Self-Development Supercomputers vs External Partnerships: Which is Better for ADAS?
Category: AI insights
Published date: 03.10.2023
Read time: 5 min
The amount of communication between various car components has increased recently thanks to the in-vehicle network. This is the consequence of a sharp rise in functionality, which provides passengers with superior comfort, entertainment, and driving performance compared to earlier models. The underlying network of wires, computers, actuators, and sensors will act as the vehicle’s central nervous system as operating the vehicle becomes a task that can only be accomplished by a supercomputer. However, many argue that the current setup needs to be reconsidered because it will not be suitable for autonomous vehicles.
Having said this, is it better to create your own custom supercomputer or partner with a cloud provider to process all of this data to implement ADAS features? In this article, we will explore this question as well as the data annotation required to train ADAS technology.
Tesla’s Proprietary Supercomputer
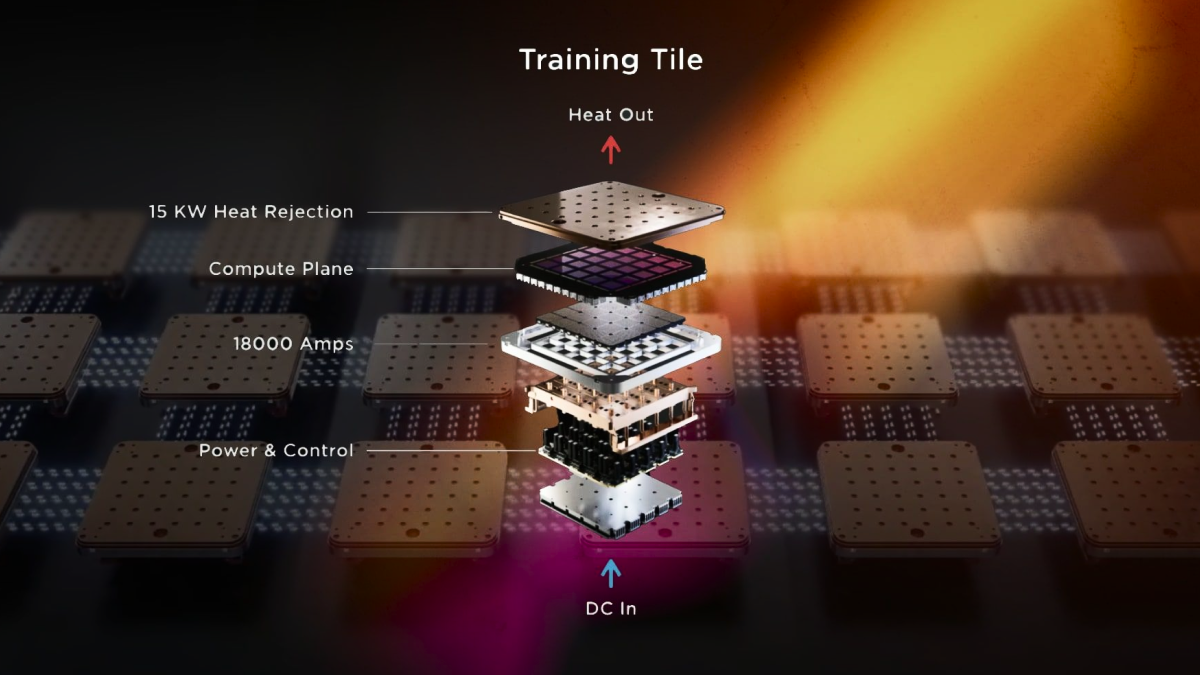
As one of the leaders in the autonomous vehicle industry, Tesla gets a lot of media attention, and it is pretty easy to see why. They use cutting-edge technology in every aspect of their vehicles, including the battery, software, and hardware, giving them a significant edge over their competitors. Therefore, exploring their self-developed supercomputer, called Dojo, is a great example of advancements a company can make in this area all by themselves.
It is a supercomputer that Tesla built specifically for use in processing and recognizing videos using computer vision. In order to develop and expand the capabilities of Tesla’s autonomous vehicles, it is used to train the machine learning algorithms used in such systems. The supercomputer is essential for training the sophisticated AI models used in self-driving cars because it can handle large amounts of data and carry out difficult computations at an astounding rate.
Since Tesla’s Dojo is specifically built for processing video data, unlike conventional supercomputers, it is ideally suited for training neural networks that evaluate visual data. Due to its focus, the supercomputer is able to manage the enormous volumes of video data produced by Tesla’s fleet of autonomous cars on a regular basis.
Partnering With a Cloud Provider for Processing Power
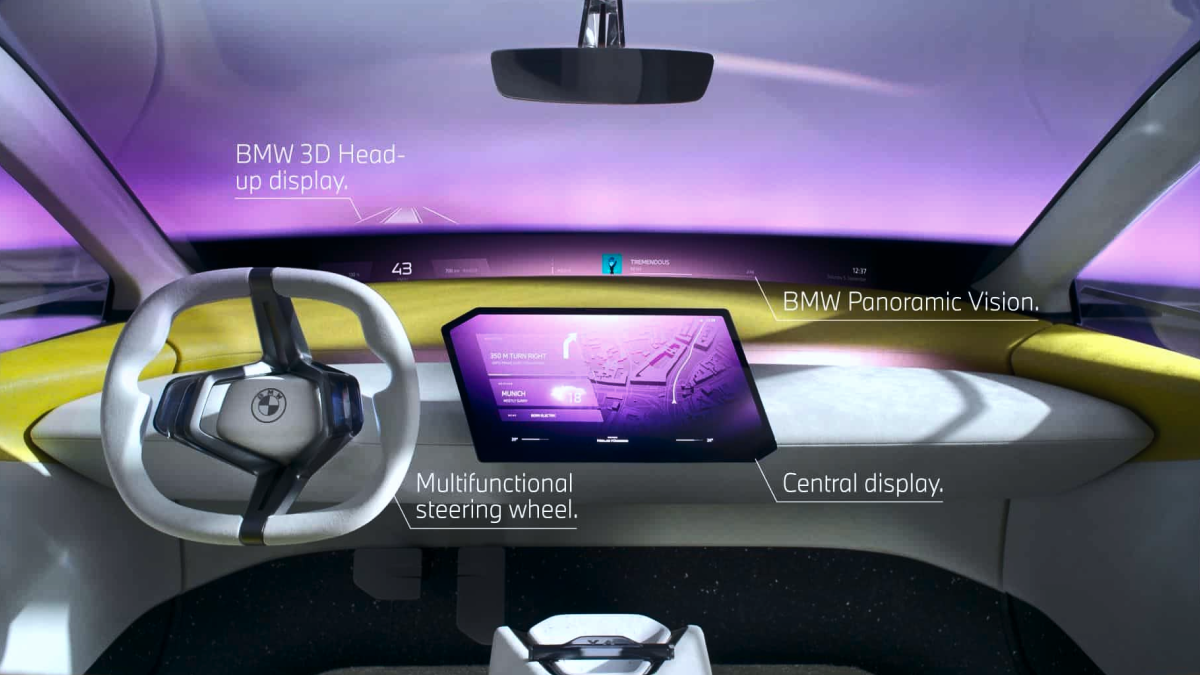
BMW decided to take a different approach and partner with AWS to create an autonomous driving platform to develop its next-generation advanced driver assistance system (ADAS) to introduce innovative features in its upcoming vehicles, known as the “Neue Klasse,” slated for launch in 2025. This innovative cloud-based system intends to speed up the production of highly automated BMW vehicles with the use of AWS computation, generative artificial intelligence (generative AI), Internet of Things (IoT), machine learning, and storage capabilities. BMW’s current Cloud Data Hub on AWS will be expanded through this partnership, enabling a seamless connection of their technologies.
BMW will be able to develop an advanced, scalable, and automated driving platform based on a single reference design, thanks to this. This platform will shorten the development cycle and broaden its use to additional BMW models. For example, the platform offers essential tools for effectively organizing, processing, and storing enormous amounts of real-time driving data in Amazon Simple Storage Service.
What Types of Data Annotation are Required to Create ADAS Technology?
ADAS technology relies on computer vision to identify objects in the physical world and navigate around them. This means data annotation techniques like polyline annotation. In both photos and videos, linear structures are defined using this method. It traces the outline of constructions like roads, rail tracks, and pipelines using short lines joined at vertices. Using annotation platforms and labeling tools, annotators add these lines to the photos. These lines must be identified in each frame of video training data.
Semantic segmentation will also be needed, which is a deep learning method that gives each pixel in a picture a label or classification. It is used to identify groups of pixels that represent various categories. An autonomous vehicle, for instance, needs to recognize other cars, pedestrians, traffic signs, pavement, and other elements of the road.
Trust Mindy Support With All of Your Data Annotation Needs
Mindy Support is a global provider of data annotation services and is trusted by Fortune 500 and GAFAM companies. With more than ten years of experience under our belt and offices and representatives in Cyprus, Poland, Romania, The Netherlands, India, OAE, and Ukraine, Mindy Support’s team now stands strong with 2000+ professionals helping companies with their most advanced data annotation challenges.