Paving the Way for High Quality Training Data
Category: AI insights
Published date: 19.07.2021
Read time: 7 min
Acquiring contextually labeled, high-quality training data is one of the most important aspects of your machine learning project. However, in addition to simply having the right tools and technology, you will also need to have the necessary expertise to attain the needed level of quality. There are a lot of processes that go into annotating massive amounts of data while maintaining quality control and you cannot underestimate this function of the model development process. Making sure that everything is done correctly requires a tremendous amount of resources and specialized expertise.
This is why a lot of companies developing AI and ML projects trust Mindy Support to deliver the data quality they need. Today we will share with you some of our quality management practices that we have put in place based on years of experience labeling tens of millions of images. This step-by-step guide will help explain the fundamentals of data quality and how Mindy Support works with you at every stage to ensure the needed level of quality.
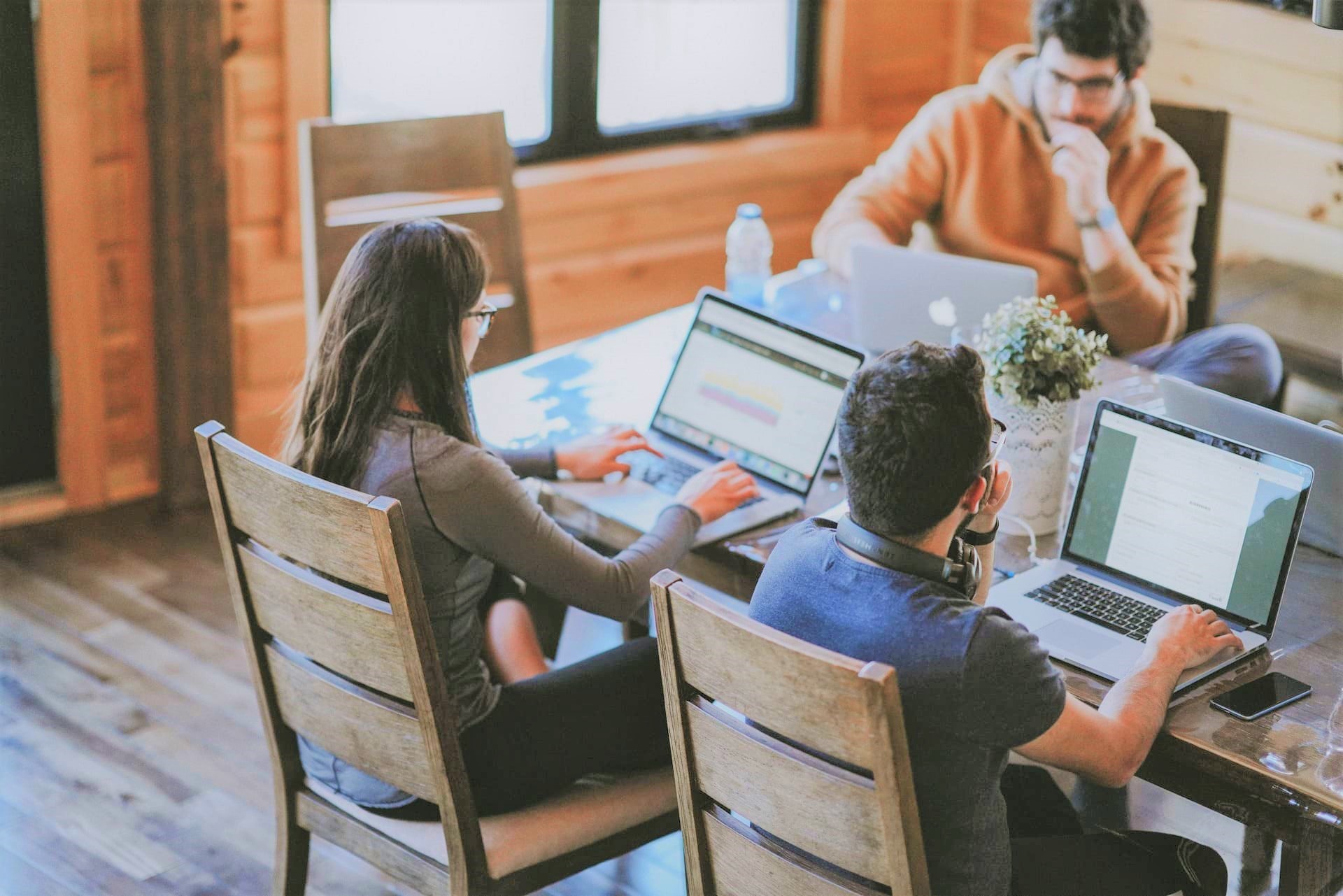
1. Setting the Requirements
Before you can start measuring the quality, you need to have clear rules in place that clearly define what quality means for your particular project. Therefore, when you are labeling thousands and thousands of images, videos, or audio recordings, they need to be compared to and measured against the ground truth data. This is how you will evaluate the quality of the dataset as a whole. The difference between the ground truth and the work performed by the data annotators will be the basis for all the quality metrics. The most common metrics include:
- Accuracy – This is a measurement of correctness. If you take all of the objects in the dataset, were the correct objects annotated? Were all of the true positives and negatives annotated correctly? Add up these categories together and then divide them by the total number of objects to get the accuracy score:
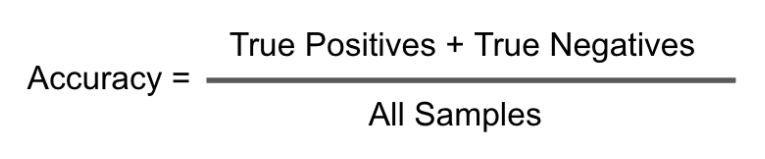
- Precision – This is a measurement of exactness. Did the annotators capture only the true positives or did they incorrectly capture false positives? Take the number of correctly annotated objects and then divide this by the total annotations to get the precision score. The formula for precision is as follows:
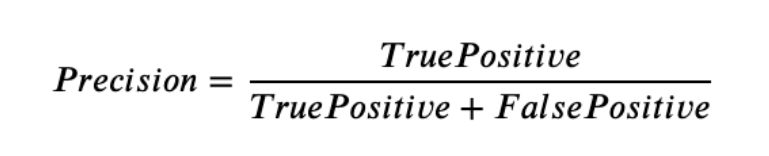
- Recall – This is a measurement of completeness. Have the annotators failed to capture some of the objects that met the necessary criteria? Take all of the objects that were annotated correctly and then divide this by the number of objects that should have been annotated to get the recall score. The formula for recall is as follows:
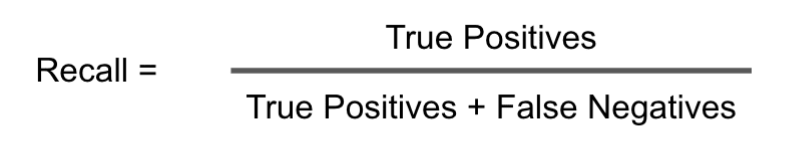
- F1 Score – The F1 score tries to strike a balance between precision and recall and tries to understand how well you are capturing all and only the objects you are supposed to annotate. The F1 score can be measured via the following formula:
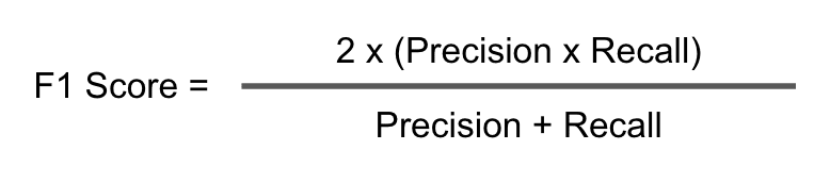
2. Managing Your Workforce and Platform
In the first step we talked about how you can measure the quality of the annotations, but you need to provide your data annotators with the proper training curriculum so that they understand the criteria domain context. At Mindy Support, we have an extensive training process that ensures that everybody understands how to properly annotate the dataset and also provides continuous training and quality assurance. If we see that a particular person is not meeting the needed quality level, we will conduct a separate training session for them.
In addition to this, we also have the technological expertise to configure the annotation platform to match the workflow. This involves configuring the labeling tools, classification taxonomies, and annotation interfaces for accuracy and throughput. We can also implement automatic QA checks if the annotation platform allows for this.
3. Establishing Quality
Since there is no one-size-fits-all solution that will be able to meet the requirements of each individual project, the risks that come with a loose set of standards should guide you in terms of the requirements. For example, you might use several different annotators to reach the target quality or conduct complex reviews against the ground truth. You may even wish to escalate the review to a subject matter expert. At Mindy Support, we have very rigorous QA standards that help us do the job right the first time. We have a dedicated staff covering such functions as Project, Quality, Operations, QA Managers, Trainers, and Team Leads.
The QA team takes the gold data or the ground truth for evaluating the performance of the data annotators. There are also some projects, for example in the healthcare industry, where a subject matter expert will also be involved in the QA process.
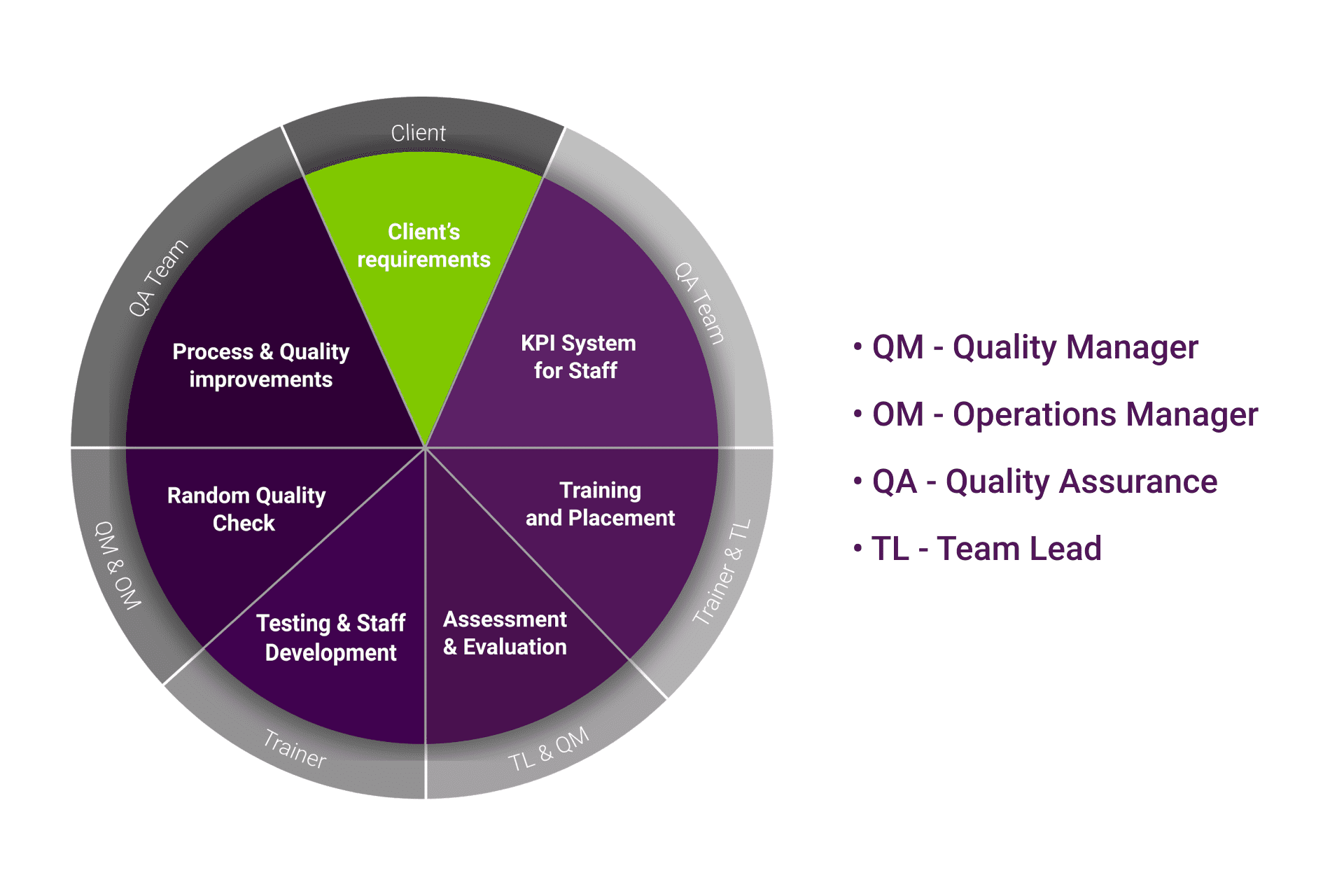
4. Iterate and Scale
In the last stage of the quality training data pipeline, you will need to fine-tune and optimize existing processes. With every iteration you need to keep track of the annotation throughput i.e. how long does it take to move through the process? Are things moving along faster? Now, think about the scalability of your process since the requirements might change as the project scales. This means that you will need to conduct ongoing employee training and scoring, which should include more edge cases.
Thanks to all of the experience we have gathered over the past eight years, Mindy Support is able to scale projects without sacrificing the quality of the annotation work.
Trust Mindy Support With All of Your Data Annotation Needs
Mindy Support understands the importance of high-quality training data to the overall success of the project and will help you avoid costly delays or redoing any of the work. Our QA process ensures that all of the work will be done right the first time and our size and location allow us to source and recruit candidates quickly making it easier to scale your project. Contact us to learn about what we can do for you.