When It Comes to Training AI, There’s No Cutting Corners
Category: AI insights
Published date: 29.11.2021
Read time: 5 min
When you are driving from one destination to another, there may be a shortcut that will allow you to get to your destination faster. However, if you take shortcuts with training your machine learning model, this could lead to disaster. Within the context of machine learning, a shortcut is when the system relies on a simple characteristic of a dataset to produce a decision, instead of learning the true essence of the dataset. However, this shortcut is caused by an earlier shortcut that was taken by developers when training the machine learning algorithms. Today we will take a closer look at this issue so you can understand some of the dangers of such shortcuts.
What Shortcuts Might Be Taken During the ML Training Process?
Let’s imagine that we are developing an autonomous vehicle and we need the machine learning algorithms to understand all of the lines on the road. Below is one of the images in the training dataset we are using:
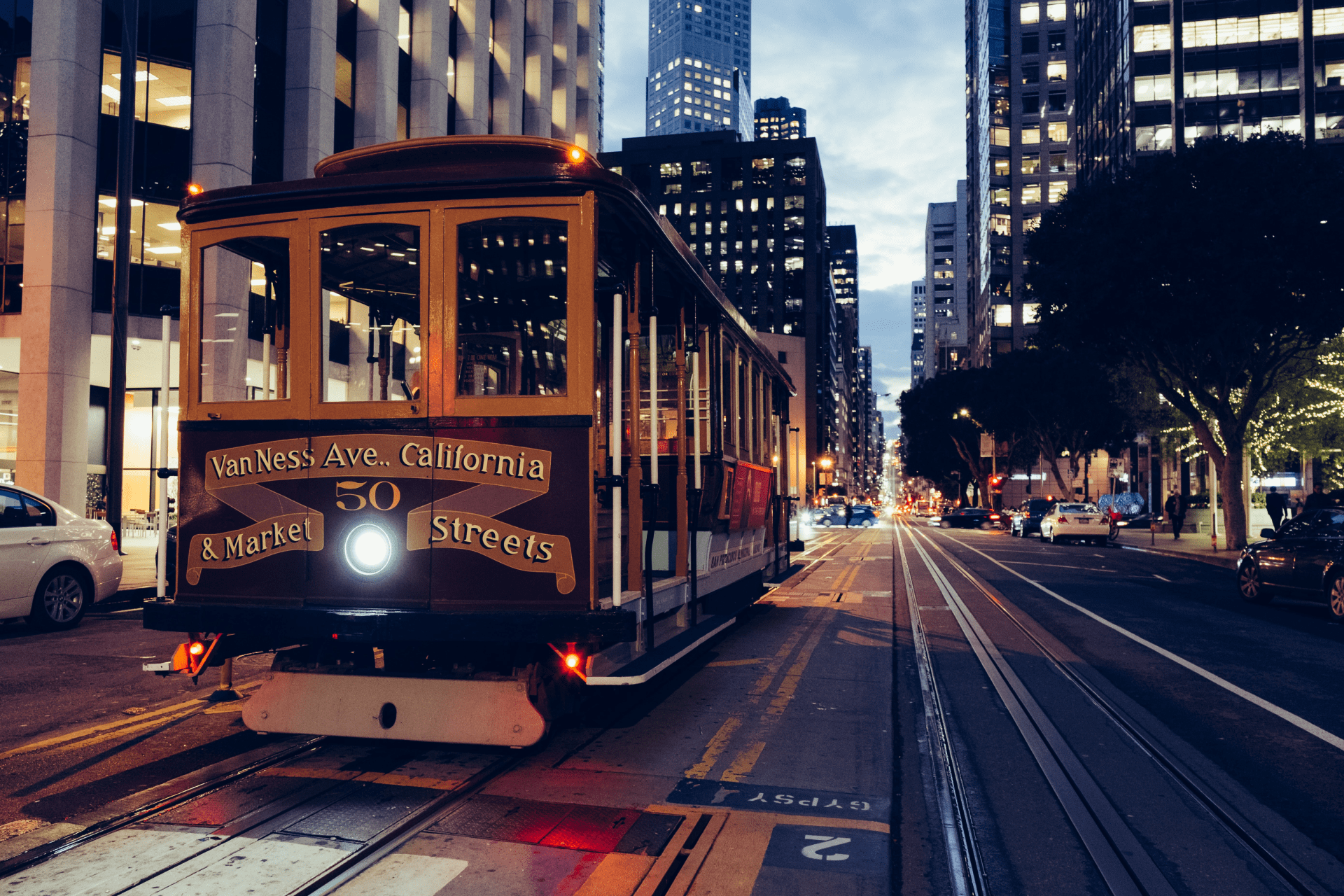
Here we have many different kinds of lines: solid lines, broken lines, and double yellow lines. So, a data annotator would need to label all of the lines in this and other images and these labeled images would need to be used to train the AI model. However, if we look carefully, we see there are also tram tracks. What would the autonomous vehicle do if it encountered these tracks on the road and they were not accounted for during the training process?
In this example, the shortcut was overlooking the tram tracks and leaving it up to the car to infer what it is based on what it knows about the other lines. This is fairly risky because, first of all, the system may correctly identify the tracks, and, secondly, the road rules for crossing the tracks may be different from a solid white line, for example. Therefore, you need to account for this situation to avoid a situation where the car doesn’t know what to do.
Speaking of unknown situations, let’s explore what happens when AI systems encounter them.
How Do AI Systems Handle Unknown Situations?
The way AI systems handle unknown situations will vary on a case-by-case basis. If we stick with our example of a self-driving car, it might use its own uncertainty to estimate the risk of potential collisions or other traffic disruptions at such intersections. It weighs several critical factors, including all nearby visual obstructions, sensor noise and errors, the speed of other cars, and even the attentiveness of other drivers. Based on the measured risk, the system may advise the car to stop, pull into traffic, or nudge forward to gather more data.
If we shift over to healthcare AI, medical data is more prone to uncertainty due to the presence of noise in the data, meaning that the data may corrupt in some way. It is worth noting that the definition of “noisy data” has expanded to include any data that cannot be understood and interpreted correctly by machines. So, it is very important to have clean medical data without any noise to get an accurate diagnosis. Generally, uncertainty may be due to two reasons: data (noise) uncertainty and model uncertainty (also called epistemic uncertainty).
So, how would the system handle this uncertainty? Well, there are several ways:
- Imprecise probability – generalizes probability theory to allow for partial probability specifications, and is applicable when information is scarce, vague, or conflicting.
- Monte Carlo Simulation – used to model the probability of different outcomes in a process that cannot easily be predicted due to the intervention of random variables.
- Bayesian Inference – Bayesian definition of probability is based on our knowledge of events. In the context of machine learning, we can interpret this difference as what the data says versus what we know from the data.
There are many more techniques, and each of them is very complex, which is why it is better to perform your data annotation work in such a way that accounts for as many variables as possible to minimize unknown events.
Mindy Support Can Provide You With All of the Data Annotation Services You Need
If you are looking for a service provider to annotate your data without cutting corners, consider hiring Mindy Support to perform this work for you. We are the largest data annotation company in Eastern Europe with more than 2,000 employees in eight locations all over Ukraine and in other geographies globally. Contact us today to learn more about how we can help you.