What is Data Labeling? The Ultimate Guide
Category: Best practice articles
Published date: 28.09.2022
Read time: 9 min
Data labeling is an important aspect of training machine learning models and making sure they can accurately identify various objects in the physical world. Labeled data plays a crucial role in the development of ML models, since it will determine the overall accuracy of the system itself. In order to help you better label data, we created this data labeling guide to help you better actualize your project.
What is Data Labeling?
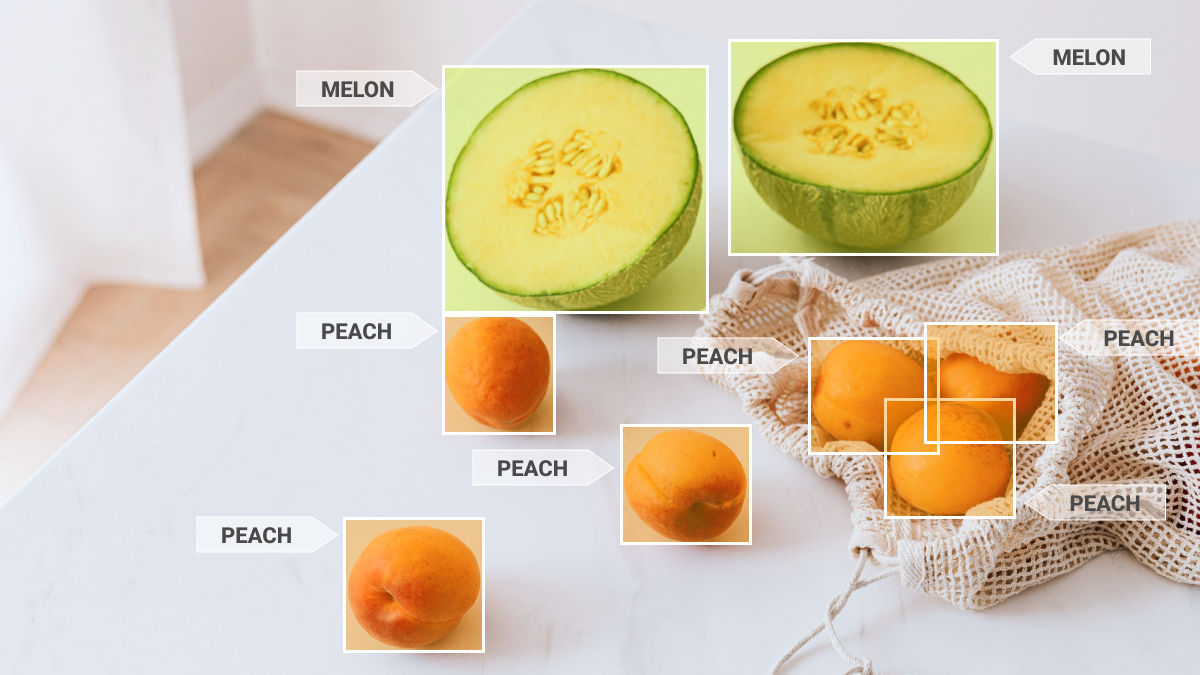
Data labeling, in the context of machine learning, is the act of recognizing unprocessed data (pictures, text files, videos, etc.) and appending one or more relevant and meaningful labels to give context, thus enabling a machine learning model to learn from the data. Labels may denote, for instance, the words spoken in an audio recording, the presence of a car or bird in a picture, or the presence of a tumor in an x-ray. For many use cases, such as speech recognition, natural language processing, and computer vision, data labeling is necessary.
Why Use Data Labeling?
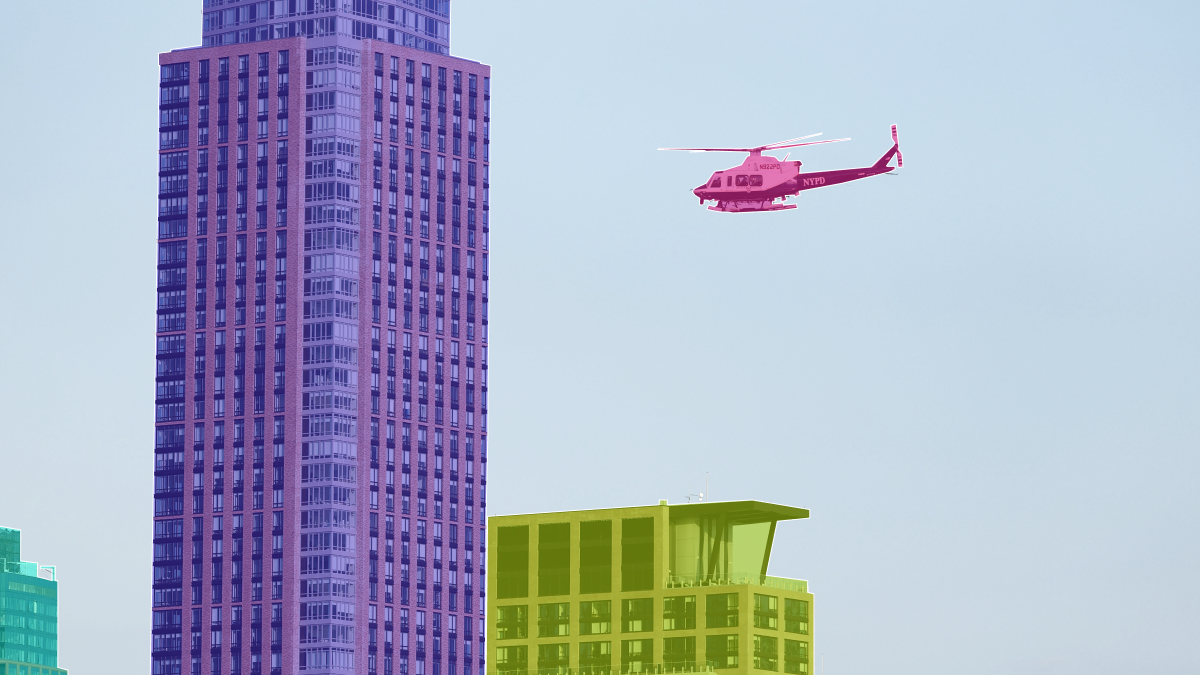
For a machine learning model to perform a given task, it needs to navigate or understand its surrounding environment accurately. This is where the data label aspect comes into play because it is exactly this that tells the model what an object is. Application stakeholders must be aware of a model’s level of confidence in its predictions in order to implement AI models in real-world applications. It is crucial to make sure that employees involved in the labeling process are being evaluated for quality assurance purposes because this may be traced all the way down to the data labelling stage.
How Does Data Labeling Work?
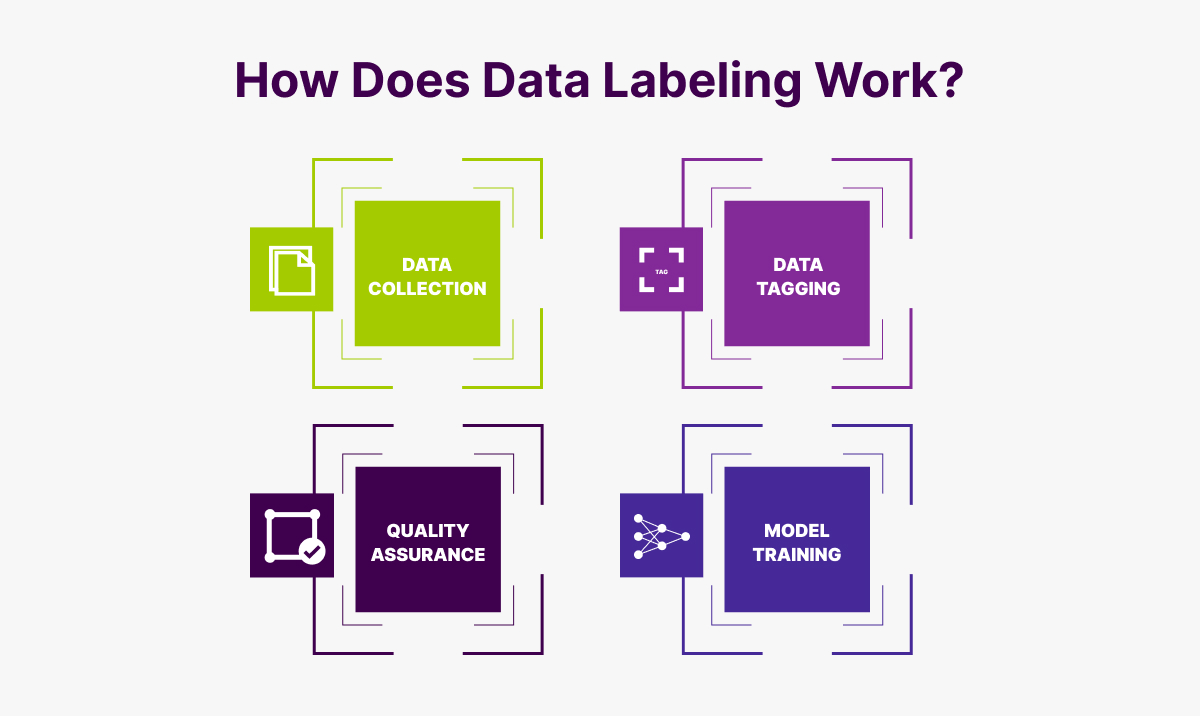
Now that we know what is labeled data, we can move on to how the entire process works. We can boil down the labeling process into four parts:
- Data collection – This is the process of assembling the data you need to label, such as images, videos, audio clips, etc.
- Data tagging – During this process, data annotators would tag all the parts of interest with a corresponding tag to allow the ML algorithms to understand the data.
- Quality assurance – The QA team would go through all the work done by the data annotators to make sure everything was done correctly, and the needed metrics were reached.
- Model training – The labeled data is used to train the model and help it accomplish the needed tasks with greater quality.
Main Types of Data Labeling
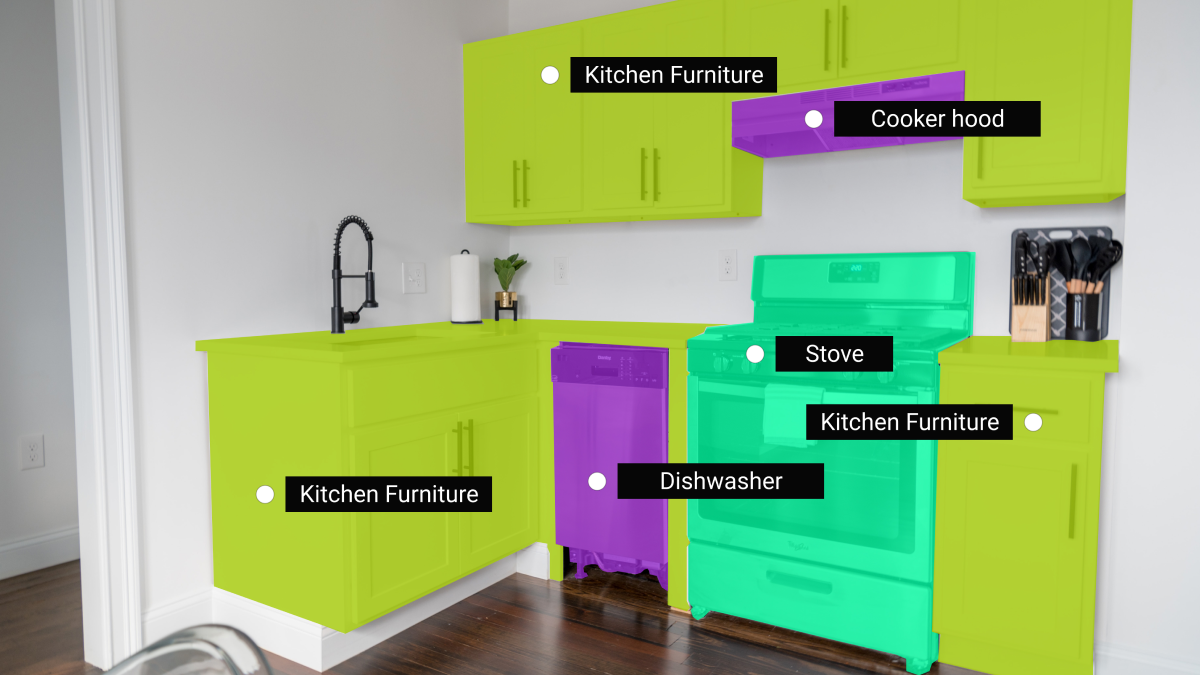
When labeling datasets, there are two main types of data labeling:
- Computer vision – This branch of computer science focuses on giving machines the ability to recognize and comprehend objects and people in pictures and movies. Similar to other forms of artificial intelligence, computer vision aims to execute and mechanize activities that mimic human abilities.
- NLP – With the use of natural language processing (NLP), computers can now comprehend, manipulate, and interpret human language. Large amounts of text and speech data are now being collected by organizations via a variety of communication channels, including emails, text messages, social media news feeds, audio, video, and more.
Benefits of Data Labeling
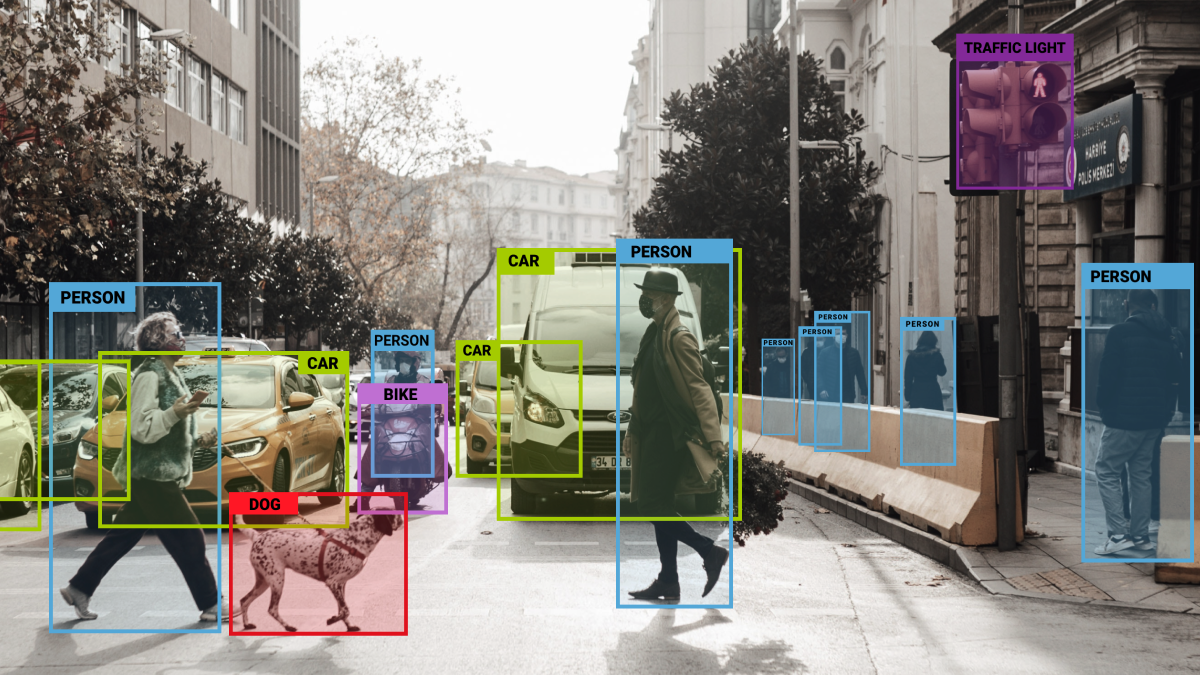
We know what is label data, but what are the benefits of doing so? Here are some of the benefits of labeling your data.
- Precise Predictions – With quality labeled data, your machine learning will have more context about the training datasets, which, in turn, will allow it to obtain greater insights and provide better predictions.
- Improved Data Usability – Thanks to data labeling, machine learning systems are better able to map one input to a particular output, which is more useful for the ML system and the end users.
- Enhanced Model Quality – The higher the quality of the labeled training datasets, the higher the overall quality of the ML system will be.
Challenges of Data Labeling
While data labeling is certainly an important process, there are also a lot of pitfalls to watch out for:
- Domain expertise – It is very important that all the data annotators have extensive experience in not only data labeling but also the industry for which the project is developed. This will help you achieve the needed quality levels.
- Resource constraint – It can be difficult to guarantee that annotators have subject knowledge in specialized industries like healthcare, finance, or scientific research. Inaccurate annotations caused by a lack of domain expertise may affect how well the model performs in practical situations.
- Label inconsistency – One typical problem is keeping labels consistent, especially in collaborative or crowdsourced labeling projects. The dataset may contain noise due to inconsistent labeling, which would impair the model’s ability to correctly generalize.
- Data quality – Model results are directly influenced by the quality of the labeled data. Model reliability depends on ensuring that labels appropriately depict real-world situations and resolving problems like mislabeling and outliers.
- Data security – Preventing privacy violations during the labeling process requires safeguarding sensitive information. Data security requires the use of strong safeguards, such as encryption, access controls, and compliance with data protection laws.
What are Some Best Practices for Data Labeling?
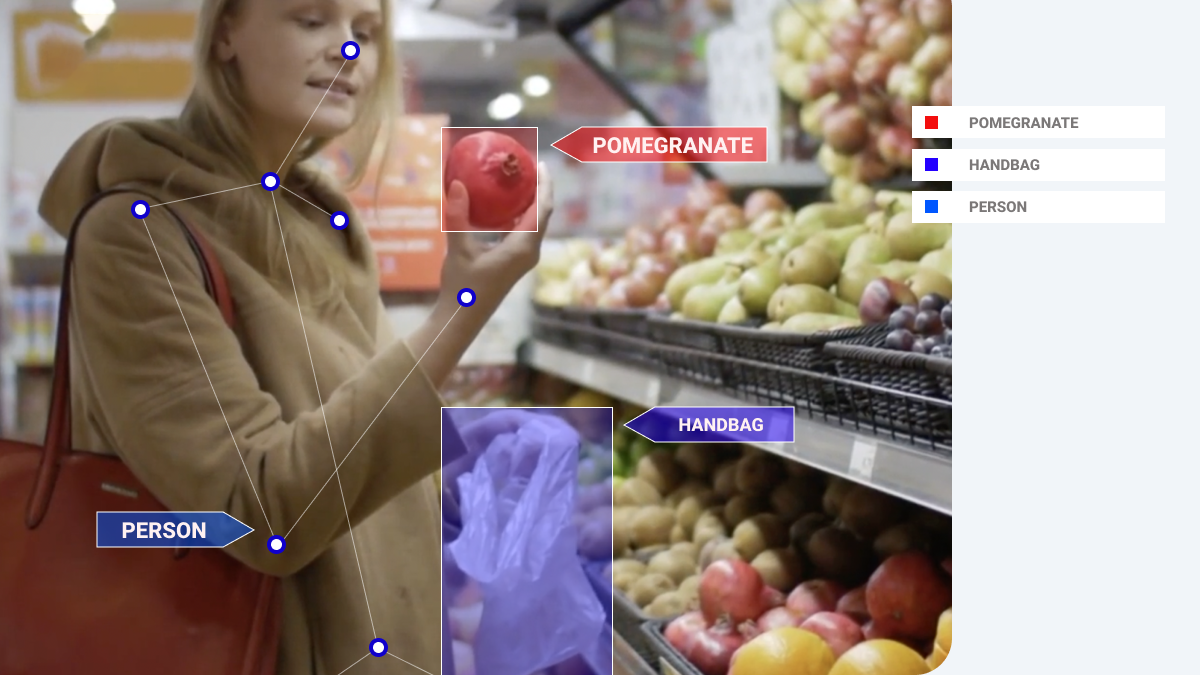
Creating reliable machine learning models requires great data labelling examples. Your actions during this stage greatly impact the effectiveness and caliber of the model. Selecting an annotation platform is crucial to success, especially if it has an easy-to-use interface. These platforms improve data labeling accuracy, productivity, and user experience.
- Intuitive interfaces for labelers – For data labeling to be precise and efficient, labelers must have interfaces that are intuitive and easy to use. These interfaces expedite the process, lessen the possibility of labeling errors, and enhance users’ data annotation experience.
- Collect diverse data – You need to make sure to have a wide range of data samples in your training datasets to make sure the ML system can detect the needed objects or correctly understand various strings of text.
- Collect specific/representative data – An ML model will need to perform a fixed number of tasks, and you need to provide it with real-world, labeled data that gives it the information it needs to understand what that task is and how to accomplish it.
- Label Auditing – It is essential to regularly validate labeled datasets in order to find and fix problems. It entails going over the labeled data to look for biases, inconsistencies, or errors. Auditing ensures the labeled dataset is trustworthy and fits the machine learning project’s goals.
- Set up an annotation guideline – It is important to have a conversation with the data annotation provider to make sure that they understand how the data should be labeled. Having a guide for the teams in place will serve as a good reference point if there are any questions.
- Establish a QA process – As we mentioned earlier, the higher the accuracy of the labeled data, the higher the accuracy of the end product will be. Therefore, it is in everybody’s interest to make sure that all the data labeling tasks are done correctly the first time.
Key Takeaways
The old adage of “garbage in, garbage out” certainly applies to machine learning. Since the input data directly affects how effective the final model is, data labeling is an essential part of training machine learning algorithms. Increasing the amount and caliber of training data can, in fact, be the most effective approach to enhancing an algorithm. The tagging duty is also here to stay due to machine learning’s increasing popularity.