How Tesla Mastered Data Annotation to Be a Leader in the Autonomous Vehicle Market
Last week, Tesla unveiled some pretty cool new innovations at their annual AI day. There was a new computer chip that was built and designed in-house, to run and train its supercomputer, called Dojo. We also saw an interesting new human-like robot and some new ways of solving computer vision problems. What did not get as much attention is the data annotation and data generation Tesla uses to power their self-driving cars. Let’s take a closer look at the new things in data annotation and data generation so we can better appreciate them.
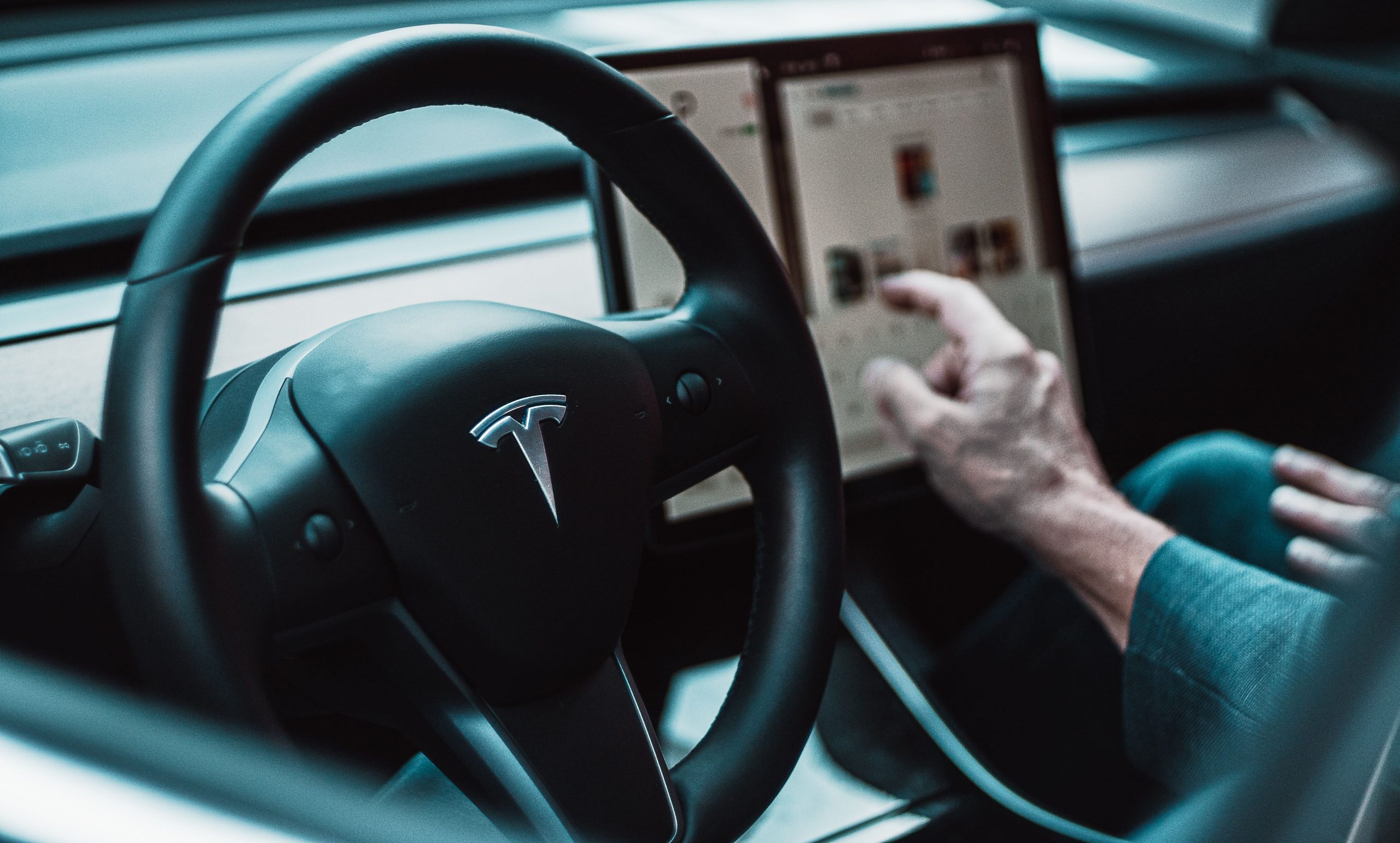
The Importance of Data Annotation
While Tesla’s autonomous vehicle technology is one of the most advanced in the world, even they were experiencing certain problems. To be more specific, they needed to solve the issue of temporary occlusions. For example, let’s say a Tesla approaches an intersection and its view of the street signs beyond the intersection is blocked by other cars. Another problem Tesla engineers were grappling with is remembering street signs and markers that appeared earlier in the trip. So, for example, if the Autopilot recognized a “Lane Ends in 100m” sign after it drove 100 meters, it had trouble “remembering” that it needed to merge to another lane.
To solve this problem, Tesla engineers used a spatial recurring network video module wherein different aspects of the module keep track of different aspects of the road and form a space-based and time-based queue, both of which create a cache of data that the model can refer back to when trying to make predictions about the road.
Ok, so, Tesla had a solution in mind, but it still needs to implement it and for this, they need a lot of data annotation. For this project, Tesla hired 1,000 data annotators who showed the audience how they annotate various images. To make all of the neural networks that predict in vector space all of the data annotations need to be done in vector space as well. This means having to create specific tools for the annotators to label in vector space and then project it out into the image space. This is very beneficial because you are annotating the data in the same space that you are making the predictions.
How Does Tesla Perform the Data Annotation Work?
Tesla is notoriously secretive about the way they create their products, but it was interesting that we got a glimpse of this at AI day. We learned that Tesla created special data annotation tools that allowed them to label in vector space significantly which shortens the amount of time it takes to annotate all of the data. To put this into perspective, Ashok Alluswamy, who leads the Planning & Controls Team at Tesla, said that the effort to remove radar dependency required 10,000 labeled video clips. Outsourced laborers would have taken several months to complete that task..
Where Does All of the Data Come From?
Before you can start annotating the data, you need to have a raw data training set already collected. Tesla accomplishes this through the Hydranet system, which is a set of eight cameras that not only allow the vehicle to “see” the road, but also collect all kinds of data about situations the car encounters. This data is used to generate highly data-dense maps showing everything from the average increase in traffic speed over a stretch of road to the location of hazards that cause drivers to take action.
On average a Tesla car generates two to five terabytes of data every week. While annotating the varieties of data generated at high velocity can be a problem, we already talked about the solutions Tesla created. It is also worth pointing out that they use auto-labeling as well, which is an AI tool that can annotate images and videos without human intervention. This allows Tesla to replace human workers in performing basic labeling tasks and use human annotators only for edge cases.
Mindy Support Provides Comprehensive Data Annotation Service
While Tesla employs in-house data annotators, the vast majority of companies developing AI products choose to outsource such work to offshore companies, like Mindy Support. We are the largest data annotation company in Eastern Europe with more than 2,000 employees in six locations all over Ukraine and in other geographies globally. Our size and location allow us to source and recruit the needed number of candidates within a short time frame and we can scale your team without sacrificing the quality of the work provided. Contact us today to learn more about how we can help you.