New Supercomputer Allows AI to Achieve Human-Level Reading Comprehension
Category: AI Insights
Published date: 09.02.2022
Read time: 6 min
DeepMind, the London-based A.I. research company that is owned by Google-parent Alphabet, has created an artificial intelligence algorithm that can perform a wide range of language tasks—from reading comprehension to answering questions on a broad range of subjects—better than any existing similar software. In a few areas, such as a high school reading comprehension test, the software approaches human-level performance. But in others, including common sense reasoning and mathematical reasoning, the system fell well short of human abilities.
Let’s take a closer look at DeepMind to understand its capabilities and the data annotation that was required to create it.
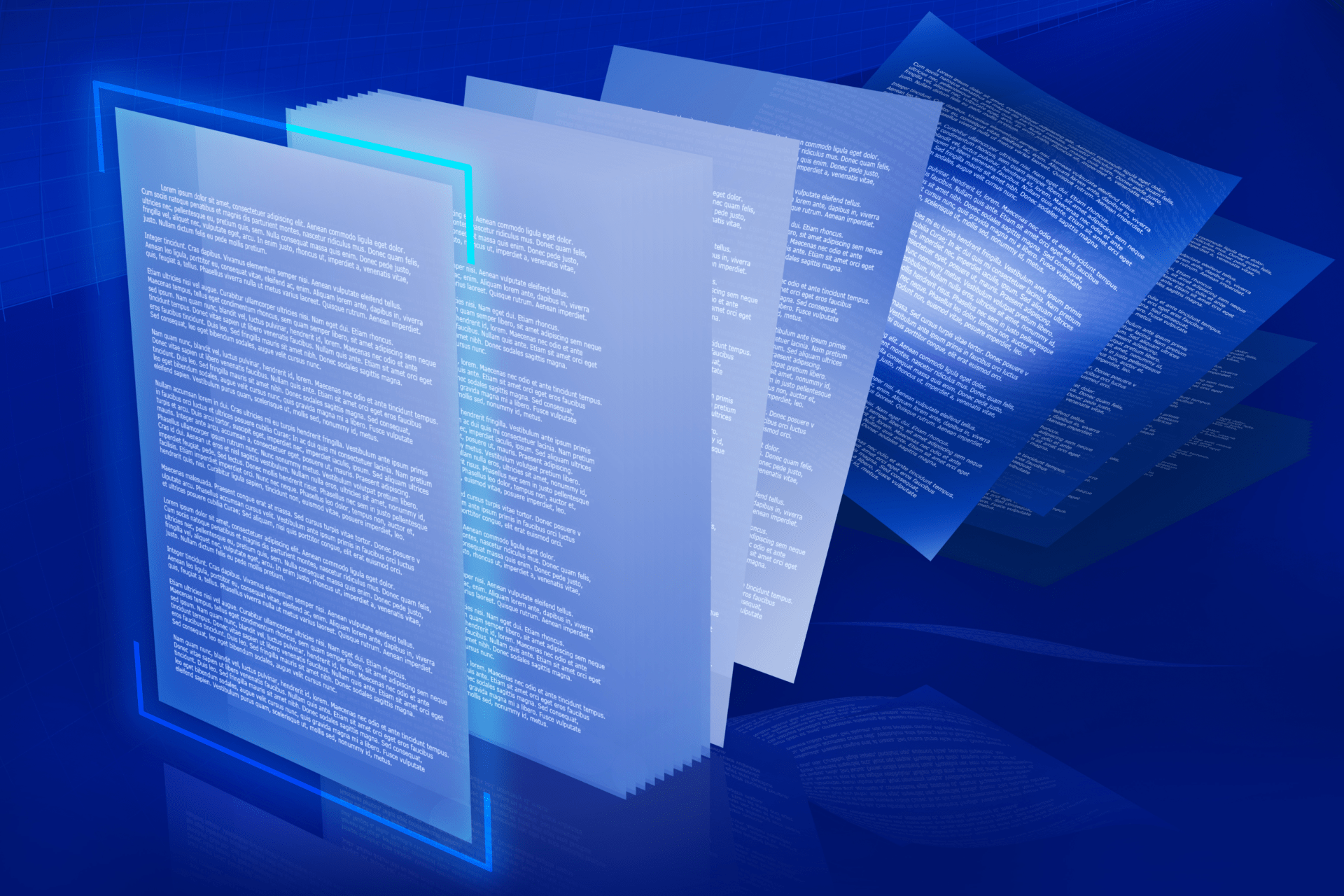
A little Background on Deep Mind
Deep Mind has amassed an impressive list of accomplishments. DeepMind Lab is best known for the creation of the AI AlphaGo, which for the first time managed to defeat the human champion of the game of Go. This is considered an important breakthrough in the study of artificial intelligence because the game of Go is varied enough that victory in it could not be calculated by brute force.
Another little-known but very impressive achievement is that it solved the problem of protein folding that biologists have struggled with for about 50 years. Using their latest AI program, AlphaFold, researchers have shown they can predict how proteins fold into three-dimensional shapes. This is an extremely complex process that is fundamental to understanding the biological mechanisms of life.
Now, the latest achievement is the new supercomputer, called Gopher and we will explore this new technology in the next section.
What is Gopher and What Does It Mean for Businesses?
Gopher is a 280-billion-parameter AI natural language processing (NLP) model. Based on the Transformer architecture and trained on a 10.5TB corpus called MassiveText, Gopher outperformed the current state-of-the-art on 100 of 124 evaluation tasks. It is significantly more accurate than these existing ultra-large language models on many tasks, particularly answering questions about specialized subjects like science and the humanities, and equal or nearly equal to them in others, such as logical reasoning and mathematics, according to the data DeepMind published.
So, what are the practical business applications of Gopher? Well, this new technology will lead to more fluent chatbots and digital assistants, more accurate translation software, better search engines, and programs that can summarize complex documents. Let’s keep in mind that even if a chatbot is already using modern AI technology, like GPT-3 which has 175 billion parameters, Gopher is much larger and brings researchers one step closer to artificial general intelligence. This is a term used to describe A.I. whose intelligence is as adaptable as a human’s—able to compose a symphony and win a quiz show and streamline a factory’s operations—and at least as capable, if not more so.
How Was Gopher Trained?
One of the first challenges in training such large models is finding the right training data. Several such datasets have been open-sourced, such as the Pile and C4, and contain documents scraped from websites such as Wikipedia. The DeepMind team was concerned that simply crawling the web indiscriminately might taint their training dataset with test datasets for their benchmark evaluations, as those are available on the web. To prevent this, DeepMind developed a data-preparation pipeline and a custom training dataset called MassiveText.
Mindy Support has experience helping our clients collect the needed training data that best suits their needs. Recently we assembled a team of 100 data annotators who created more than 20,000 dialogues on 120 different inquiry topics across 5 industries. You can read more about this in our case study.
In addition to this significant volume of data, annotations are required to train the machine learning algorithms. This includes things like semantic annotation which attaches various tags to text that reference concepts and entities, such as people, places, or topics. Named entity recognition also involves assigning labels to words or phrases within a text from predefined categories such as “actor” or “city”. These are just some of the many types of data annotation and many more will be needed to create advanced AI models.
Trust Mindy Support With All of Your Data Annotation Needs
Annotating large volumes of data in-house can be very tedious, time-consuming, and expensive. This is why you should consider outsourcing such work to Mindy Support. We are one of the leading European vendors for data annotation and business process outsourcing, trusted by several Fortune 500 and GAFAM companies, as well as innovative startups. With 9 years of experience under our belt and 10 locations in Cyprus, Poland, Ukraine, and other geographies globally, Mindy Support’s team now stands strong with 2000+ professionals helping companies with their most advanced data annotation challenges. Contact us to learn more about what we can do for you.