Human Radiologists Still Outperform AI In Cancer Detection
Mammograms are currently the best screening tool for detecting breast cancer early, but reading and interpreting them is a visually challenging task, error-prone for even experienced radiologists. This is one of the healthcare challenges a lot of researchers have been trying to solve with the help of AI. However, a study released this month in the British Medical Journal (BMJ) shows us that AI still has a long way to go towards replacing human doctors. Let’s take a closer look at the results of the study and try to understand the right role of AI in breast cancer detection.
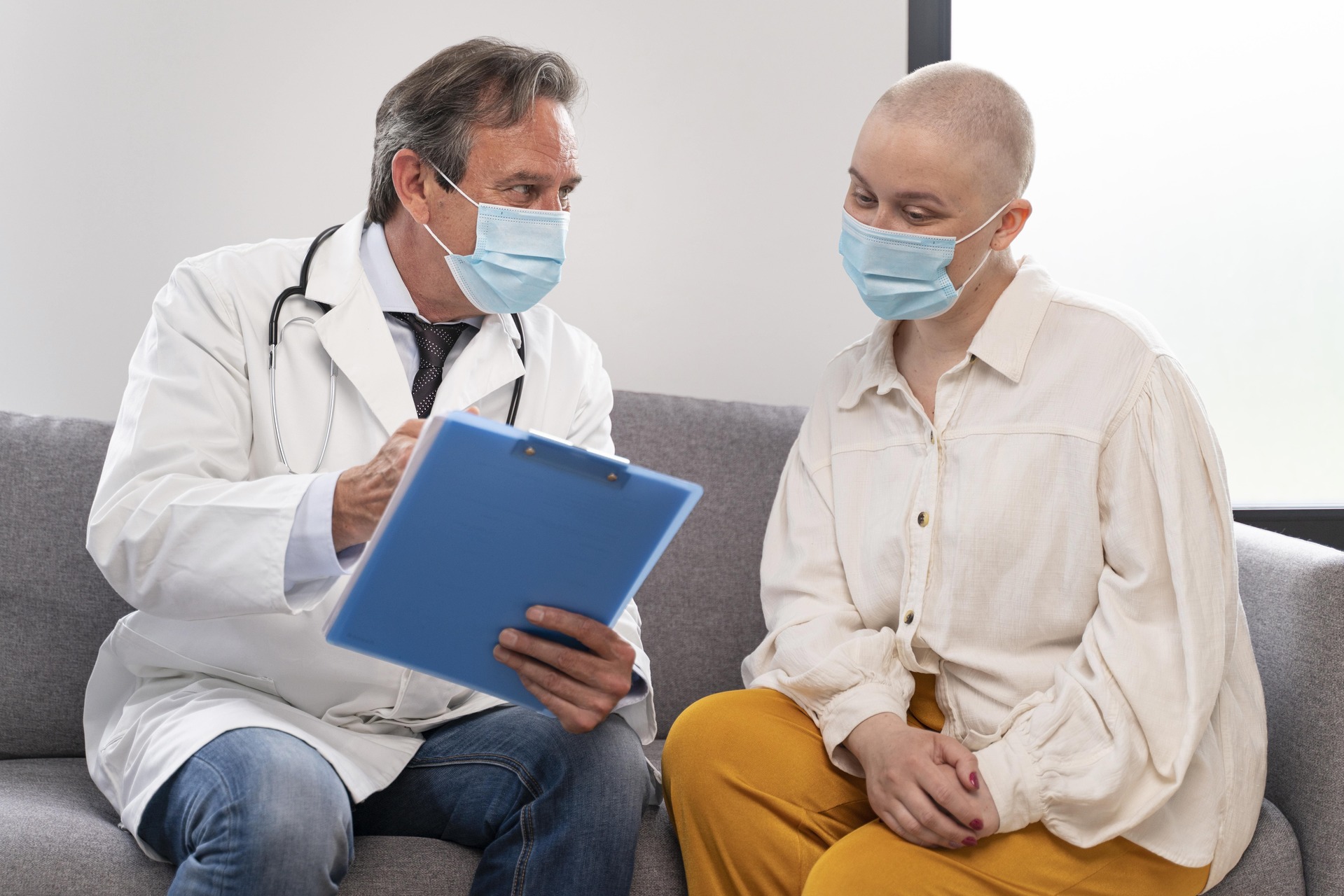
Human Doctors Still Play an Important Role in Breast Cancer Detection
The study in the BMJ found that current AI systems used for breast cancer detection still do not have the necessary accuracy levels to be used in clinical practice. This was the opinion of Sian Taylor-Phillips and colleagues. Taylor-Phillips is with the University of Warwick’s health sciences division in Coventry, U.K.
They did a study that included almost 132,000 women that were screened for breast cancer in Sweden, the United States, Germany, the Netherlands, and Spain. Three large studies involving a total of nearly 80,000 women compared AI systems with the findings of the original radiologist. Of those AI systems, 94% were less accurate than a single radiologist and all were less accurate than the opinions of two or more radiologists, which is the standard practice in Europe.
Five smaller studies involving a total of more than 1,000 women concluded that all of the AI systems they evaluated were more accurate than one radiologist, but the studies had a high risk of bias and their findings were not replicated in larger studies, according to the review.
Could More Accurately Labeled Training Data Help Increase Accuracy?
As we mentioned in the very beginning, developing deep neural networks in the field of radiology is the focus of many research groups. However, while having a refined network architecture is important, access to a large and well-curated dataset is crucial. There are some private and public organizations that offer mammograms that can be used as raw data to train AI systems. For example, the Cohort of Screen-Aged Women (CSAW) includes a total of around 2 million mammography images. However, this raw data needs to be annotated with a high level of accuracy to be useful for researchers.
Mindy Support has experience annotating mammograms and many other medical images such as MRIs, CT scans, and many others. One interesting project we recently worked on involved annotating around 4,000 mammograms. We needed to label biopsied lesions with bounding boxes. Since the data annotators for this project needed to have a medical background, we assembled a team of 4 data annotators with experience in medical data annotation. There was also a team lead with 5+ years of medical experience along with a project manager. We were able to complete the annotations with a 98% accuracy level.
Since the accuracy of the overall system depends on the quality of the data annotation, perhaps this could be a solution for increasing the accuracy of the model. It also goes to show how redundant and tedious tasks like data annotation can have a profound impact on the end product and keep development on schedule. This is why it is a good idea to outsource such tasks to a trusted service provider, like Mindy Support.
What is the Future Role of AI in Healthcare?
While the authors of the study in the BMJ concluded that AI is not ready to be used in clinical practice, this does not mean that we should abandon the idea altogether. The AI field is very dynamic and new AI systems can be perfected with new technology and more accurate data annotation. We are already seeing improvement with AI being able to analyze medical images with products from DeepHealth. A study done by researchers at the University of Massachusetts that was released in January of this year showed a lot of promise.
In this particular study, the deep-learning algorithm performed higher than the expert readers in the diagnosis of both the index cases and the pre-index examinations, with a 17.5 percent increase in sensitivity and 16.2 percent increase in specificity. The deep-learning model also performed better than earlier AI models that were also tested. Therefore, we need to keep in mind that AI technology is constantly being optimized and redeveloped for better performance.
Trust Mindy Support With All of Your Medical Data Annotation Needs
The mammogram labeling project we mentioned earlier is just a small sample of our work in medical data annotation. We have annotated many other medical images for a wide variety of projects with a >98% quality level. The reason we can achieve such a high level of quality is because of the QA process we have in place, our experience, and the training we provide to our annotators. We can also scale your projects quickly, as needed since we already have 2,000 employees in 6 locations all over Ukraine and other locations all over the word.