New AI Vehicles Provide Feedback on Driving Decisions
Category: AI Insights
Published date: 26.09.2023
Read time: 6 min
One of the biggest reservations drivers have about riding in an autonomous vehicle is that oftentimes, the car can be unpredictable. It’s difficult to understand what the vehicle will do in any given situation. However, thanks to new developments in AI, the autonomous vehicle provides feedback to the passengers about why it took a particular action. In this article, we will talk about the AI technology needed to develop this technology and the data annotation needed to train it.
Providing Passengers With Feedback
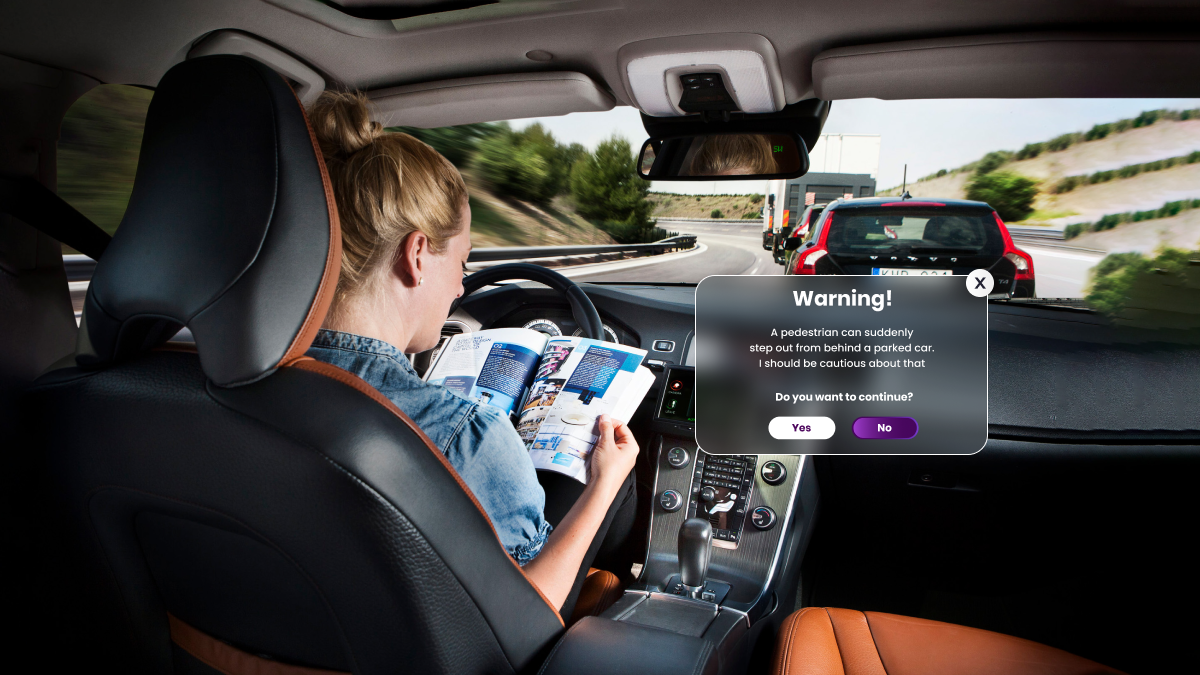
An artificial intelligence system for automated driving that provides feedback to its riders has been unveiled by a British tech start-up. The model can respond to inquiries that shed light on the motivations behind the choices it made and the actions it took. The system responded that a line of parked cars was a potential risk when questioned about the dangers of driving down a street in north London. When questioned further about why the parked cars were a hazard, the system responded, “Because there’s a chance that a pedestrian might slip out from between them, might step out unexpectedly. I should be cautious about that”.
Despite its seeming simplicity, the discussion between a human and a machine gets to the heart of one of the biggest worries about artificial intelligence (AI): the transparency of certain systems’ logic and the reasons behind what they do. According to many observers, establishing the public’s trust and allaying any concerns they may have regarding AI technologies depends on this transparency.
The developers of this technology think that by making autonomous driving better and safer, natural language has the potential to completely transform the industry. To make training AI models easier, it is possible to add natural language sources like national road user laws.
How Was This AI Model Trained?
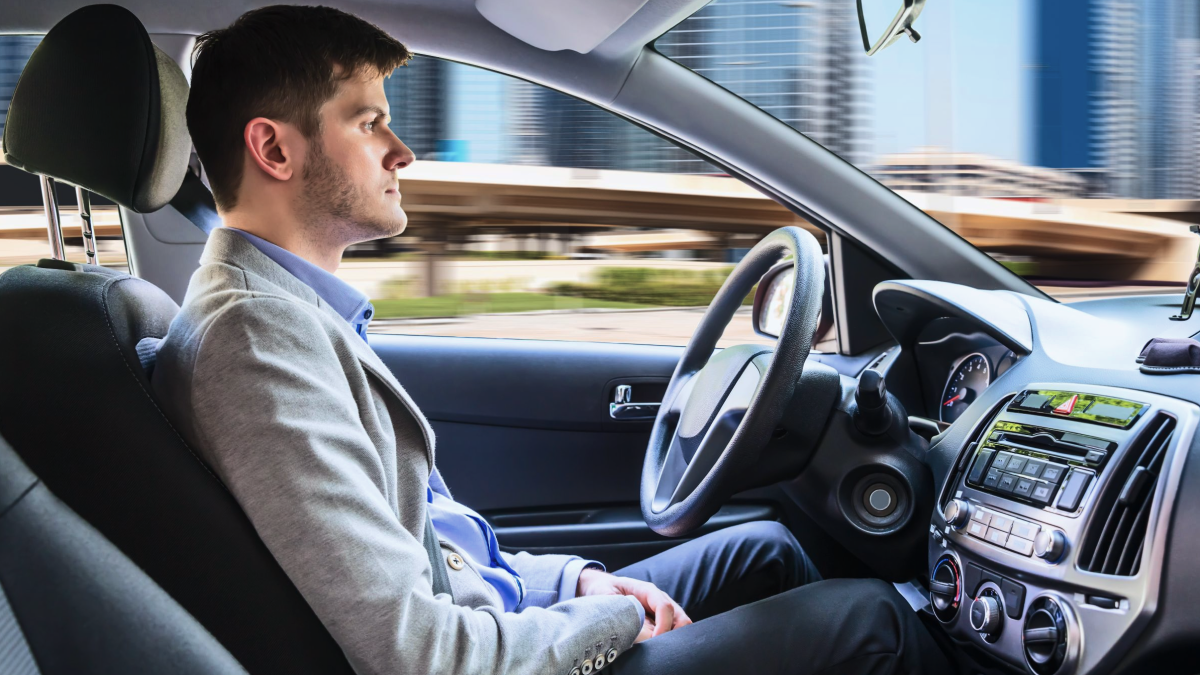
The AI vehicle can justify driving actions since it was taught using real-world data from drivers who commented while driving. This incorporation of language creates a new sort of data for understanding, elucidating, and training AI models. The car can answer inquiries concerning a wide range of driving scenarios in addition to providing commentary. Through the use of this capability, Wave is able to tweak the model in response to user comments. The responses provided allow engineers to assess the model’s knowledge of the situation and logic, which can assist the vehicle more effectively in identifying areas for development and boost system confidence.
This is what enables the vehicle to continuously deliver textual statements outlining the issue and defending choices, much like a driver speaking out loud or a driving teacher trying to maintain the learner’s focus. This textual logic could increase the sense of safety in cars by making their decisions seem less like a “black box.” It could also contribute to the safety of autonomous vehicles by allowing the system to reason textually through traffic scenarios that are not included in the training data.
The developing company is currently testing its self-driving technology on UK roads and is undertaking Europe’s largest last-mile autonomous grocery delivery trial with supermarket chain Asda in London. The company is confident that developments like this new AI car will help make the commercial deployment of self-driving vehicles a reality while building confidence in AI decision-making.
What Types of Data Annotation Needed to Create This Technology?
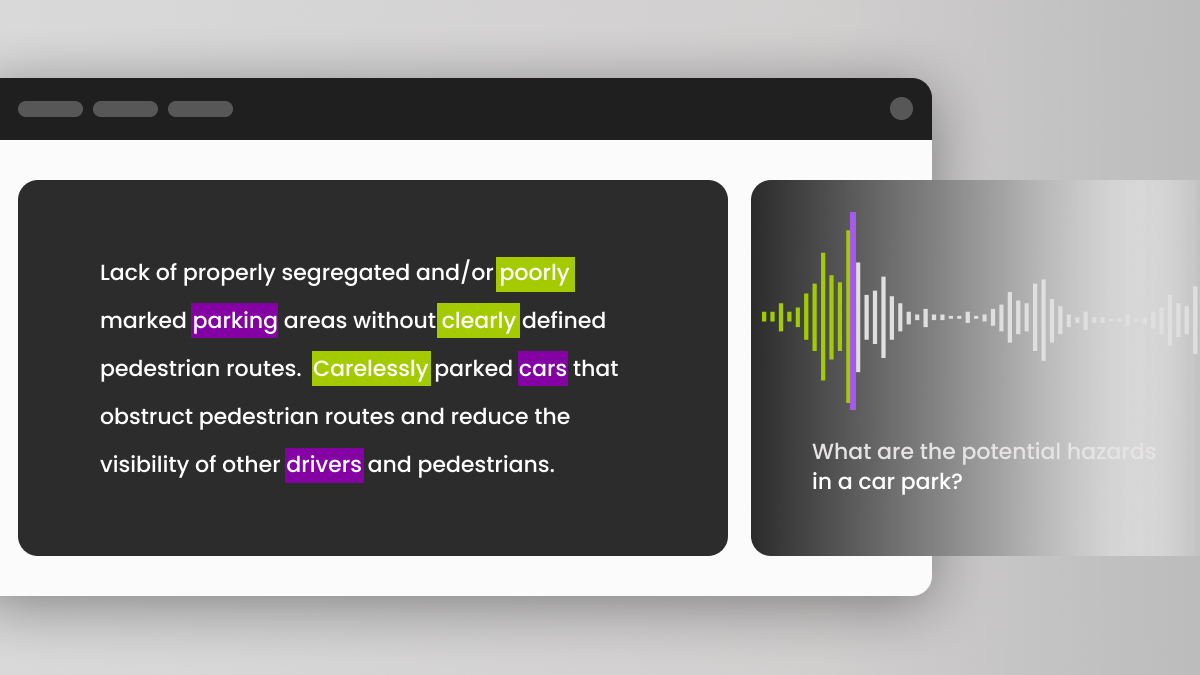
Since the AI vehicle needs to converse with human drivers, data annotation for natural language processing is needed. This includes techniques like entity annotation, which demonstrates to NLP models how to recognize named entities, keyphrases, and components of speech inside a text. Annotators in this work must carefully read the text, identify the target entities, highlight them on the annotation platform, and select a label from a specified list. Entity linking is frequently used in conjunction with entity annotation to aid NLP models in learning more about named entities.
Tagging various data in the audio recording will also be necessary since it helps the AI system identify and flag grammatical, semantic, or phonetic elements in the audio data. The data annotation work here could include things like linking anaphora and cataphora to their antecedents and subjects, annotation of all the various functions of words, tagging the intonation pauses, and other speech nuances.
Trust Mindy Support With All of Your Data Annotation Needs
Mindy Support is a global provider of data annotation services and is trusted by Fortune 500 and GAFAM companies. With more than ten years of experience under our belt and offices and representatives in Cyprus, Poland, Romania, The Netherlands, India, OAE, and Ukraine, Mindy Support’s team now stands strong with 2000+ professionals helping companies with their most advanced data annotation challenges.