Everything You Need to Know About Video Annotation
Category: Best practice articles
Published date: 23.03.2023
Read time: 9 min
Self-driving cars pose estimation, and many other extraordinary applications of computer vision use videos as their data are just a few examples. As a result, video annotation is essential for developing computer vision algorithms. Annotating videos requires a lot of attention to detail and expertise. In this article, we will take a closer look at video annotation and explore the various types of video annotation and the industries that use them to create AI products.
What is Video Annotation?
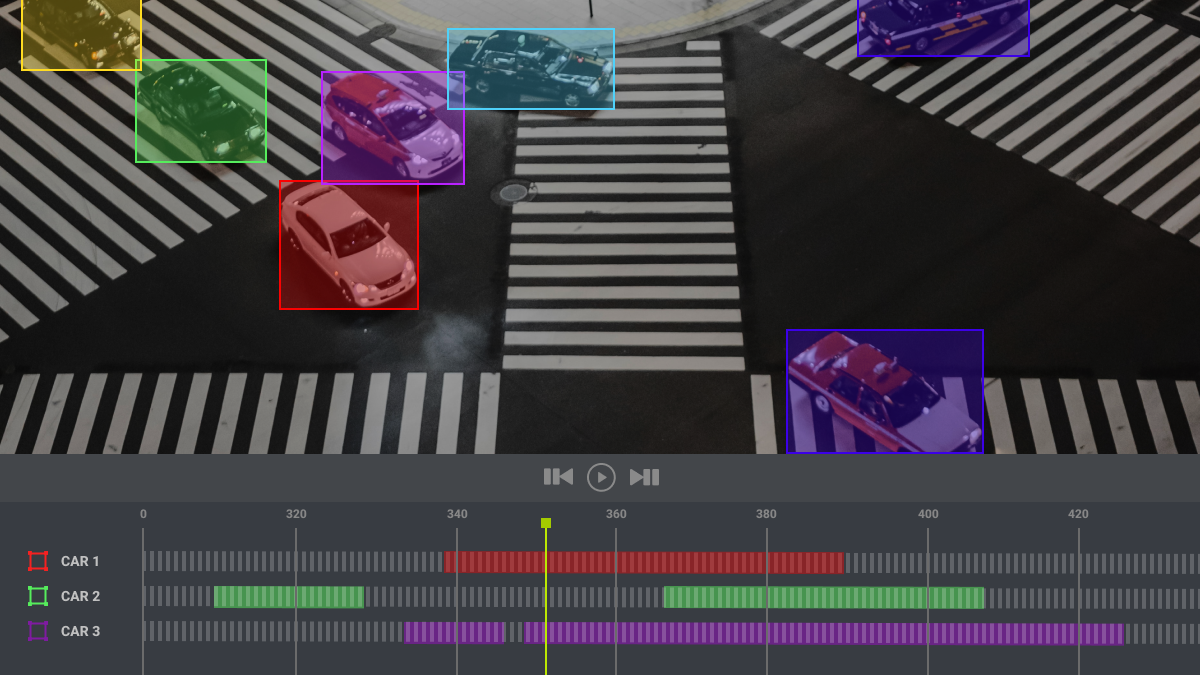
The process of labeling or categorizing video clips that are used to train computer vision models to recognize or identify objects is known as video annotation. Contrary to image annotation, frame-by-frame annotation is used in video annotation to identify items for machine learning models.
Ground truth databases are created by high-quality video annotation in order to maximize machine learning performance. Numerous deep learning uses exist for video annotation in a variety of fields, such as autonomous driving, medical AI, and geospatial technology.
What are the Various Types of Video Annotation?
A number of techniques are used to annotate videos, including landmark, semantic segmentation, 3D cuboid, polygon, and polyline annotation. Let’s take a look a look at all of these video annotation types in greater detail:
SEMANTIC SEGMENTATION
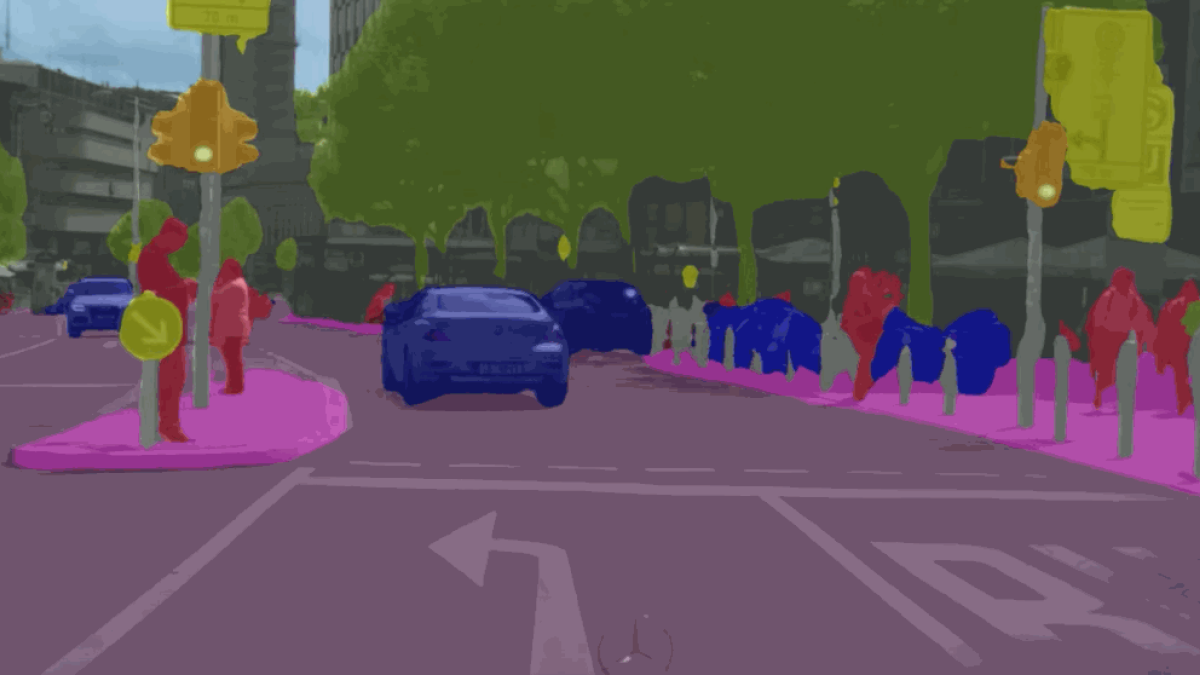
Another form of video annotation that aids in improving artificial intelligence model training is semantic segmentation. This technique assigns each pixel in an image to a particular class. Semantic segmentation handles multiple objects of the same class as one entity by giving a label to each image pixel. However, multiple objects belonging to the same class are handled as separate individual instances when instance semantic segmentation is used.
LANDMARK ANNOTATION
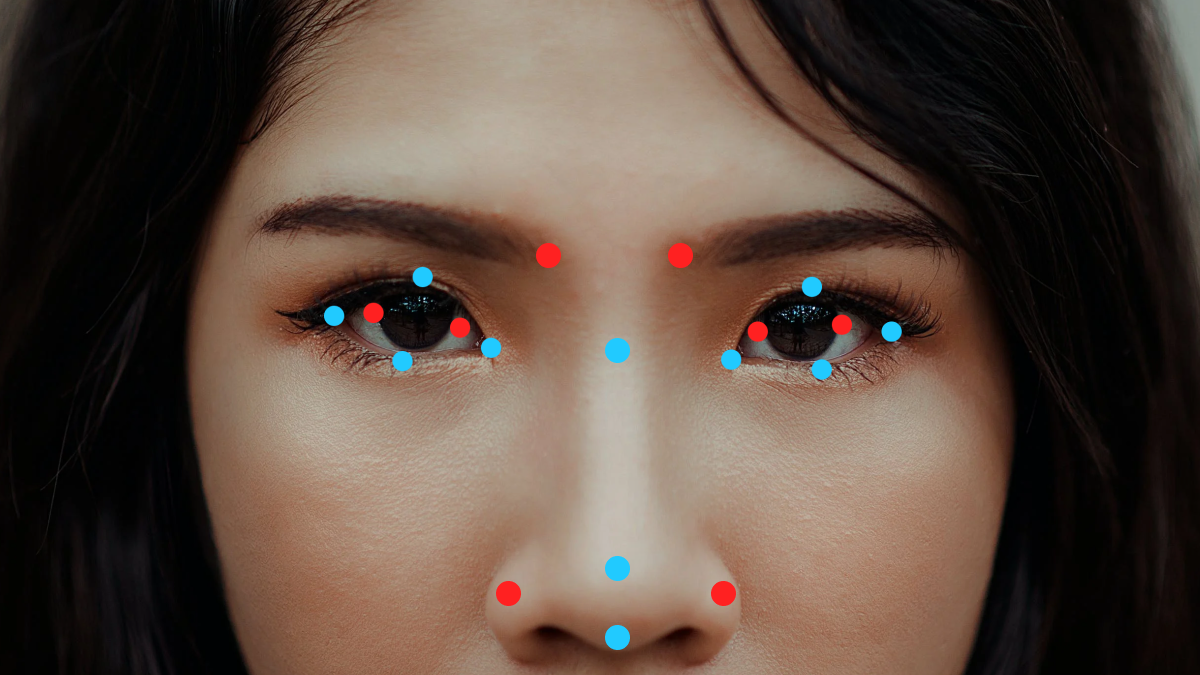
Also known as keypoint annotation, it is generally used to identify smaller objects, shapes, postures, and movements. The object is covered with dots that are connected together to form a skeleton that is visible in every movie frame. For the creation of AR/VR apps, facial recognition software, and sports analytics, this type of annotation is primarily used to identify facial features, poses, emotions, and human body parts.
POLYGONS
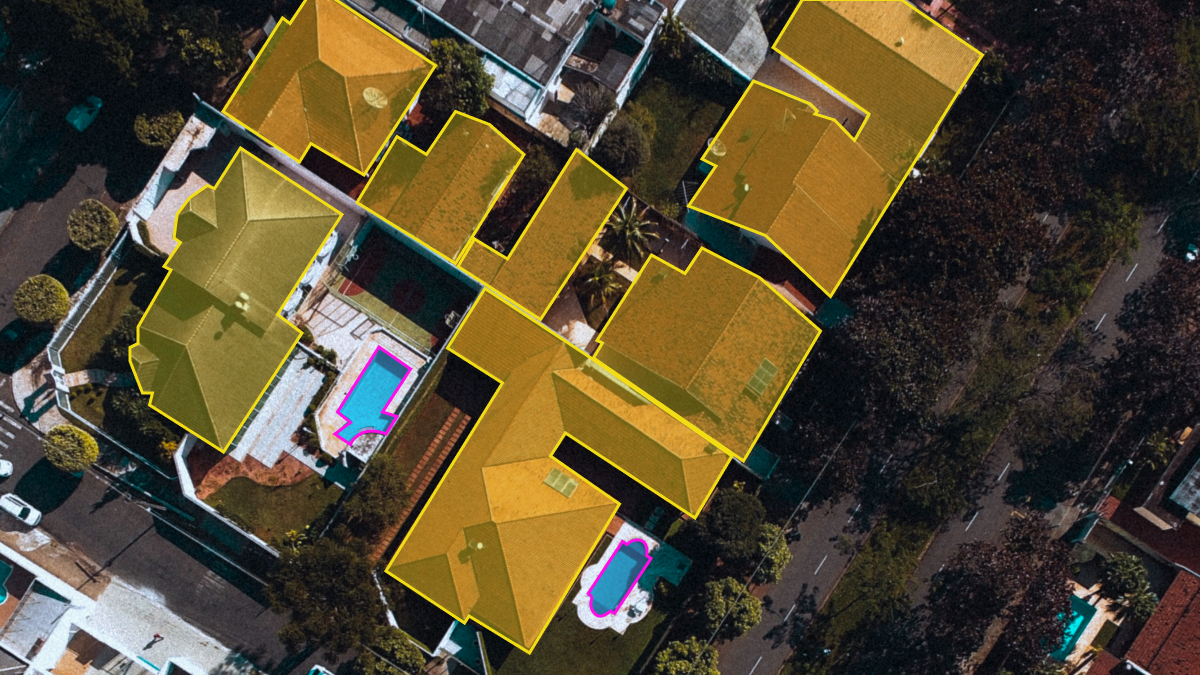
When a 2D or 3D bounding box technique is found to be inadequate to accurately measure an object’s shape or when the object is moving, the polygon annotation technique is typically used. An irregular object, like a person or an animal, is apt to be measured using polygon annotation, for instance. The annotator must exactly place dots around the edge of the object of interest in order to draw lines around it using the polygon annotation technique.
3D CUBOIDS
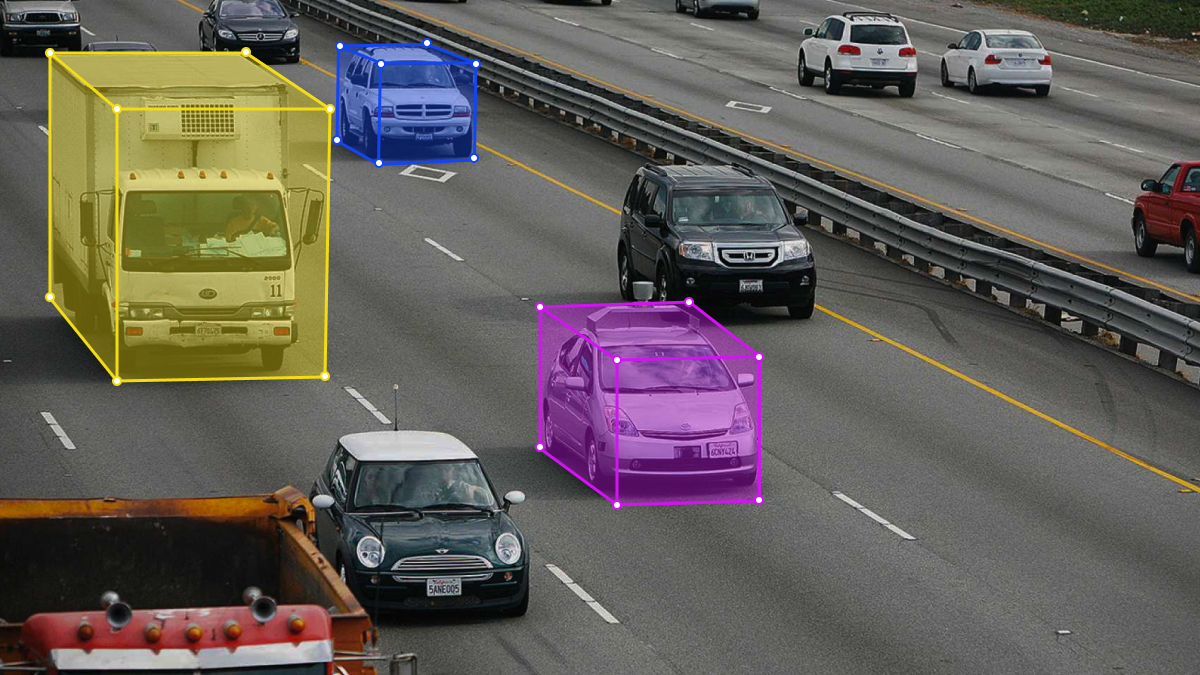
An accurate 3D representation of objects is achieved using this style of annotation method. When an item is moving, the 3D bounding box method labels its length, width, and depth and examines how it interacts with its surroundings. In relation to its three-dimensional surroundings, it aids in determining the object’s location and volume. Beginning with bounding boxes around the item of interest, annotators place anchor points at the box’s edge. By measuring the estimated length, height, and angle in the frame, it is possible to determine where the edge might be during motion if one of the object’s anchor points is obscured or out of view due to another object.
POLYLINES
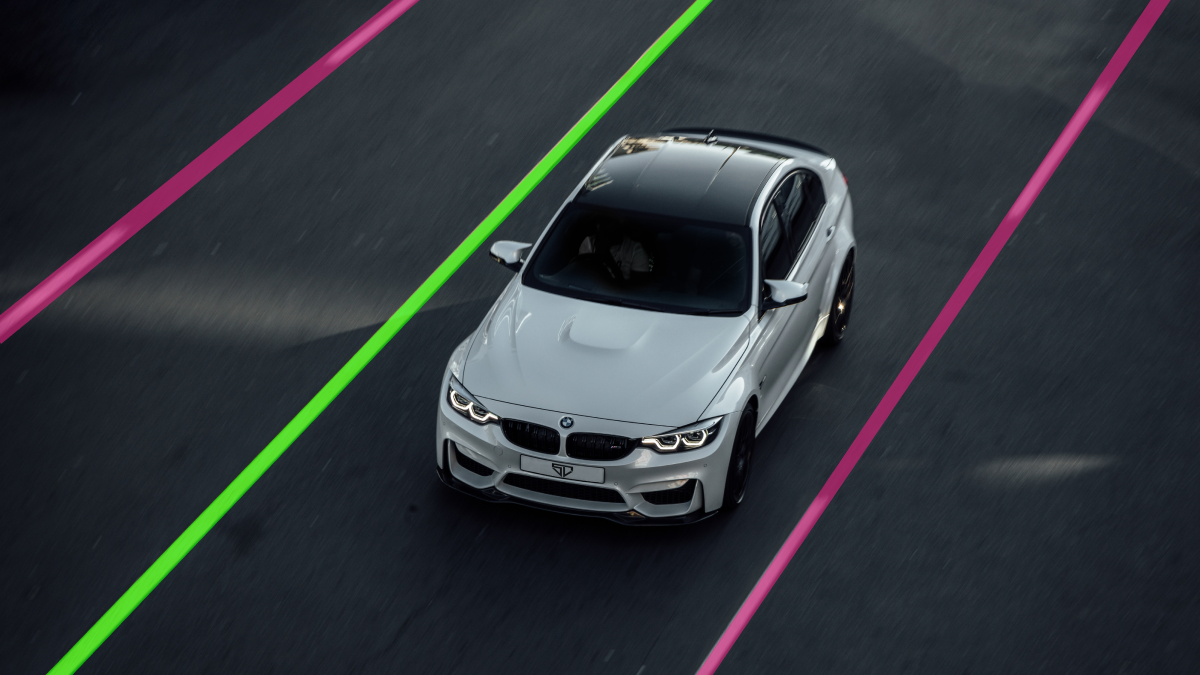
In order to create highly accurate autonomous vehicle systems, polyline annotation helps teach computer-based AI tools to recognize street lanes. The computer uses lane detection, border detection, and boundary detection to enable the machine to see the path, traffic, and diversion. In order for the AI system to recognize lanes on the road, the annotator sketches exact lines along the borders of the lanes.
In Which Industries Would Video Annotation Be Used?
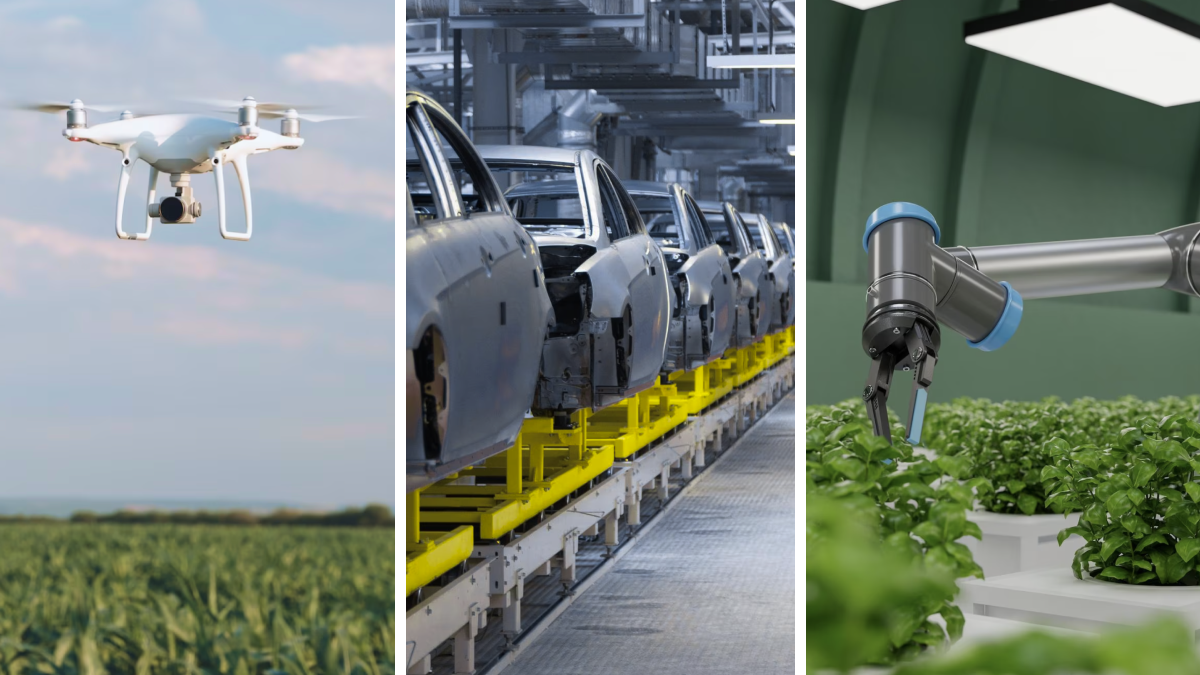
Although it seems like video annotation has limitless potential, some sectors use it much more than others. However, it is undeniably true that there is still more to come in terms of innovation and that we have only just begun to scratch the surface. In the next section, we’ve enumerated the sectors that use video annotation more often:
- Automotive – AI systems with computer vision capabilities aid in the development of autonomous and automated vehicles. The development of sophisticated autonomous vehicle systems that can identify objects like signals, other cars, pedestrians, street lights, and more has made extensive use of video annotation.
- Agriculture – Artificial intelligence and computer vision technologies are being used to advance crops and livestock. Additionally, video annotation is used to better understand and monitor plant development, observe livestock movement, and enhance the efficiency of harvesting equipment. Among other things, computer vision can assess the quality of grains, weed development, and herbicide use.
- Robotics – From meticulously annotated datasets of images and videos, highly functional industrial robots learn to identify objects and map their route without running into any barriers. A computer vision model can identify things in its environment with the help of video annotation and can then interact with those objects or avoid them as needed. This technology has the potential to significantly enhance safety on the assembly line and boost manufacturing productivity.
Mindy Support Video Annotation Customer Cases
We already know there are many different types of video annotation, but what are the practical applications? Let’s take a look at some use cases from our experience.
HELPING OUR ClIENT EXTRACT INSIGHTS FROM HOCKEY MATCHES WITH EVENT ANNOTATION
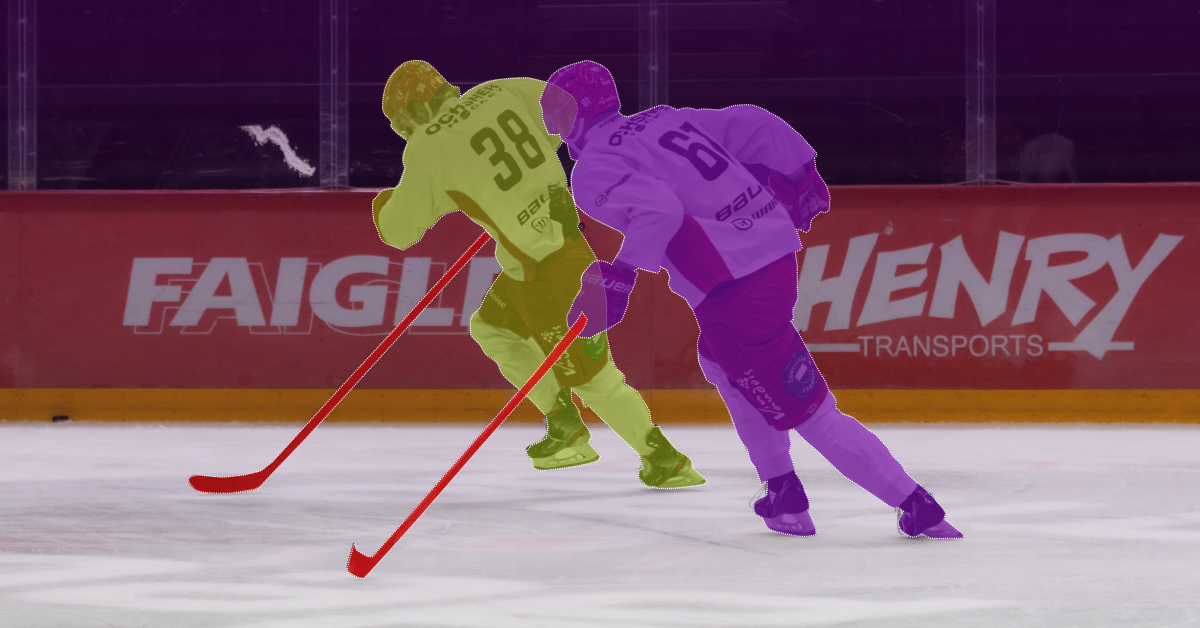
The first use case is from a company that provides professional and junior hockey teams from all around the world with the most comprehensive advanced analytics that continuously evolve with the sport. The company tracks and analyzes a lot of indicators of the hockey games, like the amount of time players spend on the ice, the number of face-offs, puck possession time, the number of penalty shots, etc. They needed us to help annotate all of these events in the videos. Learn more about our work here in our case study
ANNOTATING THE MOVEMENT OF PEOPLE USING KEYPOINTS
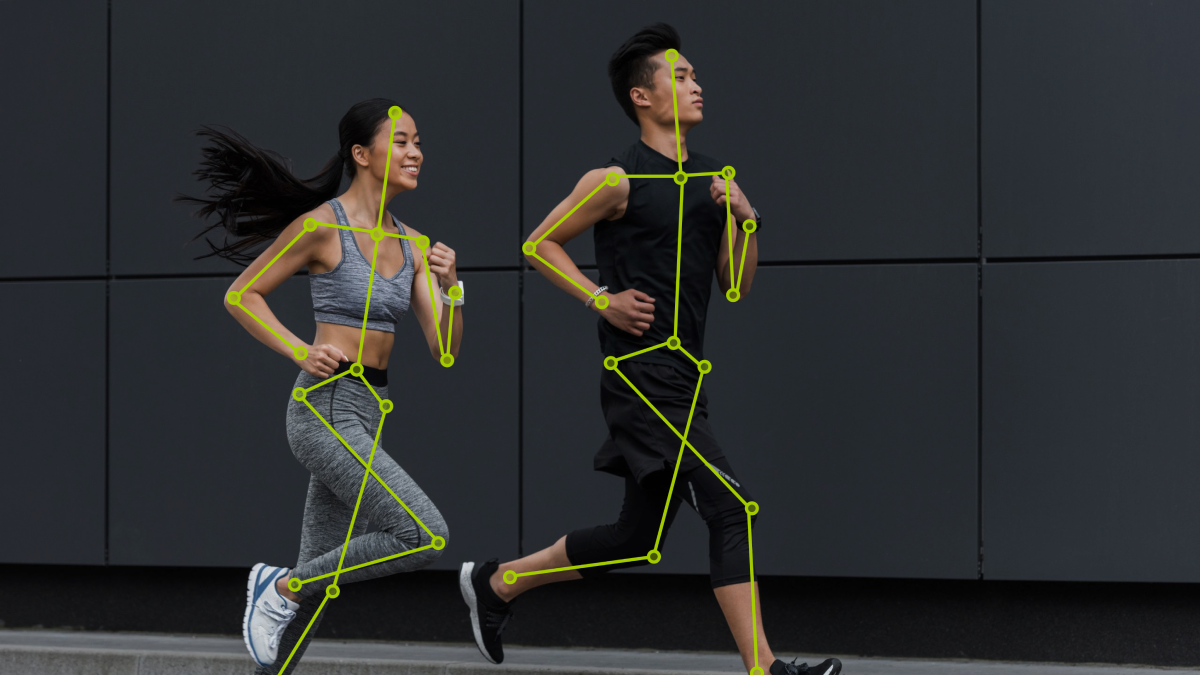
The next use case is from our client in the US who needed to annotate the human skeleton with key points on each frame in a video which is very challenging because the person is constantly moving in the video and therefore the combinations of points are constantly changing. We hired a team of 115 full-time data annotators to perform all of the needed labeling tasks. In total we annotated 12,000 videos which is 1,200,000 frames. Check out the results we were able to achieve in our case study.
ANNOTATING ABRUPT MOVEMENTS FOR ENHANCED SECURITY ON PUBLIC TRANSPORT
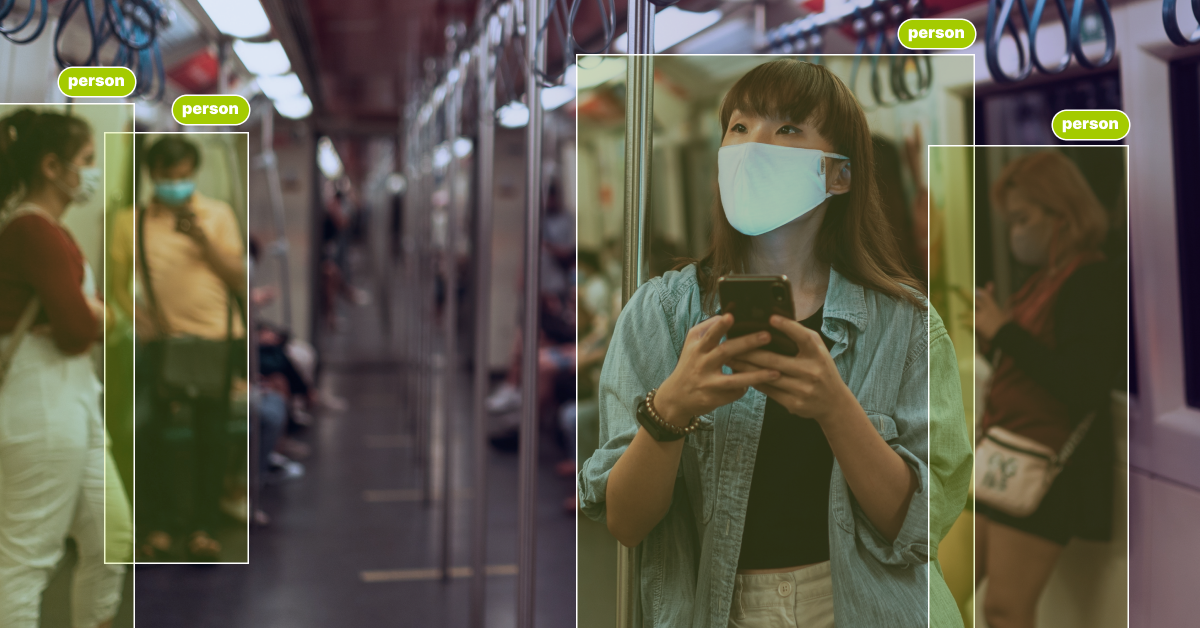
Finally, we want to tell you about the work we did for a client from France who was developing a tool that could increase passenger security on buses and trains. The tools would recognize abrupt and sudden movements which could be used to train security officers to identify potential threatening actions by passengers. We needed to annotate a sizable dataset of 500 videos containing various abrupt actions and poses. This was a really interesting work we did that you can read about in our case study.
Trust Mindy Support With All of Your Data Annotation Needs
Mindy Support is a global provider of data annotation and data preparation services, trusted by Fortune 500 and GAFAM companies. With more than 10 years of experience under our belt and offices and representatives in Cyprus, Poland, Romania, Bulgaria, India, OAE, and Ukraine, Mindy Support’s team now stands strong with 2000+ professionals helping companies with their most advanced data annotation challenges.