Facebook’s Ego4D Highlights the Importance of Data Collection
Category: AI Insights
Published date: 01.11.2021
Read time: 6 min
This month, Facebook AI unveiled one of its most advanced and long-term projects, Ego4D. What’s great about Ego4D is that it is a massive egocentric dataset consisting of 3,025 hours of video collected by 855 unique participants from 74 worldwide locations in 9 different countries. This will be a tremendous help to researchers who are working on AI technology that would allow the AI to understand and interact with the world like we do, from a first-person perspective. Let’s take a closer look at how companies developing AI solutions will be able to take advantage of Ego4D and then move on to the importance of having the right training data for your AI project.
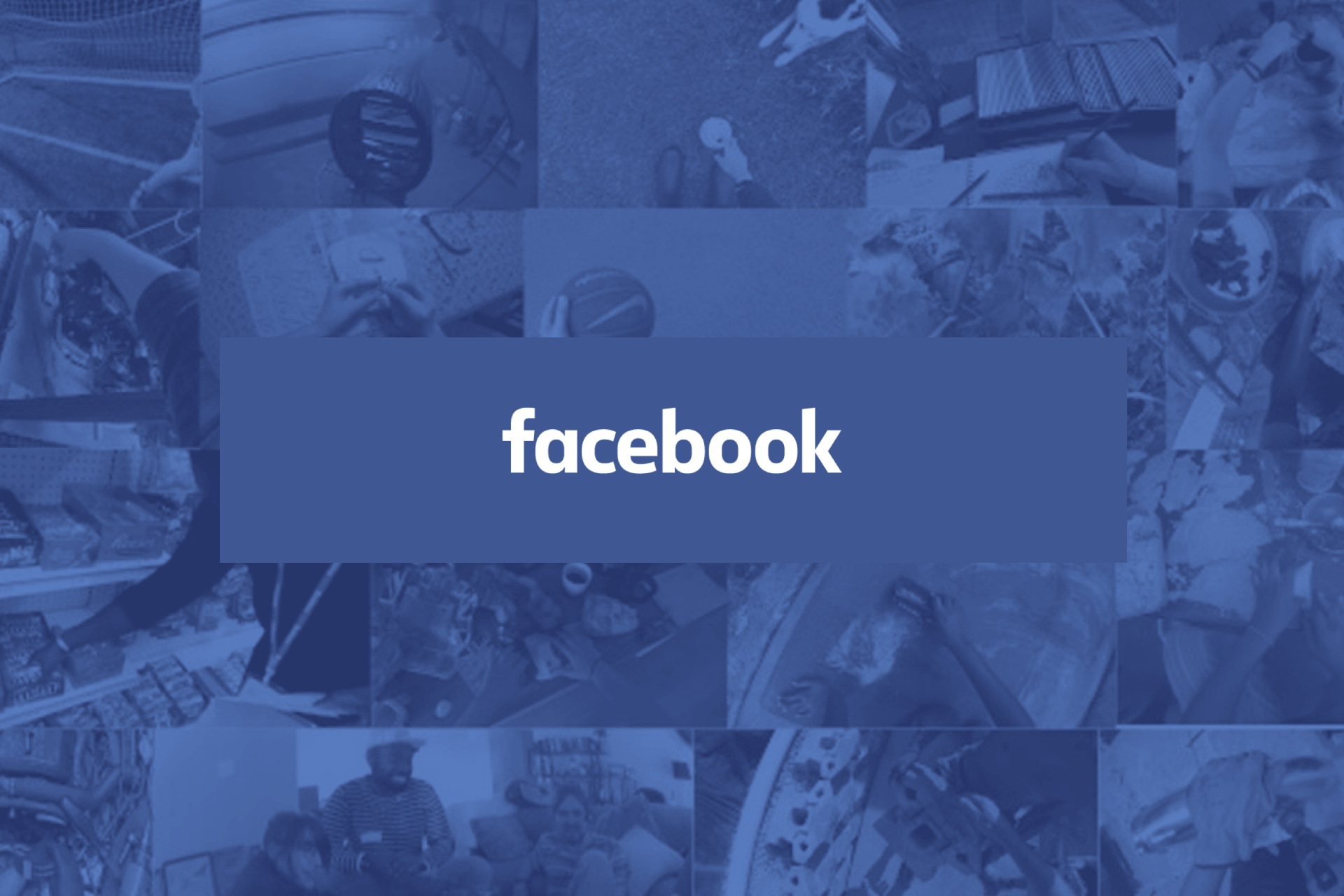
What Problem is Ego4D Intended to Solve?
When we think about the usual way researchers train AI models, they typically use photos and videos captured in third-person, meaning that, for example, one person takes a picture of somebody else performing some action. Now imagine being able to take a picture from the first-person perspective. You are in the middle of the action and can see what the person performing the action is seeing. These are the types of photos and videos next-gen AI systems will need to learn from.
Facebook needs such a dataset because they are developing a number of projects that can benefit from AI models trained using video footage shot from a human perspective. This includes smart glasses like Ray-Ban Stories, released last month on Facebook, and virtual reality, which Facebook has invested heavily since acquiring Oculus for $2 billion in 2014. However, if we look at the broader implications, AI that understands the world from this point of view could unlock a new era of immersive experiences, as devices like augmented reality glasses and virtual reality headsets become as useful in everyday life as smartphones.
Why Is It So Important to Have the Right Training Data?
One of the things we have learned from Facebook AI is that training data is very important. From the perspective of the research direction of artificial intelligence technology, whether in the field of traditional machine learning or deep learning, supervised learning based on training data is still a major model training method. Especially in the field of deep learning, more labeled data is needed to improve the effectiveness of the model. Human data annotators would be responsible for preparing the training dataset with labels, 2D/3D boxes, semantic segmentation, and many other annotation types.
By identifying similar patterns the algorithm can then self-identify unlabeled data in the future. It is here where the machine is learning and it is precisely why the data that forms these patterns must be high quality or “clean.” Feeding the machine with poor or “dirty” data creates problems that will compound down the line as the algorithm is put into use and interprets the wrong patterns in the future.
What if the Needed Training Data is Not Available?
There may be situations where a company needs a very specific dataset to add new functionalities to their AI system, but it is simply not available in open-source repositories or for purchase from another company. Now, in the case of Facebook, they saw that what they were looking for is not available so they simply made their own training dataset. Now, you may think that only a billion-dollar company, like Facebook, might be able to afford such a luxury, but the good news is that data collection outsourcing has democratized this process. Now, companies of all sizes can collect the data they need.
For example, we at Mindy Support, recently helped one of our clients collect training data for an AI chatbot that could converse with customers in the insurance, banking, logistics, e-commerce, and other industries. We assembled 100 agents that generated and annotated more than 20,000 dialogues over the course of 4,000 hours. You can read more about this in our case study.
We helped another client who was working on an in-car system that could detect that a driver is falling asleep and send out an alert to wake them up. This was a large project that involved collecting data from video recordings of eye reactions to external factors in a state of varying degrees of fatigue and under the influence of various factors. As you may imagine, finding a training dataset for such a project is very difficult so we helped them create one as well. You can read more about this use case here.
Trust Mindy Support to Help You Collect the Needed Data
If you are unable to find exactly the right training dataset for your project, consider hiring Mindy Support to help you collect the needed data. We are the largest data annotation company in Eastern Europe with more than 2,000 employees in seven locations all over Ukraine and in other geographies globally. Contact us today to learn more about how we can help you.