LiDAR is Powering ADAS Technology
Category: AI Insights
Published date: 04.05.2023
Read time: 6 min
The day of reliable autonomous vehicles is soon arriving. If advanced driver-assistance systems (ADAS) are to offer comprehensive car safety solutions, they must deliver highly precise data more effectively for improved driver support. Light detection and ranging (LiDAR)-based ADAS systems, along with vision and radar-based sensors, provide a very safe driving experience by fully regulating the vehicle’s speed and steering.
In this article, we will take a closer look at the role LiDAR is playing in the development of future ADAS systems and the data annotation that is required to create them.
What is LiDAR?
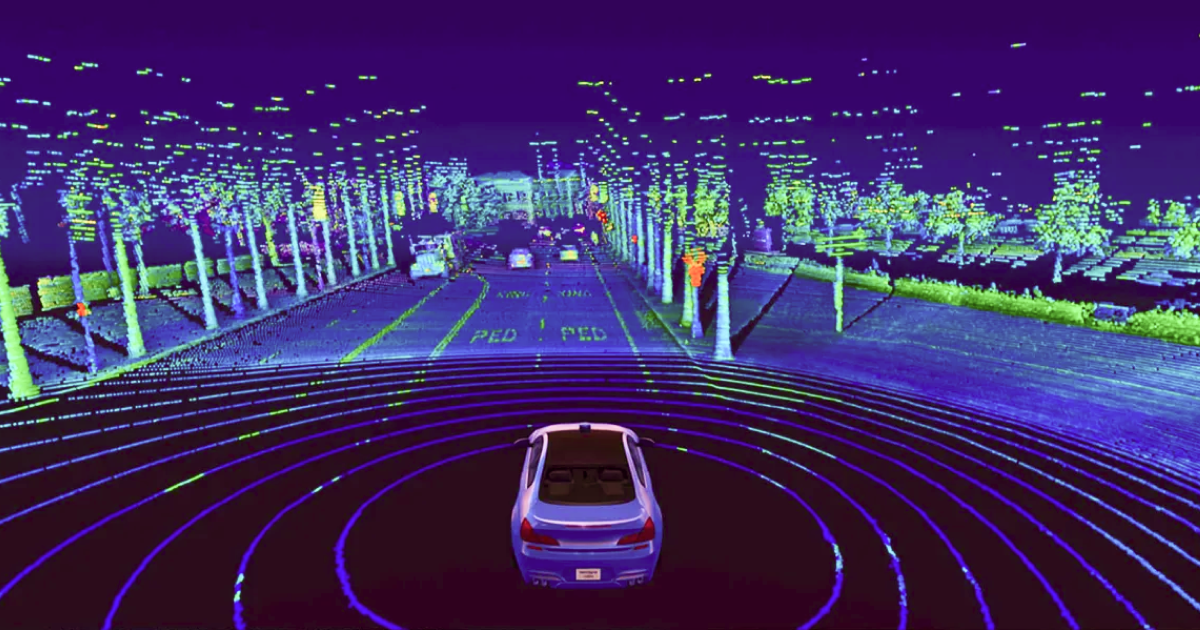
LiDAR, an optical technology, is frequently mentioned as a crucial technique for autonomous cars to sense distance. A lot of vendors are striving to create affordable, portable LiDAR systems. LiDAR is a crucial enabling technology that almost all manufacturers who are pursuing autonomous driving believe in, and certain LiDAR systems are now accessible for ADAS.
Instead of using radio electromagnetic waves, LiDAR implements the well-established and simple radar idea using a laser-based light beam. The target is illuminated by optical pulses in LiDAR systems, which then capture the reflected return signal and analyze it to get data on the pulse intensity, round-trip duration, phase shift, and pulse width. All of this results in the formation of a 3D Point Cloud, which is a vast array of minute individual points displayed in 3D space.
How Can LiDAR Improve ADAS Technology?
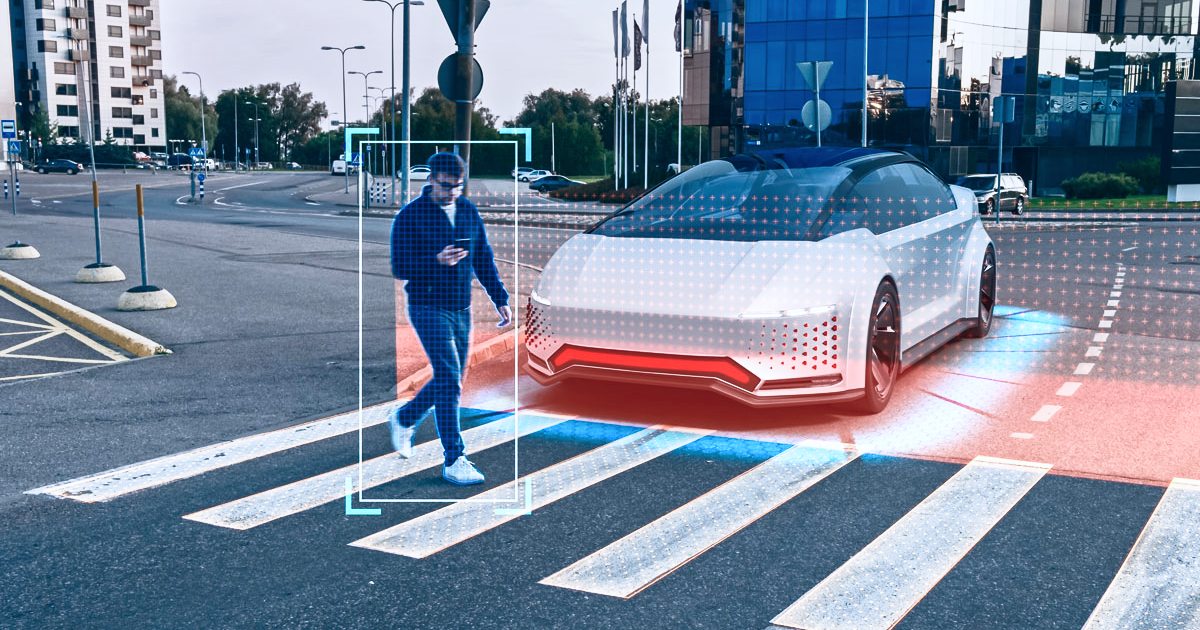
Autonomous vehicles are becoming more and more common, and self-driving vehicles may soon be a reality. A growing variety of ADAS functions, such as lane-keeping, adaptive cruise control, and structures for identifying blind spots during overtaking, are now possible thanks to high-performance sensors.
ADAS is both a helpful tool for drivers and a response to the demand for higher safety standards. As it can be used in adaptive cruise control, pedestrian detection, blind-spot detection, and all other use cases that call for the detection and mapping of objects around the vehicle, LiDAR is one of the most crucial parts of ADAS.
Most cars now come equipped with ADAS, which is Level 2 of the driving automation scale. Autonomous or semi-autonomous cars need sensors that can give a high level of safety. This means that the sensor must be reliable in all weather conditions and unaffected by elements like sun, rain, or fog for automobile applications. LiDAR sensors can be used in high-vibration transport systems, including driverless cars, construction, mining, and agriculture.
What are Some Examples of LiDAR in ADAS?
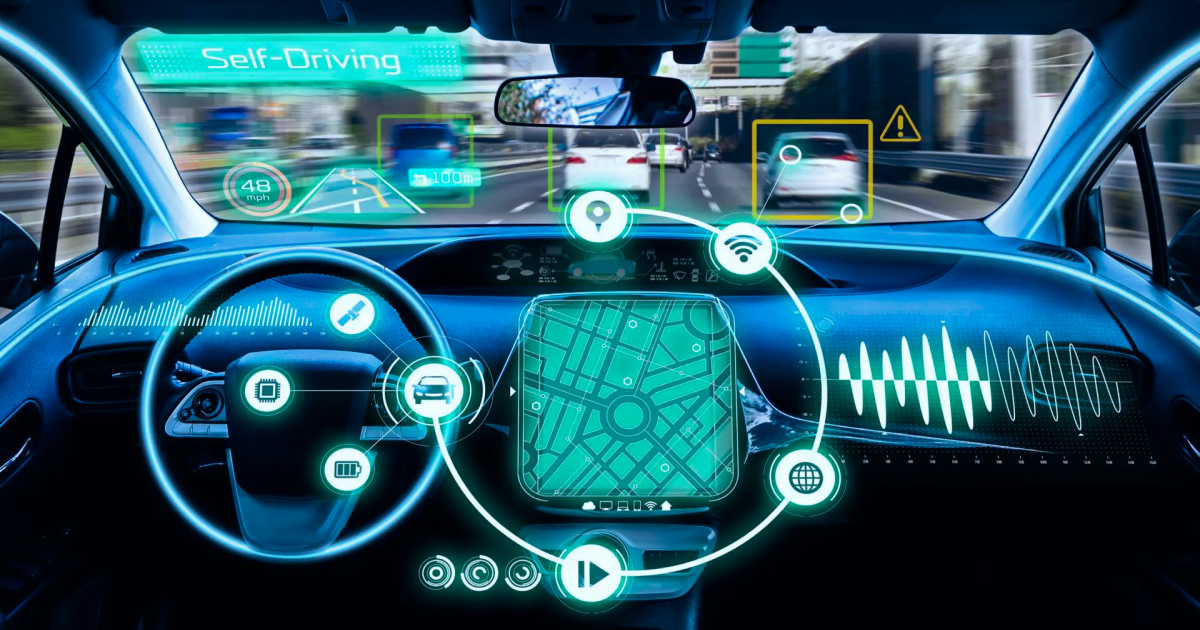
There are many examples of practical applications of LiDAR in ADAS, such as:
- Adaptive cruise control – Since LiDAR sends out pulses of light that bounce off other cars and return back to the LiDAR, it can allow the car to better navigate the road, especially during inclement weather conditions.
- Backup cameras – LiDAR can better detect the proximity of your car to another one since it actually measures the distance between the two objects based on how long it takes the light to travel.
- Blind spot monitoring – the pulses of light that LiDAR sends out can detect objects that are in the driver’s blind spot, and it can cover a broader area than cameras mounted on the cars.
What Types of Data Annotation are Required to Prepare LiDAR Datasets?
As mentioned earlier, LiDAR produces a 3D Point Cloud, which needs to be annotated with methods like 3D bounding boxes where a cube will be drawn around vehicles, pedestrians, traffic signs, and anything else useful in an image. Objects that do not fit neatly into a 3D box will have to be contoured with a polygon. Polyline annotation will also be necessary since this is what allows the AI system to learn about the delineation markings on the road.
Finally, more advanced types of annotation may also be necessary, depending on the specifications of the project. This includes semantic segmentation, which is where an AI model can learn how to identify individual objects and classes within an image by manually labeling each pixel. It helps an AI model to recognize objects and better understand the context of an image.
Trust Mindy Support With All of Your Data Annotation Needs
Mindy Support is a global provider of data annotation services and is trusted by Fortune 500 and GAFAM companies. With more than ten years of experience under our belt and offices and representatives in Cyprus, Poland, Romania, The Netherlands, India, OAE, and Ukraine, Mindy Support’s team now stands strong with 2000+ professionals helping companies with their most advanced data annotation challenges.