New Methodology of Extracting 3D Information From 2D Images
Category: AI insights
Published date: 09.10.2023
Read time: 6 min
In order to help artificial intelligence (AI) retrieve 3D Information from 2D photographs, scientists have developed a new technique, making cameras an extremely useful tool for such future technologies. This work is highly relevant to many applications, especially those involving driverless vehicles. For autonomous vehicle designers who can add several cameras to provide system redundancy, cameras are a more cost-effective alternative to other instruments like LIDAR, which uses lasers to estimate distances.
The success of this redundancy, however, rests on the AI’s capacity to decode 3D navigational data from the 2D images that these cameras record. By enabling the extraction of 3-D data from 2D photos, a new method called MonoXiver significantly contributes to solving this problem and improving the capabilities of autonomous cars.
In this article, we will take a look at this new technique and the data annotation that needs to go along with to train AI models.
What is the Current Approach?
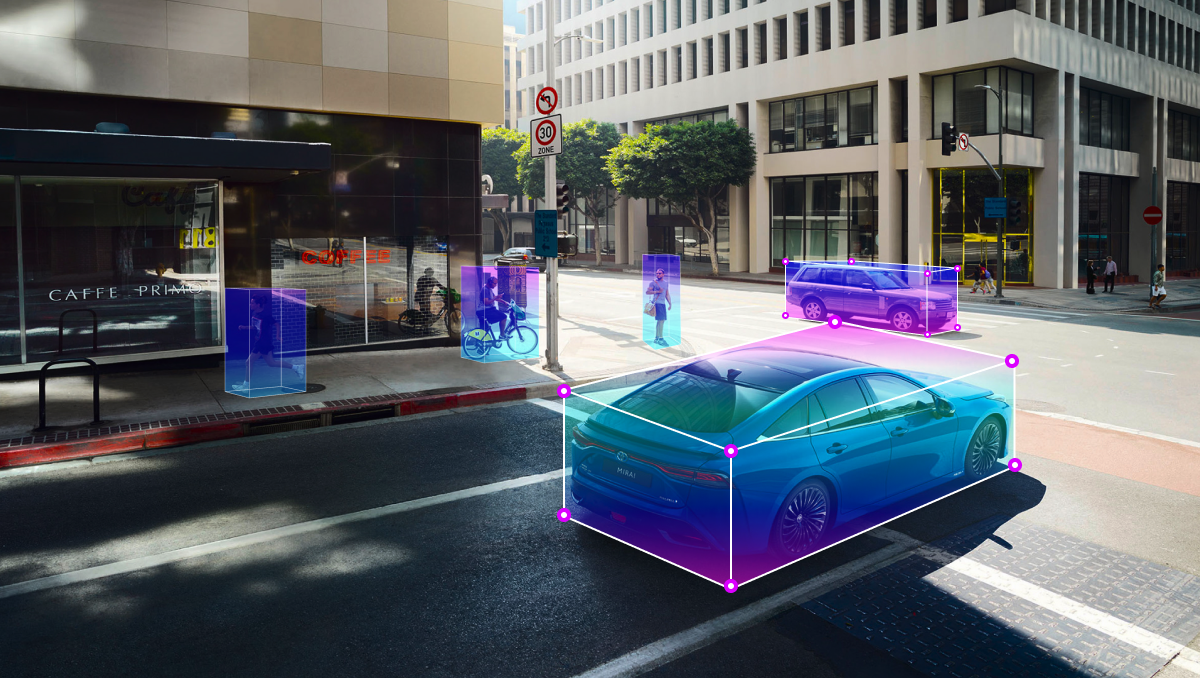
Existing techniques that try to extract 3D Information from 2D photos, such as the MonoCon approach, rely on the usage of bounding boxes. Through these methods, AI systems are taught to examine 2D images and draw 3D bounding boxes around specific objects, such as individual vehicles on a street. These bounding boxes are represented by cuboids, which are eight-pointed three-dimensional rectangles that resemble the corners of a shoebox. These bounding boxes’ purpose is to help the AI estimate the size of objects in the image and determine the spatial relationships between them.
Bounding boxes essentially aid the AI in determining the size of an automobile and its location in relation to other moving cars on the road. While this method can be pretty effective, we can improve on it with the MonoXiver approach, which will be discussed in the next section.
How Does the MonoXiver Method Improve 3D Information Extraction?
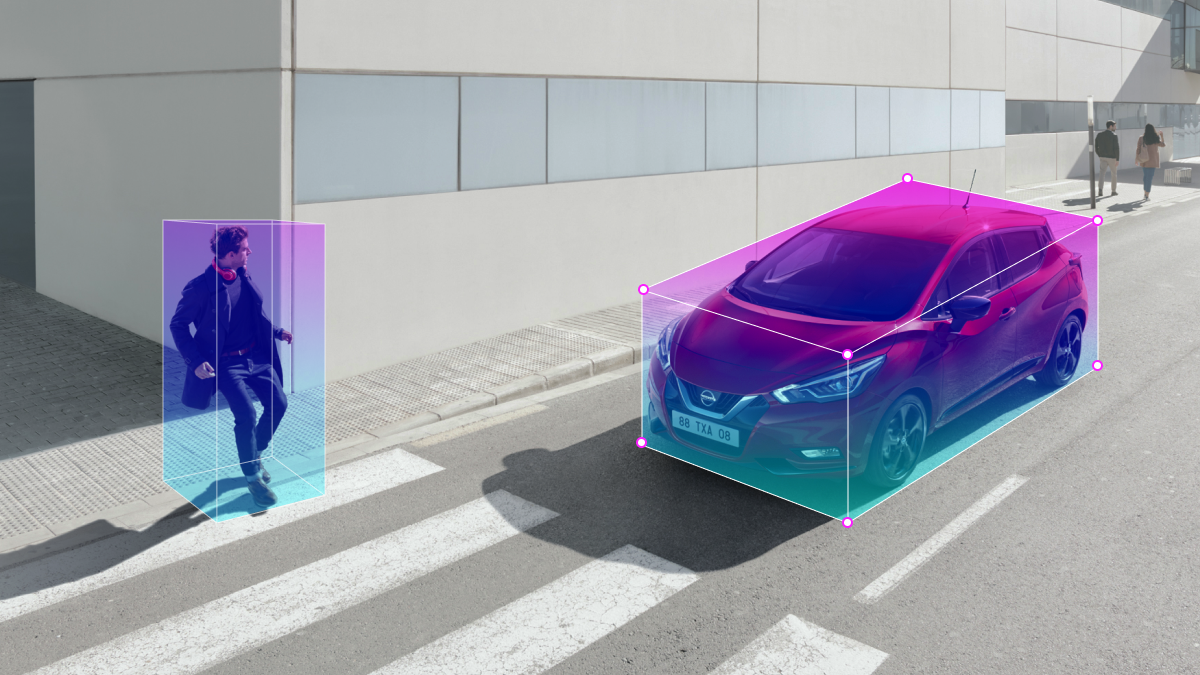
The MonoXiver technique adopts a different approach than previous programs, where bounding boxes can be imperfect and may not fully enclose all portions of a vehicle or item present in a 2D image. Each bounding box is used as a starting point and anchor for the AI’s study. The AI then does a second analysis of the area surrounding each anchor bounding box. The anchor is surrounded by numerous additional bounding boxes as a result of the secondary investigation.
The AI makes two crucial comparisons to determine which of these secondary boxes best captures any missing parts of the object. The initial comparison evaluates each secondary box’s “geometry” to see if it contains forms that match those in the anchor box. The second comparison looks at each secondary box’s “appearance” to see if it has colors or other visual traits that closely reflect those in the anchor box. With the aid of this thorough strategy, MonoXiver is better able to estimate object dimensions and positions and improve the accuracy of object detection in 2D images.
In conclusion, MonoXiver improves methods already in use to extract 3D Information from 2D photos using bounding boxes. The old bounding boxes frequently failed to adequately represent every aspect of an object in a 2D image. Each bounding box serves as the beginning point for a subsequent examination of the region around it by MonoXiver, which results in several secondary boxes. Then, it evaluates the shape and design of each secondary box to see which best catches any object parts that are missing.
What Types of Data Annotation Needed in Conjunction With MonoXiver?
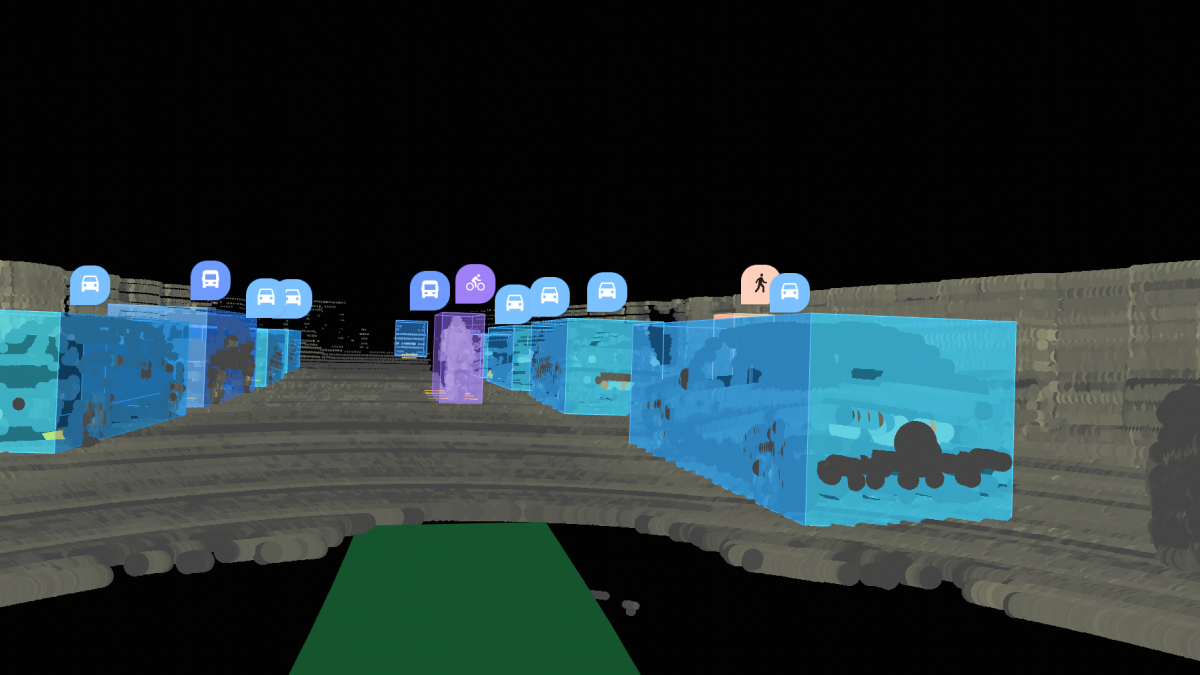
The biggest data annotation method we talked about in this article is the bounding box, which can be 2D or 3D. Bounding box annotation is used to train AI systems to detect various objects in a real-world scenario. The objects are detected in images and videos with high accuracy by using bounding boxes. It is also important to note that bounding boxes are important in 3D Point Cloud annotation as well.
In comparison to 2D bounding boxes, 3D bounding box annotation provides Information on the height, depth, and length of the objects in the photographs, increasing the accuracy of image classification. Additionally, compared to 2D Bounding boxes, 3D Bounding boxes provide more clearly defined boundaries for the objects in the photos.
Although there are many uses for the bounding box approach, object detection is its most crucial use. Thanks to the settings made when annotating the boxes on the set of photos presented, computer vision algorithms can instantly determine that these elements appear exactly like the references it was given previously. The altered colors of those objects will subsequently be displayed on the screen.
Trust Mindy Support With All of Your Data Annotation Needs
Mindy Support is a global provider of data annotation services and is trusted by Fortune 500 and GAFAM companies. With more than ten years of experience under our belt and offices and representatives in Cyprus, Poland, Romania, The Netherlands, India, OAE, and Ukraine, Mindy Support’s team now stands strong with 2000+ professionals helping companies with their most advanced data annotation challenges.