Revolutionizing In-Vehicle Experiences with Large Language Models
Automotive Large Language Models (ALLMs) are advanced AI systems specifically trained by data labeling and other techniques to understand and generate human language in the context of automotive data solutions. These models can assist with various tasks such as enhancing in-car voice assistants, improving customer service through chatbots, and aiding in the design and engineering processes by providing insights and automating documentation.
This technology is revolutionizing the way cars are driven by enabling more natural and intuitive voice interactions, allowing drivers to control vehicle functions, navigate, and access information seamlessly through conversational AI. They enhance personalization by learning user preferences, providing tailored recommendations, and ensuring safer, more enjoyable journeys with hands-free operation and advanced infotainment systems.
Needless to say, there are some big impacts that large automotive language models are having on the automotive industry, such as streamlining operations from design and manufacturing to customer service and after-sales support. They are fostering innovation in autonomous driving, enhancing vehicle safety through real-time data analysis, and transforming user experiences with advanced in-car assistants and personalized services, driving the industry toward a more connected and intelligent future.
Significance of ALLMs in Automotive Innovation
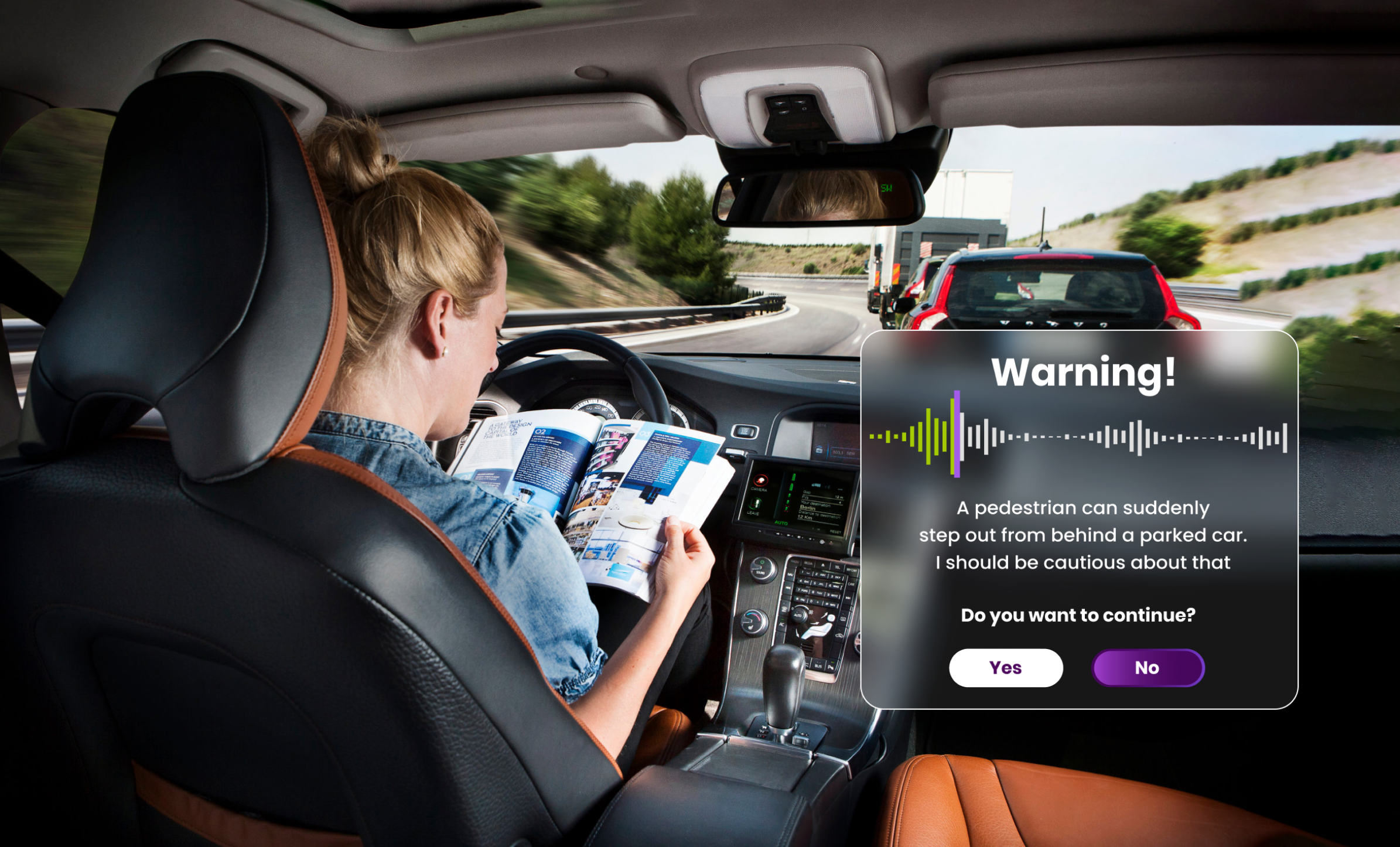
Driver Assistance Systems are improved by ALLMs by providing real-time, context-aware responses and predictive insights, which enhance navigation, hazard detection, and driver alerts. These models enable more sophisticated, natural language interactions, allowing drivers to access information and control effortlessly, thus increasing safety and convenience on the road.Large language models in cars are also enhancing car connectivity and interactions by enabling seamless communication between the vehicle, driver, and external devices through natural language processing. They facilitate intuitive voice commands and personalized user experiences, allowing drivers to control smart home devices, access cloud-based services, and stay connected while on the go, thus transforming cars into integrated hubs of digital interaction.
Automotive Large Language Models are revolutionizing car interactions and the way cars are driven by enabling more intuitive and responsive driver-vehicle interactions through advanced voice commands and real-time data processing. They support autonomous driving technologies by interpreting and responding to complex scenarios, enhancing navigation, and providing predictive maintenance alerts, thereby making driving safer, more efficient, and increasingly autonomous.
Benefits of ALLMs in Automotive Applications
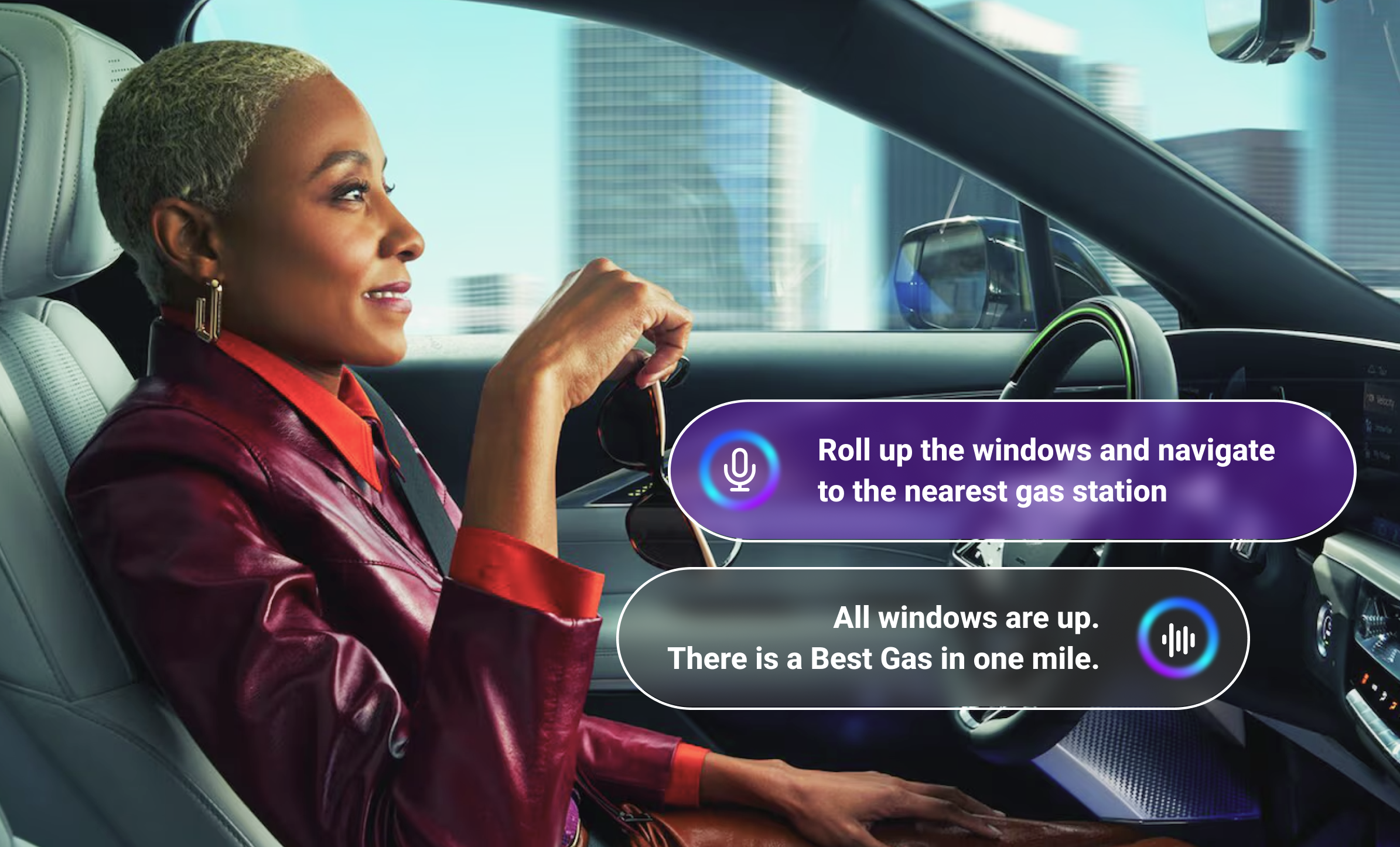
Artificial intelligence in vehicles is making driving safer by providing real-time hazard detection, adaptive cruise control, and lane-keeping support through advanced machine-learning algorithms. These systems enhance situational awareness, reduce human error, and offer timely alerts and interventions, significantly lowering the risk of accidents and improving overall road safety.Next-gen car entertainment and connectivity systems are transforming the in-vehicle experience by integrating high-speed internet, streaming services, and seamless device pairing. Such advancements enable passengers to enjoy personalized multimedia content, real-time navigation updates, and smart home controls, creating a connected and immersive environment on the go.
Enhanced user experience and satisfaction in modern vehicles are achieved through intuitive interfaces, personalized settings, and advanced voice recognition technologies. These innovations allow for effortless control of vehicle functions, tailored infotainment options, and seamless connectivity, ensuring a comfortable and engaging driving experience that meets individual preferences and needs.
Multimodal Data Annotation for ALLMs
Multimodal data is important in the automotive context as it combines information from various sources such as cameras, sensors, and GPS to create a comprehensive understanding of the vehicle’s environment. This integration enhances the accuracy of driver assistance systems, supports autonomous driving capabilities, and improves safety by providing a more detailed and reliable analysis of road conditions and potential hazards.
Annotation techniques used by automotive outsourcing for automotive large language models involve several approaches to label and annotate data effectively:
- Text Annotation – Identifying and tagging specific entities, intents, or sentiments within textual data related to automotive contexts, such as vehicle features, user queries, or service requests.
- Speech Annotation – Transcribing and annotating spoken language to train models for speech recognition and generation, crucial for developing robust in-car voice assistants.
- Image and Video Annotation – Labeling visual data from cameras and sensors to recognize objects, traffic signs, pedestrians, and other relevant elements for autonomous driving and driver assistance systems.
- Sensor Data Annotation – Annotating data from various sensors (e.g., lidar, radar) to classify objects, detect obstacles, and predict movements, vital for enhancing vehicle safety and navigation.
- Behavioral Annotation – Analyzing and labeling driving behaviors and patterns to improve predictive models for adaptive cruise control, lane-keeping assistance, and other driver assistance features.
Multimodal data annotation presents challenges, such as aligning diverse data types (text, image, video, sensor data) for comprehensive analysis and ensuring consistency across annotations. Solutions involve developing integrated annotation tools that support multiple data formats, employing automated annotation techniques where feasible, and establishing rigorous quality control measures to maintain accuracy and reliability in annotated datasets. These efforts are crucial for training robust automotive AI models capable of understanding complex, real-world environments and interactions.
Risks and Challenges of Implementing ALLMs in Vehicles
Implementing large language models (LLMs) in automotive systems raises significant security and privacy concerns. These models could be vulnerable to cyberattacks, potentially allowing malicious actors to manipulate vehicle behavior or access sensitive data. Additionally, the collection and processing of vast amounts of user data by LLMs pose risks to user privacy, necessitating robust encryption and stringent data handling protocols to prevent unauthorized access and misuse.
Integrating AI for automotive innovation, like ALLMs, into vehicle systems presents several challenges, including the need for substantial computational power and real-time processing capabilities within the vehicle’s hardware. Ensuring seamless and reliable communication between the LLMs and the vehicle’s existing software and hardware infrastructure is also complex, requiring sophisticated integration techniques and rigorous testing to maintain safety and functionality.
The deployment of large language models (LLMs) in automotive systems brings forth ethical and regulatory issues, such as ensuring fairness and avoiding biases in decision-making processes that could impact driver and passenger safety. Additionally, there is a need for comprehensive regulatory frameworks to govern the use of LLMs, addressing concerns related to accountability, transparency, and the protection of consumer rights and data privacy.
Tools and Technologies for ALLM Deployment in Vehicles
Automotive large language model platforms are designed for enhanced car connectivity and the functionality and user experience of in-vehicle systems through advanced natural language processing capabilities. These platforms enable a range of applications, from sophisticated voice assistants that can understand and respond to complex commands to predictive maintenance systems and advanced driver assistance systems (ADAS) that leverage contextual understanding to improve safety and efficiency.
LLMS for safer drivingintegration with ADAS enhances their ability to interpret and respond to natural language commands, enabling more intuitive interaction between the driver and the vehicle. This integration improves the functionality of ADAS features such as navigation, collision avoidance, and adaptive cruise control by providing real-time, context-aware insights and instructions based on the driver’s spoken input. Enhancing automotive large language model (ALLM) capabilities through advanced data analysis technologies allows for more accurate and personalized responses by leveraging vast amounts of driving and user behavior data. Machine learning and big data analytics enable ALLMs to continuously learn and adapt, improving their predictive accuracy and the overall driving experience by identifying patterns and trends in real time.
Case Studies of ALLM Integration in Vehicles
Successful implementations of automotive large language models (ALLMs) in cars have led to significant advancements in driver assistance and infotainment systems. For example, vehicles equipped with ALLMs now feature highly responsive voice-activated controls for navigation, climate settings, and entertainment, enhancing convenience and safety by allowing drivers to keep their focus on the road. Real-world applications of ALLMs include advanced voice assistants for hands-free control, real-time language translation for international travelers, and intelligent navigation systems that provide context-aware route suggestions. The benefits of ALLMs in these applications encompass increased driver safety through reduced distraction, enhanced user convenience, and a more personalized driving experience tailored to individual preferences and needs.
Lessons learned from the implementation of automotive large language models (ALLMs) highlight the importance of robust data privacy measures and the need for continuous updates to address emerging security threats. Future directions involve refining the integration of ALLMs with other vehicle systems, enhancing their contextual understanding, and expanding their capabilities to support fully autonomous driving, thereby offering an even safer and more intuitive user experience.
Future Directions and Opportunities in Automotive AI
Emerging trends in AI for automotive innovation include the development of more advanced autonomous driving technologies, leveraging deep learning and sensor fusion for improved perception and decision-making. Additionally, the integration of AI-driven predictive maintenance and smart connectivity features enhances vehicle performance and user convenience, paving the way for a future of highly intelligent, self-sufficient vehicles. Potential applications of automotive large language models (ALLMs) beyond current capabilities could include more sophisticated interaction with passengers, such as personalized entertainment recommendations based on mood analysis or proactive assistance in emergency situations through advanced dialogue understanding. Additionally, future ALLMs might facilitate seamless integration with smart city infrastructures, enabling vehicles to communicate with traffic systems and infrastructure for optimized navigation and energy efficiency.
The integration of advanced large language models in vehicles holds profound implications for the future of in-vehicle experiences. It promises to transform how drivers and passengers interact with vehicles, offering personalized, intuitive interfaces that enhance convenience, safety, and overall satisfaction. These developments are poised to redefine the driving experience, making it more interconnected with digital services and adaptable to individual preferences and needs.
Conclusion
Automotive large language models (ALLMs) offer benefits such as enhanced user convenience through intuitive voice commands, improved safety features like proactive assistance, and personalized driving experiences. However, they also bring risks related to data privacy vulnerabilities and the potential for system malfunctions. Tools like robust encryption protocols and continuous monitoring are essential to mitigate these risks and ensure the secure and effective deployment of ALLMs in vehicles.
The integration of automotive large language models (ALLMs) signals a significant evolution in the automotive industry, shifting towards more intelligent and connected vehicles. This evolution includes advancements in autonomous driving capabilities, enhanced user interfaces, and the potential for new business models centered around data-driven services and personalized experiences. Manufacturers and stakeholders must adapt to these changes by investing in AI development, cybersecurity measures, and regulatory compliance to stay competitive and meet evolving consumer expectations.
Looking ahead to the future of AI-driven vehicles, we anticipate continued advancements in autonomous capabilities, with vehicles becoming more integrated into smart city infrastructures and offering increasingly personalized experiences. AI-driven vehicles are poised to redefine transportation by prioritizing safety, efficiency, and sustainability while transforming the way people interact with and perceive automotive technology.