Skim Through Research Papers With This AI Tool
Category: AI Insights
Published date: 09.11.2023
Read time: 6 min
As you write your research paper, you’re searching for publications that will bolster your claims. You don’t have endless time to read every article available, yet you still want to locate excellent supporting material. A good technique to determine an article’s applicability to your research paper and whether it’s worthwhile to read through to the end is to skim it. Best of all, there is an AI tool that will help you do just that.
In this article, we will take a look at a new AI tool that helps researchers skim through scientific papers and the data annotation required to create this tool.
Skim Long Texts With AI
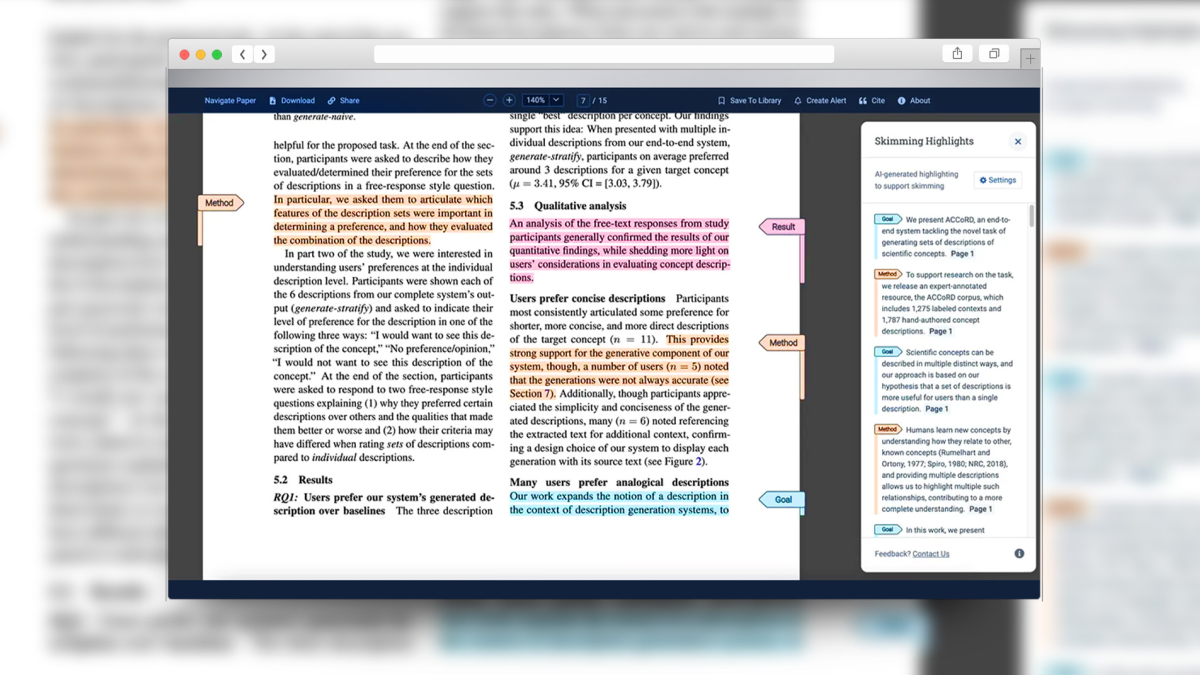
With the growth of knowledge work and the information explosion that followed, specialists are expected to go through and interpret vast amounts of quickly changing data. The field of scientific study is one where this tendency is very noticeable. It takes a lot of work for researchers to stay current with the literature. They accomplish this by going about their daily business of gathering papers, scanning or reading the ones they think are most pertinent, and entering the information they learn from reading into their own records.
Semantic Scholar, a research and product development team within AI2, has released a new tool as part of its Semantic Reader that enhances skimming. This AI-powered application helps students concentrate on their reading and develop their research skills over time by automatically pre-highlighting and color-coding a research paper to highlight the objectives, methodology, and important findings.
The tool classifies sentences into four categories: objective, novelty, results, and method. It was trained with the CSAbstruct dataset, a corpus of abstracts from computer science papers with manually-curated “gold” labels. Since sentences only came from paper abstracts, we ultimately found the model insufficient for classifying sentences from the body of papers. This is why the dataset was further improved by labeling negative sentences selected from full papers.
How Do These AI Text Skimming Tools Work?
When a user inputs text into the AI tool, it extracts metadata, section headers, mathematical symbols, and textual tokens. After that, it divides the tokens into sentences while also combining the bounding boxes of the tokens and sentences at the same time. Every sentence has a label that corresponds to its section header and paragraph index; these labels are used to prioritize which sentences should have highlights shown.
The text is then classified into the four categories mentioned in the previous section while highlighting each of these sections with a distinct color. A reader can choose to enhance or decrease the density of highlights to more thoroughly examine a paper or to conduct a quick, high-level scan of it. A reader has the option to disable highlights of a specific aspect if they would prefer not to go through a specific type of content as they skim (for example, they may want to know about a study’s findings but not its methodology). The sidebar has sliders for each facet that readers can use to adjust the density of highlights, which appear and disappear in the paper. Markers also appear and disappear in the scrollbar, and a count of highlights changes next to the slider so that the reader can see the influence on highlight density.
What Types of Data Annotation are Needed to Train These AI Models?
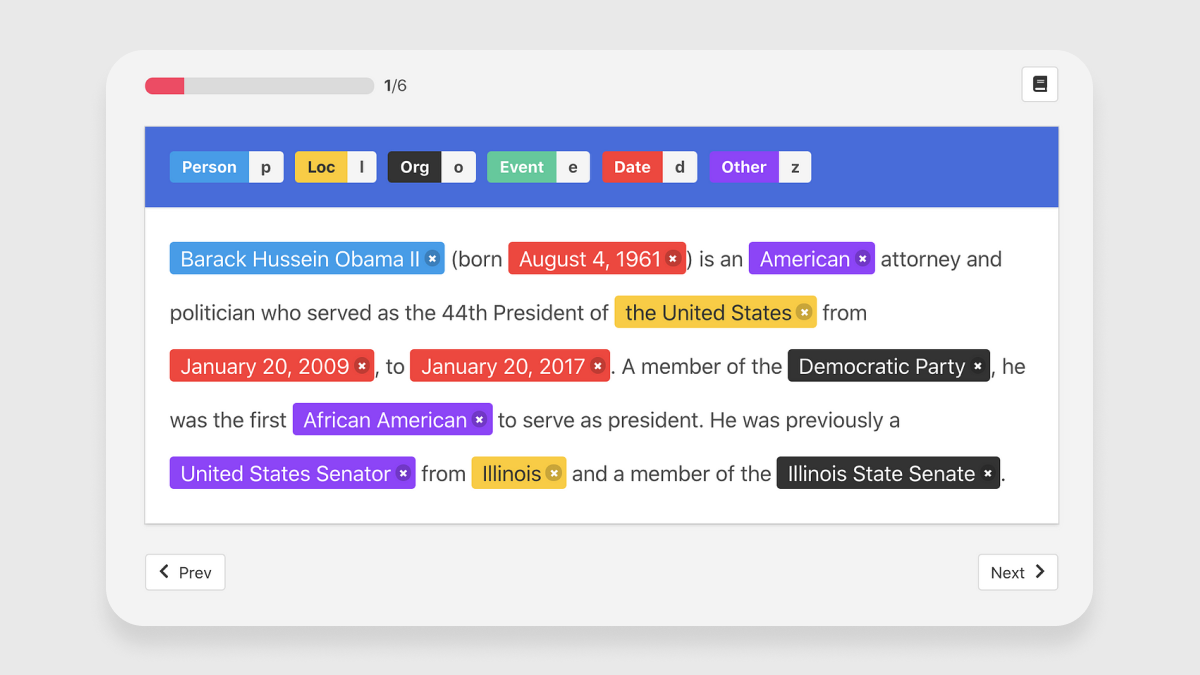
The AI skimming tools rely on recognizing and classifying text, which means that various text annotation techniques will need to be performed to train these AI models. This includes things like entity annotation, which is one of the most crucial steps in creating training datasets for chatbots and other NLP applications. It involves finding, extracting, and tagging textual items and encompasses named entity recognition, keyphrase tagging, and part of speech tagging.
In addition to this, all the annotated entities will need to be linked, which is another form of text annotation. Here, entities are linked to larger repositories of data about them.
Sentiment annotation is also necessary, which is the labeling of a text’s internal feelings, opinions, or sentiments. Texts are provided for annotation, and the task is to select the label that best captures the sentiment or viewpoint expressed in the text. An easy illustration would be to examine customer reviews. After reading the reviews, commentators would categorize them as favorable, neutral, or unfavorable.
Trust Mindy Support With All of Your Data Annotation Needs
Mindy Support is a global provider of data annotation services and is trusted by Fortune 500 and GAFAM companies. With more than ten years of experience under our belt and offices and representatives in Cyprus, Poland, Romania, The Netherlands, India, OAE, and Ukraine, Mindy Support’s team now stands strong with 2000+ professionals helping companies with their most advanced data annotation challenges.