Leveraging AI During the Vehicle Manufacturing Process
Today’s cars are increasingly complex to assemble. In fact, the average passenger car consists of about 30,000 parts that need to be put together to make the vehicle work. In addition to this, these parts are imported from various countries all over the world. As if this wasn’t complex enough, it is also difficult to find skilled workers and manage all of the dependencies between teams. All of these challenges could result in costly delays. This is where AI can come to the assistance of car manufacturers by helping bring clarity to complex interactions and automate many processes to prevent failure down the road. Today we will talk about how automotive OEMs leverage AI in the vehicle manufacturing process.
Preventing Injuries
Toyota recently decided to implement new AI technology in its North American factories that could improve automation in production lines, thereby improving operating conditions and making conditions safer for workers. This will allow Toyota to spot deficiencies in production that go beyond simple observation by managers. The new AI system will monitor all points of operation with computer vision cameras which will allow Toyota managers to analyze potential issues before they become actual problems.
In addition to this, each workstation at Toyota North American factories will be equipped with self-contained intelligent devices that include a camera and processor, which will collect information on worker motions that could be detrimental to their health over the long term. This is done by tracking 17 specific joint movements and spotting physically demanding tasks that could cause injuries. If the worker is incorrectly performing certain motions or activities, it will detect this as well. The management team will be able to obtain reports in real time where improvements or adjustments are needed, which will allow the company to improve efficiency while keeping the workers safe.
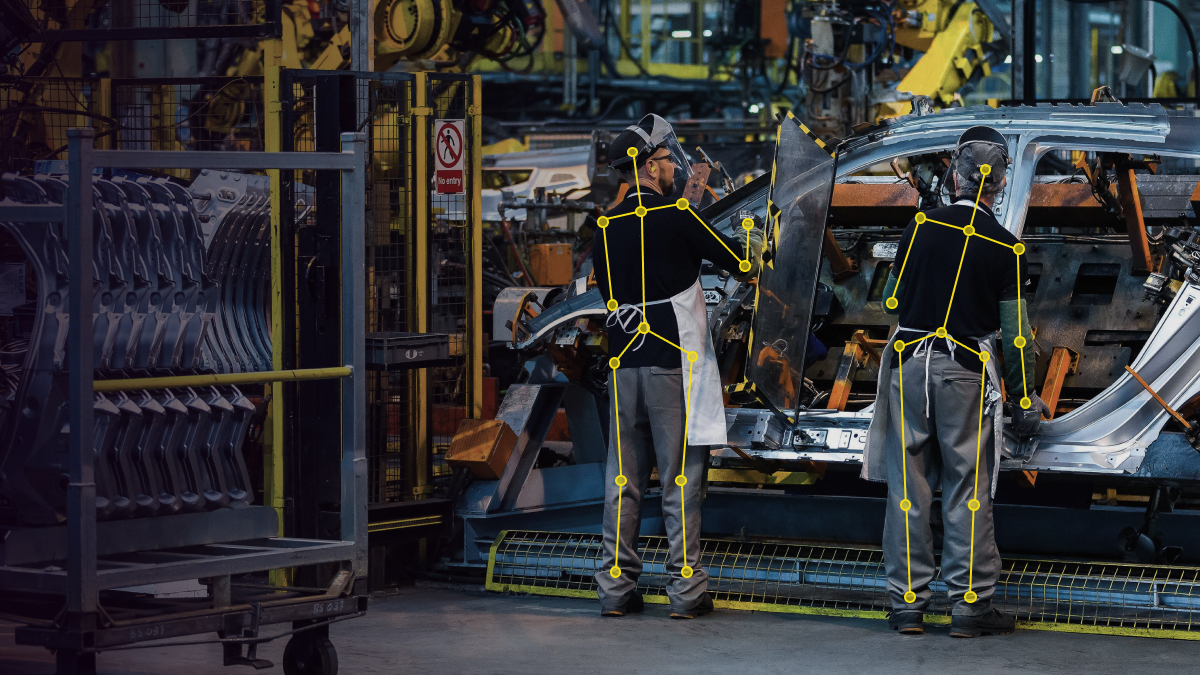
Implementing the Necessary QA Processes
One of the major benefits of AI is that not only can it analyze large volumes of data but also learn and make conclusions. This capability can be used to find possible weak points in production, improve the QA process of car bodies and also monitor potential machine overloads for potential failures. This allows car manufacturers to prevent defective cars from reaching consumers and avoid production downtime. A great example of this is Audi using AI to find even the smallest cracks in sheet metal within seconds.
Audi produces cars with sophisticated designs, and they inspect all of the components right after production. This includes a visual inspection by employees but also by cameras installed right on the presses. They evaluate the captured images with the help of image-recognition software. This process will soon be replaced by an ML procedure. Software based on a complex artificial neural network operates in the background of this innovative procedure. The software detects the finest cracks in sheet metal with the utmost precision and reliably marks the spot.
Making the Manufacturing Process More Efficient
For years, companies in the automotive industry have been trying to find ways to enhance work on the production line and increase efficiency in areas where people would get tired easily or be exposed to danger. Industrial robots have been present in car factories for a long time, but only artificial intelligence has allowed us to introduce a new generation of devices and their work in direct contact with people. AI-controlled co-bots move materials, perform tests, and package products, making production much more effective.
What Types of Data Annotation are Necessary to Create Such AI Technology
The data annotation methods will ultimately depend on the tasks the AI system will need to perform. For example, if we look at the AI developed by Audi, which is able to recognize even the tiniest scratches, semantic segmentation would be necessary to train such an AI system. The reason for this is that semantic segmentation is one of the most detailed types of data annotation. This is where data annotators analyze images of various scratches and label each pixel of an image with a corresponding class. The reason such a level of detail is needed is that the scratches can come in many different forms and can be very difficult to spot. Therefore, after the data process is complete, it is a good idea to perform a comprehensive QA analysis of the work done.
The technology used by Toyota tracks worker movements to enhance safety. Basically, here data annotators would look at videos and annotate every frame with key points that can be used in combination to form a point map that defines the pose of an object. The AI tool will connect all of the key points and perform pose estimation to determine if the performed action is dangerous or not. It is worth mentioning that such keypoint annotation can be used to train a machine learning model to mimic human pose and then extrapolate their functionality for task-specific applications, for example, AI-enabled robots.
Trust Mindy Support With All of Your Data Annotation Needs
Mindy Support is a global company for data annotation and business process outsourcing, trusted by several Fortune 500 and GAFAM companies, as well as innovative startups. With nine years of experience under our belt and offices and representatives in Cyprus, Poland, Romania, The Netherlands, India, and Ukraine, Mindy Support’s team now stands strong with 2000+ professionals helping companies with their most advanced data annotation challenges.