New and Improved LiDAR Technology Could Enhance the Safety of Autonomous Vehicles
Category: AI Insights
Published date: 02.03.2023
Read time: 6 min
LiDAR is one of the most important technologies that allow autonomous vehicles to navigate the road. However, there are certain objects that do not accurately reflect the pulses of light sent out by the LiDAR, making it difficult to get an understanding of just how far away the object really is. In this article, we will talk about how LiDARs work, what new advancements in LiDAR have to offer, and the data annotation required to train AI with LiDARs.
How Do LiDARs Work?
LiDARs send out pulses of light that bounce off objects and return back to the LiDAR. The faster the light returns, the closer the object is to the LiDAR and, conversely, the longer it takes, the farther away the object is located. This creates a 3D Point Cloud, which is a digital representation of how the AI system sees the physical world. In addition to the development of autonomous vehicles, 3D Point Cloud is widely used for product development and analysis in fields related to architecture, aerospace, traffic, medical equipment, regular consumer items, and more. The potential use cases and applications are only expected to increase in the future.
What are the Latest Advancements in LiDAR Technology?
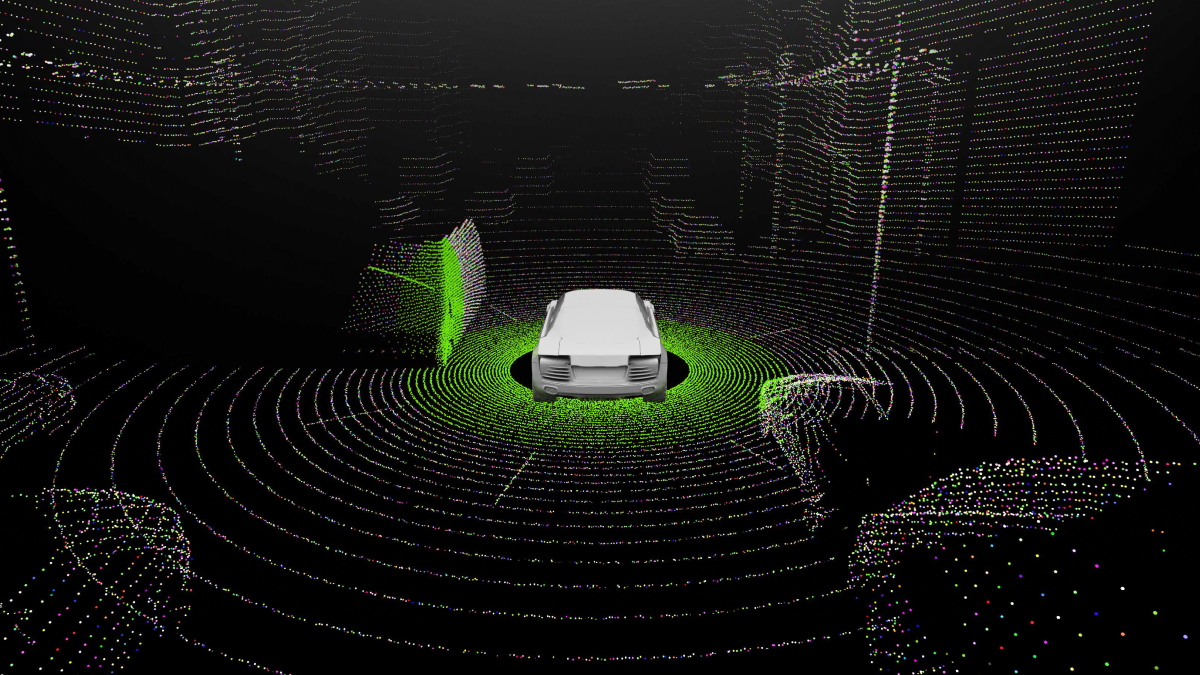
As mentioned in the previous sections, for the LiDAR to determine the proximity of an object, the light needs to bounce off a surface and return back. However, not all surfaces properly reflect the light. However, signals, such as point clouds captured by light detection and ranging sensors, are often affected by highly reflective objects, including specular opaque and transparent materials, such as glass, mirrors, and polished metal, which produce reflection artifacts, thereby degrading the performance of associated computer vision techniques.
A team of investigators from Kyoto University in Japan developed a new non-mechanical 3D LiDAR system that is capable of measuring poorly reflective objects and tracking their motion. This means that the vehicles will be able to reliably navigate dynamic environments without losing sight of poorly reflected objects. This new development is called a dually modulated photonic-crystal laser (DM-PCSEL). This advancement may eventually lead to the creation of an on-chip, all-solid-state 3D lidar system.
The DM-PCSEL integrates non-mechanical, electronically controlled beam scanning with flash illumination used in flash lidar to acquire a full 3D image with a single flash of light. This unique source allows researchers to achieve both flash and scanning illumination without any moving parts or bulky external optical elements, such as lenses and diffractive optical elements.
Measuring Distances of Poorly Reflected Objects
The DM-PCSEL-based 3D lidar system lets us range highly reflective and poorly reflective objects simultaneously. The lasers, 3D time of flight (ToF) camera, and all associated components required to operate the system were assembled in a compact manner, resulting in a total system footprint that is smaller than a business card. The researchers demonstrated the new system by using it to measure the distances of poorly reflective objects that were placed on a table in a lab. They were also able to demonstrate that the system could automatically recognize poorly reflective objects and track their movement through selective illumination. The next step would be to demonstrate the system in practical applications like autonomous vehicles and robots as well.
What Types of Data Annotation are Necessary to Train AI With LiDARs?
Earlier on, we talked about how LiDARs produce 3D Point Clouds, but this raw data needs to be annotated. One of the most important annotation types is polyline annotation which allows the AI to see the road markings that delineate lanes. In addition to this, semantic segmentation will also be necessary, which involves classifying individual points of a 3D point cloud into pre-specified categories. Segmenting objects in 3D point clouds is not a trivial task. The point cloud data is usually noisy, sparse, and unorganized. Apart from that, the sampling density of points is uneven, and the surface shape can be arbitrary with no statistical distribution pattern in the data.
In addition to this, the 3D Point Cloud may need to be color coded to identify the proximity of objects to the LiDAR. For example, objects that are closer may be blue or violet since these colors have a short wavelength. Conversely, objects farther away may be colored red or orange since they have a long wavelength.
Trust Mindy Support With All of Your Data Annotation Needs
Mindy Support is a global data annotation and data preparation provider, trusted by Fortune 500 and GAFAM companies. With more than 10 years of experience under our belt and offices and representatives in Cyprus, Poland, Romania, The Netherlands, India, UAE, and Ukraine, Mindy Support’s team now stands strong with 2000+ professionals helping companies with their most advanced data annotation challenges.