public transportation in rural areas is often inadequate due to the lack of appropriate infrastructure, limited bus schedules, and bus stops, and lack of information about bus schedules and fares. One of the new proposed ways of dealing with this problem is to implement AI-powered autonomous vehicles, like buses and shuttles and buses, in rural communities. In this article, we will explore this new solution and take a look at the data annotation needed to create these vehicles.
Improving Accessibility to Transportation
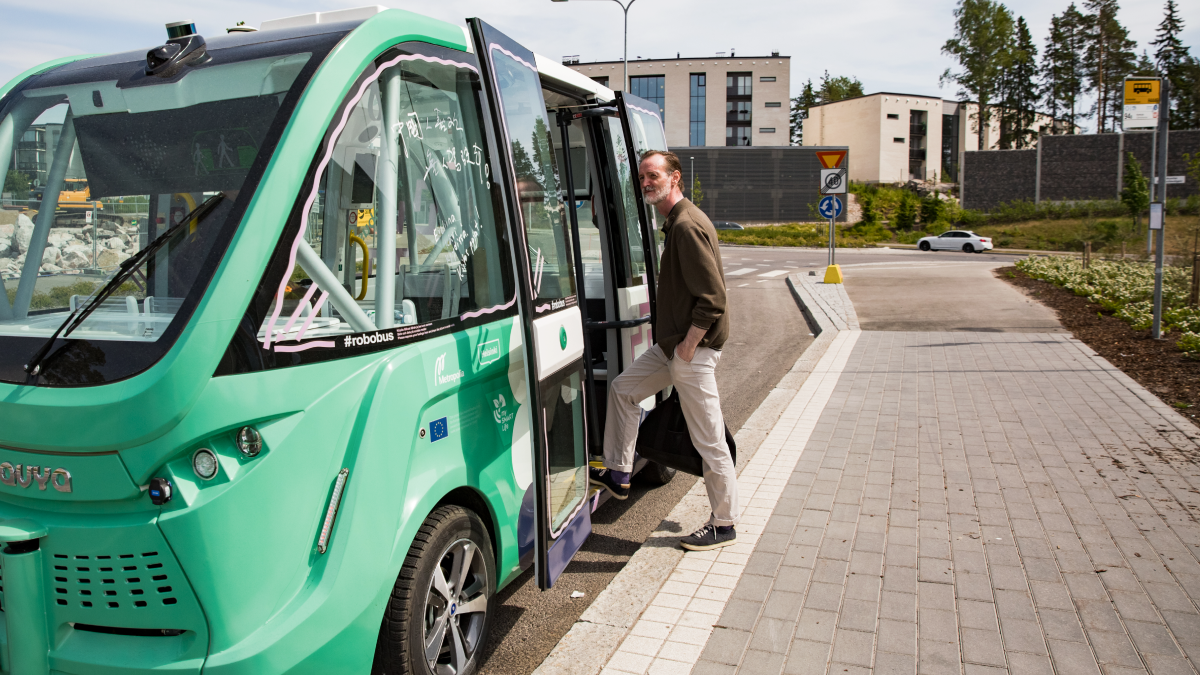
Rural residents require dependable transportation in order to access social services, employment possibilities, consumer services, healthcare services, and educational and training opportunities. Accessing recreation and other daily activities needs it as well. However, very often, public transportation is unavailable or difficult to access, and rural community members may not be able to receive important services. Barriers to transportation can result in missed healthcare appointments, delays in receiving healthcare interventions, and missed or delayed use of needed medications—all of which may have negative consequences for managing health conditions.
AI-powered buses can help provide greater access to transportation by providing a mix of cost savings and greater efficiency. For example, AI technology could optimize bus routes by analyzing vast amounts of historical and current passenger data. Using this as a platform, AI-based applications may then simulate various solutions and forecast how they would impact timelines and efficiency. Additionally, better route planning goes hand in hand with greater linkages. Nobody enjoys getting to their connection point only to learn that their next bus left a minute ago and that they must wait for another half an hour.
As we can see, AI-powered buses could offer a win-win scenario for companies, commuters, and government officials. However, the AI technology used to power these next-generation buses needs to be accurately trained to make sure it gets the job done correctly. This is done through data annotation, and we will explore this later in the article.
Reducing Greenhouse Emissions
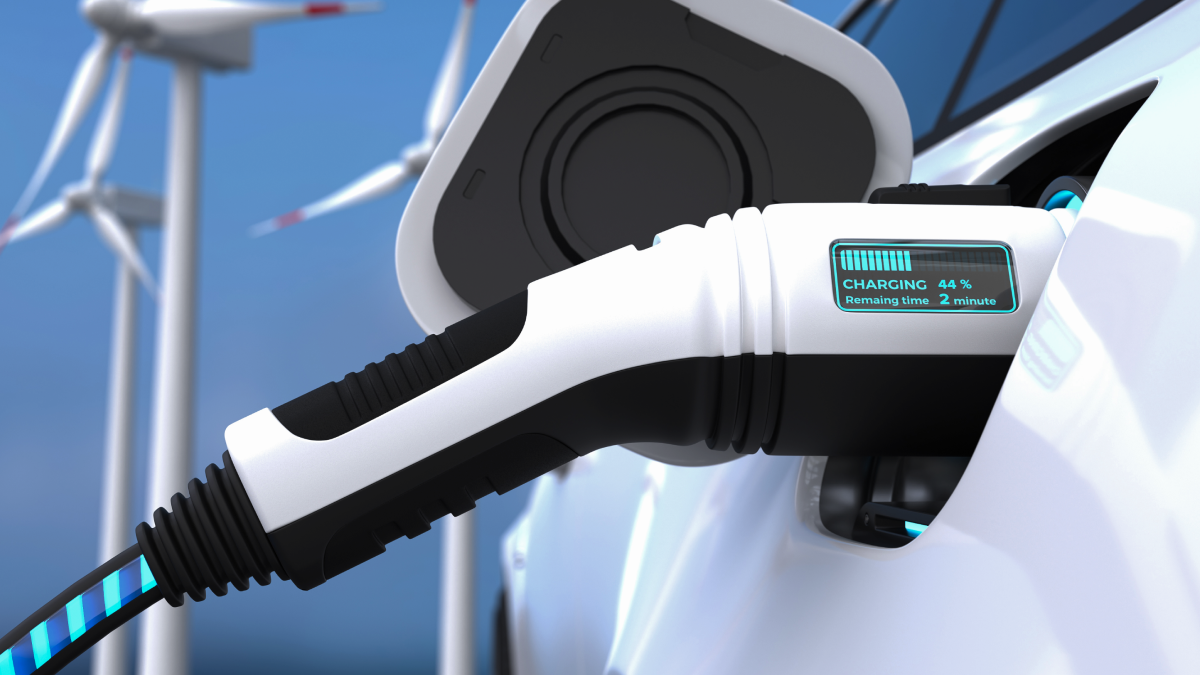
Buses are a substantial source of the transportation industry’s carbon emissions, which are among the top global emitters. Many cities are using artificial intelligence (AI) to assist in cutting carbon emissions in an effort to lessen the environmental impact of bus transit. The efficiency boost offered by AI can be used to reduce carbon emissions. For example, individual bus performance may be tracked using AI-powered tools, which can also notify drivers when maintenance is required. By guaranteeing that buses are operating at their highest level of efficiency, this can aid in lowering fuel consumption and carbon emissions.
In addition to this, the route planning possibility discussed in the previous section can be used to lower emissions since more optimized routes and schedules lead to less fuel consumption. Data from AI buses can also be analyzed to find even more opportunities to improve fuel efficiency. Finally, if a city decides to transition to more electric vehicles, AI-powered solutions can be used to monitor the performance of electric buses and identify areas where improvements can be made.
What Types of Data Annotation are Needed to Make AI Buses a Reality
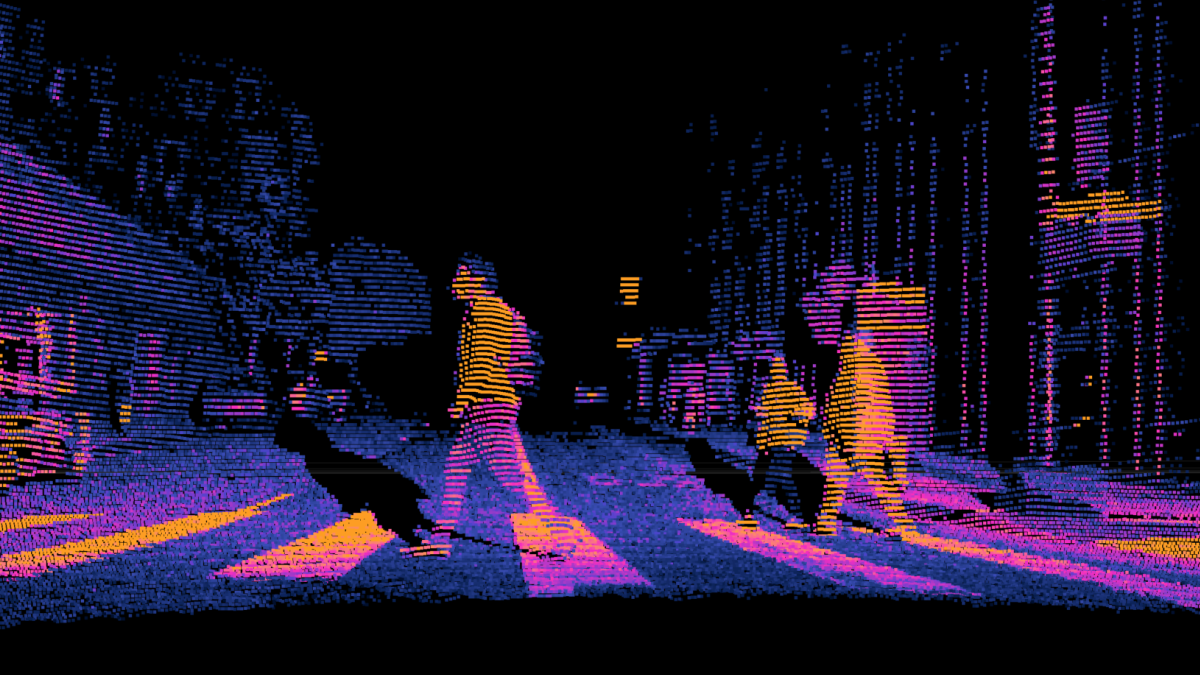
One of the main tools used in the development of autonomous vehicles, such as buses or cars, is LiDAR. This technology creates a 3D Point Cloud, which is a digital representation of how the AI system views the physical environment. This 3D Point Cloud Needs to be annotated with techniques like cuboids, which help the system identify things like vehicles, vulnerable road users, traffic signs, traffic lights, etc. More detailed types of annotation are usually required, like polylines, to enable the system to identify curbs, road pavement, and lane markers.
Additional image and video annotation methods can be helpful in training AI-powered buses like semantic segmentation. It is a computer vision task in which the goal is to categorize each pixel in an image into a class or object. The goal is to produce a dense pixel-wise segmentation map of an image, where each pixel is assigned to a specific class or object. Similarly, instance segmentation can be useful here, which identifies specific entities within a class. For example, if semantic segmentation identifies all the pedestrians in the image, instance segmentation can identify individual pedestrians.
Trust Mindy Support With All of Your Data Annotation Needs
Mindy Support is a global provider of data annotation services and is trusted by Fortune 500 and GAFAM companies. With more than ten years of experience under our belt and offices and representatives in Cyprus, Poland, Romania, The Netherlands, India, OAE, and Ukraine, Mindy Support’s team now stands strong with 2000+ professionals helping companies with their most advanced data annotation challenges.