Training Autonomous Vehicles in a Virtual Environment
Category: AI Insights
Published date: 28.04.2022
Read time: 6 min
Training autonomous vehicles requires massive amounts of training data in the form of videos or images which later need to be annotated to train the machine learning algorithms. However, obtaining the needed training data can be challenging, especially if you consider how many driving scenarios an autonomous vehicle can encounter on the road. If you need images or videos of very specific situations, how would you go about obtaining this data? One company, Wasabi World, is trying to simplify this process by offering a very creative solution. Let’s take a look at the virtual environments they created to understand how they can be used to train autonomous vehicles.
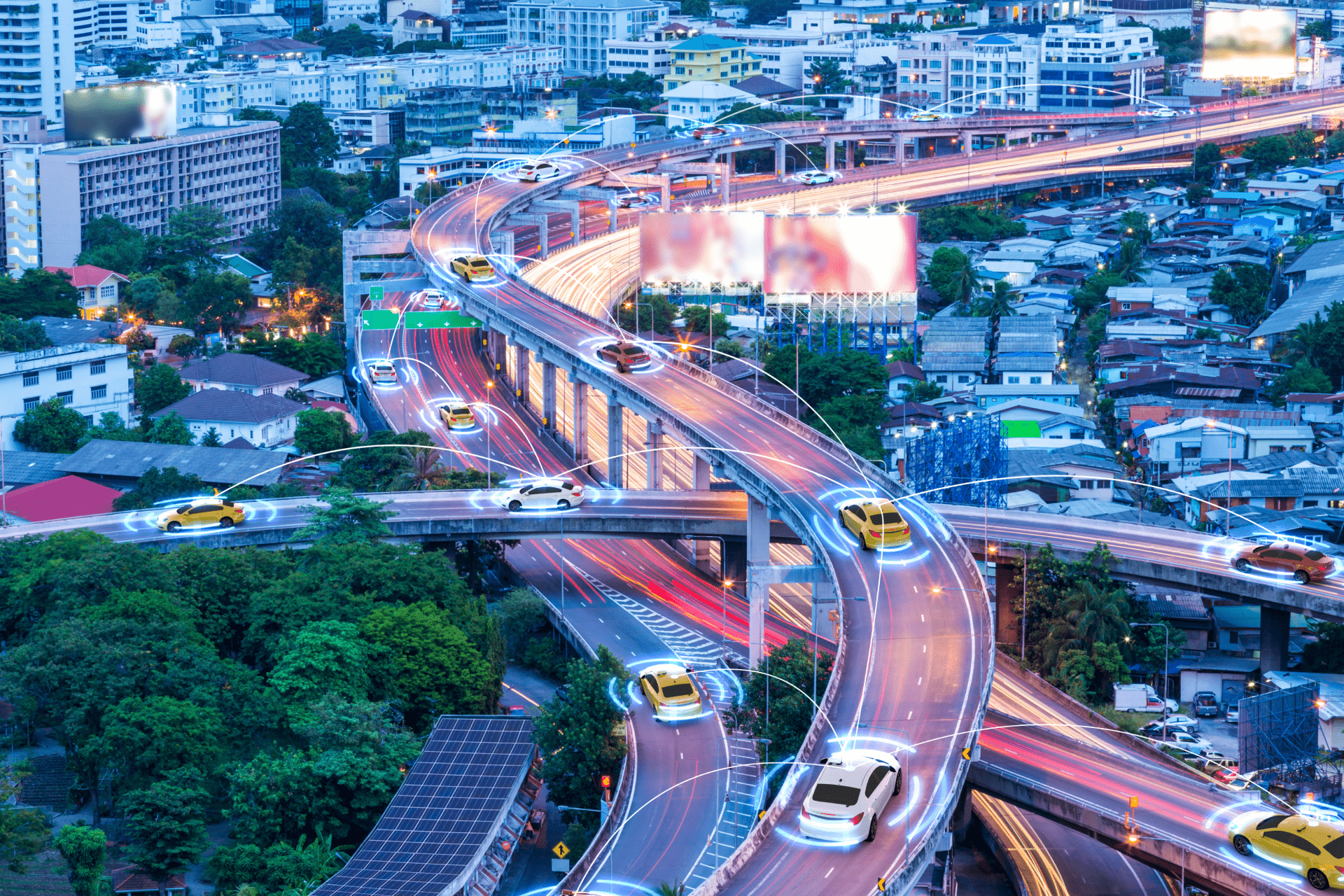
How Can Virtual Environments Help Train Autonomous Vehicles?
When you get behind the wheel, a mix of intuition, instinct, and learned skills helps you process what’s happening and make instantaneous decisions about how to navigate obstacles, when to slow down, speed up, stop, and much more. The human brain’s ability to do all this is remarkable. Realizing the promise of self-driving technology requires us to teach the “brain” of self-driving vehicles to do exactly the same—while eliminating the risks of distraction, fatigue, and other human-specific vulnerabilities. If a company tried to collect the needed training data that would allow the machine learning algorithms to comprehend the world like a human, it would need to drive millions of miles for thousands of years to experience everything necessary to learn to drive safely in every possible circumstance.
This is why Wasabi World decided to create a virtual world where it will be possible for companies to test the AI software. While it is not the first company to create such virtual environments, it does take them to the next level since the world itself is generated and controlled by AI, which acts as both driving instructor and stage manager—identifying the AI driver’s weaknesses and then rearranging the virtual environment to test them.
Additional Problems Solved by Virtual Environments
Sometimes the autonomous vehicles will encounter uncommon situations like a bicyclist trying to drive across the road or the big truck occluding certain details of the road ahead. These are just some of the many possibilities and to test them all correctly would require thousands of driving miles. Therefore, you cannot rely on real-world testing alone since such situations will not happen all that frequently. The virtual environment could generate pretty much any driving scenario you need and even use real-world camera data from its cars to make the simulations more realistic.
Researchers will then be able to change all kinds of parameters such as the vehicle type, road layout, number of pedestrians, and anything else. Testing with this kind of synthetic data is 180 times faster and millions of dollars cheaper than using real data.
Disadvantages of Using Virtual Environments
Having said this, there are some downsides to using such simulated environments. Low-fidelity simulators may evoke unrealistic driving behavior and therefore produce invalid research outcomes. The AI can find glitches in the simulation that let them defy physics by launching themselves into the air or pushing objects through walls. While automotive simulators have come a long way and simulation has now become a cornerstone in the development of self-driving cars, common standards to evaluate simulation results are lacking. For example, the annual mileage Report submitted to the California Department of Motor Vehicle by the key players such as Waymo, Cruise, and Tesla does not include the sophistication and diversity of the miles collected through simulation. It would be more beneficial to have simulation standards that could help make a more informative comparison between various research efforts.
Further, there are no simulators currently available that are capable of testing the concept of connected vehicles, where vehicles communicate with each other and with the infrastructure. However, there are testbeds available.
Data Annotation is Still Needed Even With Virtual Worlds
Even though all of the scenarios encountered by the vehicles are simulated, they still need to be able to recognize all of the items in the virtual environment such as other vehicles, street signs, pedestrians, and many other things. This requires data annotation methods such as semantic segmentation, 2D/3D bounding boxes, and labeling to train the machine learning algorithms to recognize all of the objects on the road. Since this is a very time-consuming process, a lot of companies choose to outsource data annotation to Mindy Support.
Trust Mindy Support With All of Your Data Annotation Needs
If you are creating an AI product that requires large volumes of data annotation, consider outsourcing such work to Mindy Support. We are a global company for data annotation and business process outsourcing, trusted by several Fortune 500 and GAFAM companies, as well as innovative startups. With nine years of experience under our belt and offices and representatives in Cyprus, Poland, Romania, The Netherlands, India, and Ukraine, Mindy Support’s team now stands strong with 2000+ professionals helping companies with their most advanced data annotation challenges. Contact us to learn more about what we can do for you.