How AI is Transforming Supply and Logistics
Category: AI Insights
Published date: 09.12.2020
Read time: 6 min
Companies across various industries are starting to incorporate more AI technology into their everyday work processes and the logistics and supply chain industries are really capitalizing on all of the efficiency, automation, and cost reduction it offers. Given all of the benefits AI offers, it should come as no surprise that AI in the supply chain and logistics market will grow at a CAGR of 45.3% from 2019 to 2027 to reach $21.8 billion by 2027. Since the application of AI in supply chain and logistics is growing so rapidly, let’s take a look at some of the ways companies are using this technology to uncover the value it offers.
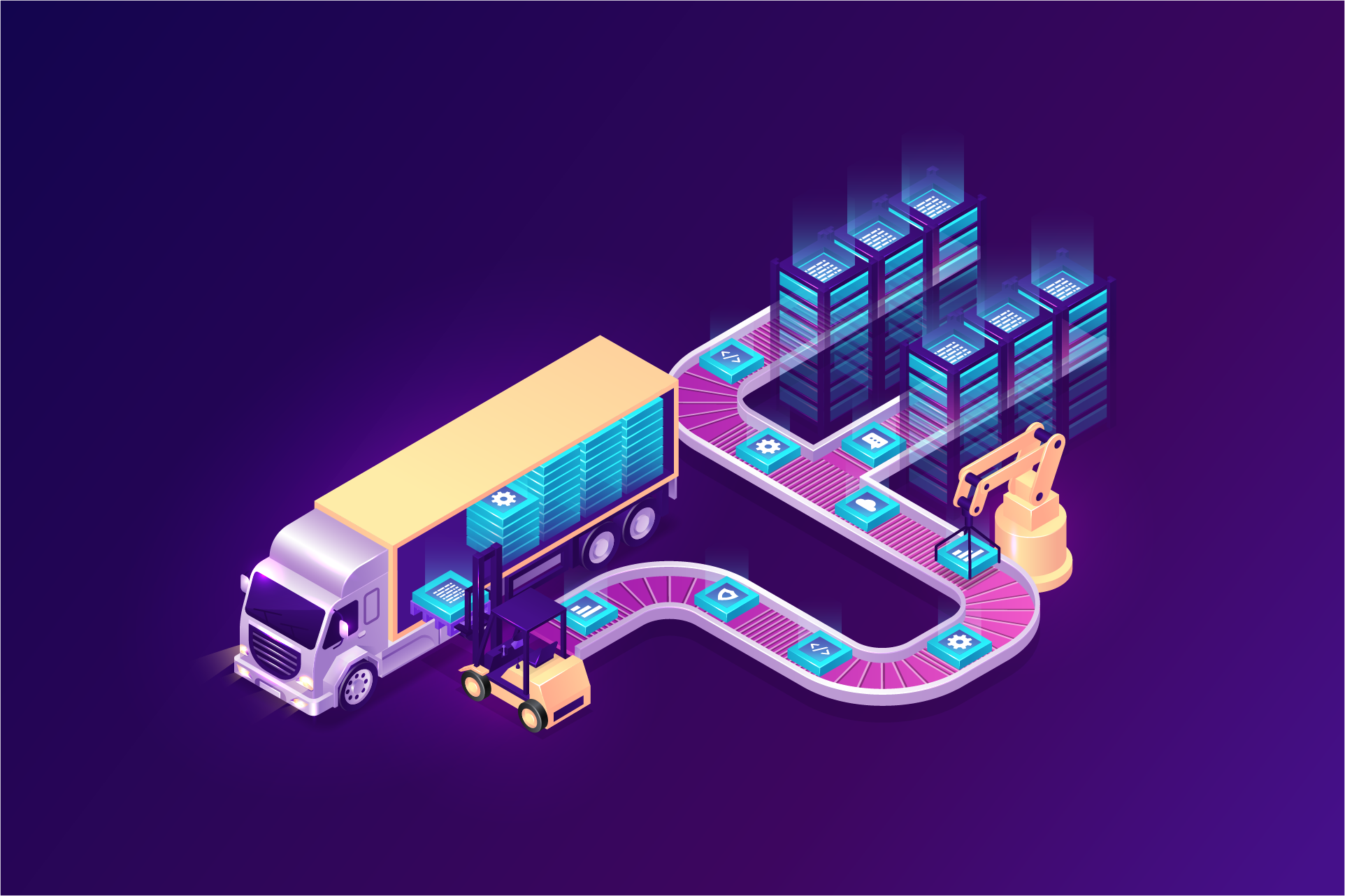
Using Robots to Enhance Service Operations
A typical warehouse will contain thousands and thousands of items while larger companies, like Amazon, will have millions of items on various distribution centers all over the world. Imagine how much time is wasted every year on simply walking up and down the aisles and taking an item off the shelf. If an employee doesn’t know where a particular item is and has to look around the warehouse to find it, then even more time is wasted. Such unnecessary motion is costing warehouses $4.3 billion every year or 265 million labor hours. Automated guided vehicles (AGV) can help reduce or even eliminate all of this wasted time by transporting items inside the warehouse, taking inventory, and many other tasks.
AGVs use computer vision to make their way around the warehouse and identify all of the items in their immediate environment. This is usually done by LiDAR technology which measures the time of flight of a pulse of light to be able to tell the distance between the sensor and an object. This creates a 3D point cloud which is a digital representation of how the robot sees the physical world. This 3D point cloud would need to be annotated by humans where they label each object in the image and even label the distances with various colors representing the wavelength. For example, the ground will always be blue on the 3D point cloud because it is the lowest point in the image and blue has a short wavelength.
Improving Warehouse Safety
Warehouses can be hazardous work environments given the number of people that work inside and all of the machinery they need to operate. In fact, one wrong move could lead to injuries and even fatalities. For example, operating forklifts is particularly hazardous and the Occupational Safety and Health Administration (OSHA) estimates that forklifts alone cause 101 deaths and nearly 95,000 workplace injuries each year. AI can assist forklift operators by providing them with an extra set of eyes since its sensors and cameras can warn the driver about other people or objects in their blind spots. In general, AI would provide workers with a lot more situational awareness, leading to improved safety conditions.
Such technology would also require highly detailed data annotation since the system would need to differentiate between all of the various objects and human beings. It would also need to have a sense of how much danger it poses and the proximity of the object or person to the forklift to reduce or even eliminate false positives. Human data annotators would have to label thousands and thousands of images ranging from simple 2D box annotation to the 3D point cloud annotation we mentioned earlier which can be very time-consuming, but the success of the project depends on having quality training data. This is why a lot of companies choose to outsource such work to a service provider.
Using AI to Improve Quality Control
A lot of processes in the supply chain have been impacted by AI and quality control is no exception. Computer vision cameras are enabling companies to achieve improved quality inspection at speeds, latency, and costs beyond the capabilities of human inspectors. When training the system to measure the quality control level, the first step would be deciding which features captured by the camera are relevant to the inspection. This can be things like edges, curves, corners, etc. Then the researchers would need to create a rule-based system detailing, for example, the acceptable curvature angle, or what a damaged edge on a box looks like. Once again, human data annotators would be responsible for annotating all of these various aspects in images for the system to learn from.
Mindy Support is Offering Comprehensive Data Annotation Services
All of the various technologies that we mentioned above require a lot of data annotation work to create quality training datasets. Performing such work in-house or in your local market can be very time-consuming and expensive, which is why it would be a lot easier to outsource such tasks to Mindy Support. We are one of the largest BPO providers in Eastern Europe with more than 2,000 employees in six locations all over Ukraine. We have a successful track record of actualizing data annotation projects of various sizes and complexities and we will be able to assemble a team for you within a short time-frame.