How AI is Transforming Agriculture
Category: AI Insights
Published date: 15.08.2023
Read time: 6 min
Agriculture has seen many changes and difficulties in recent decades, necessitating the sector’s modernization. A few of the issues pushing the digitization of agriculture include the rising global food demand, the depletion of natural resources, the need to minimize negative environmental effects, and international rivalry. This will allow farmers to maintain the needed levels of revenue while putting out the needed crop levels to feed the world population.
AI can assist farmers in their day-to-day lives of harvesting crops and making various processes more efficient. In this article, we will take a look at some of the ways AI is transforming the agriculture industry and the data annotation needed to train this technology.
Faster Detection of Pests and Diseases
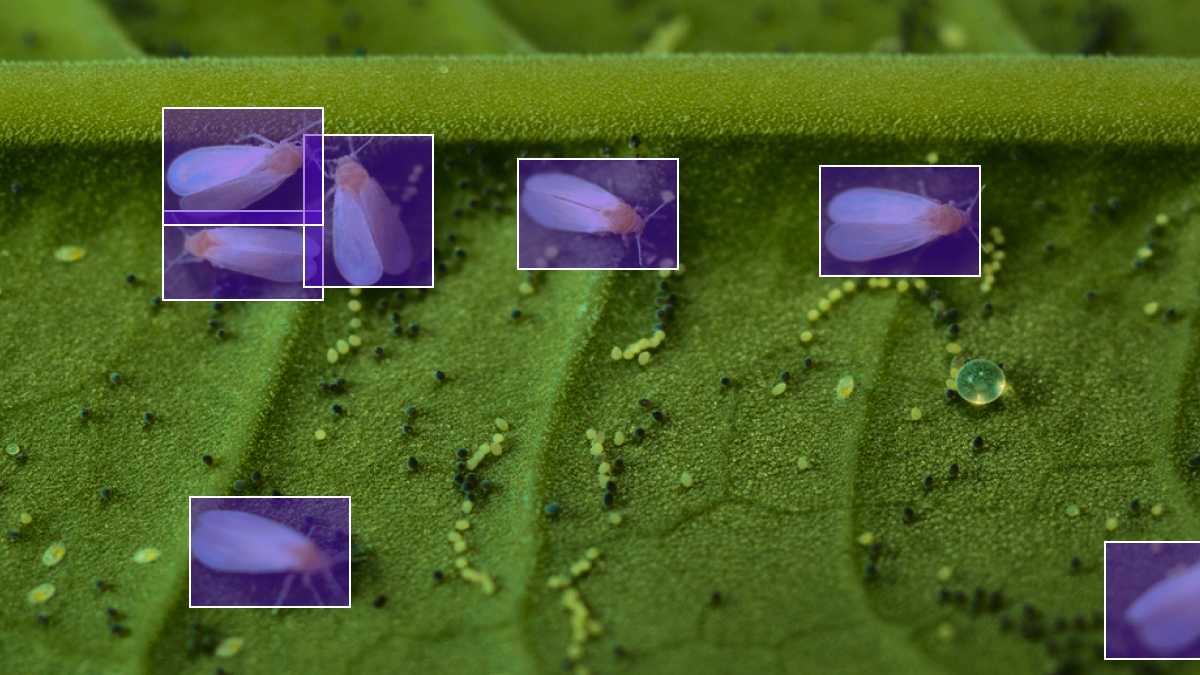
A higher level of yields and better fruit quality are made possible by the prompt diagnosis of pests and diseases. Rapid resolution of phytosanitary issues is essential. Uncontrolled pests or diseases harm crops, lowering their capacity for production and raising costs, which could jeopardize the success of an entire agricultural operation.
Satellites, drones, and software for field assessment monitoring are just a few examples of technologies that might help farmers quickly respond to pests and illnesses that could gravely jeopardize the output of their crops. You must be ready since a plague could spread in month two of the campaign and consume the majority of your budget. The easiest method to do this is via technology. In order to achieve the best-integrated pest management (IPM) control, growers may now more quickly reach problem areas and apply specific treatments to stop issues from spreading throughout their productive area.
Labor Optimization
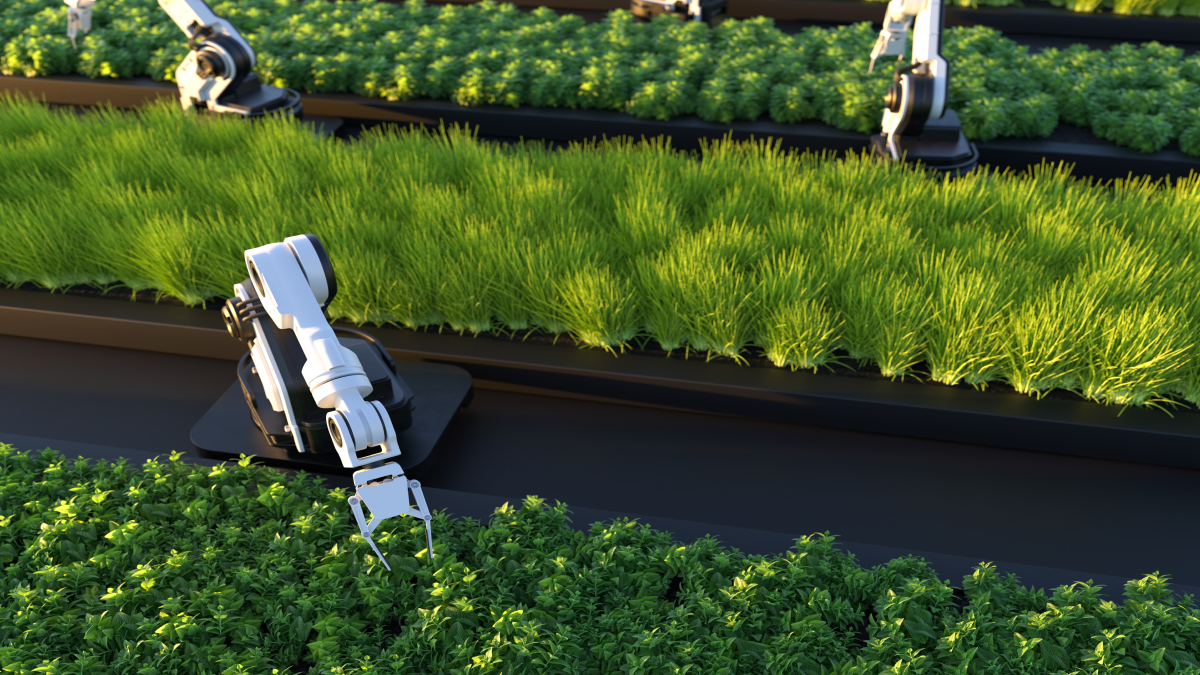
In recent years, robotic tools for agriculture have advanced significantly. These AI-based technologies are primarily used for weeding, tracking soil moisture and other field conditions, or employing remote-controlled tractors to plant fruits and vegetables. This means that human workers no longer have to be burdened with performing these mundane tasks and can focus on higher-level initiatives.
Teaching robots to perform these tasks requires modernized versions of both the fields and orchards and how the fruits and vegetables appear to the AI system. For example, let’s imagine an AI robot that can pick apples. Traditional orchards are extremely difficult for algorithms to analyze and process because of the unevenly shaped trees and enormous canopies. The difficulties of computer vision are exacerbated by shifting sunbeams, fog, and clouds. Even human fruit pickers encounter difficulties with tangled, tall, old trees; as a result, they end up spending the majority of their time moving ladders and not selecting fruit.
For this reason, many farmers have transitioned to orchards where trees grow flat against trellises, their trunks, and branches at right angles to create a “wall of fruit”. The emergence of robotic farmworkers could even be an opportunity for humans to engage in different – and far less strenuous – work than pruning or harvesting.
What Types of Data Annotation are Needed to Train These AI Robots?
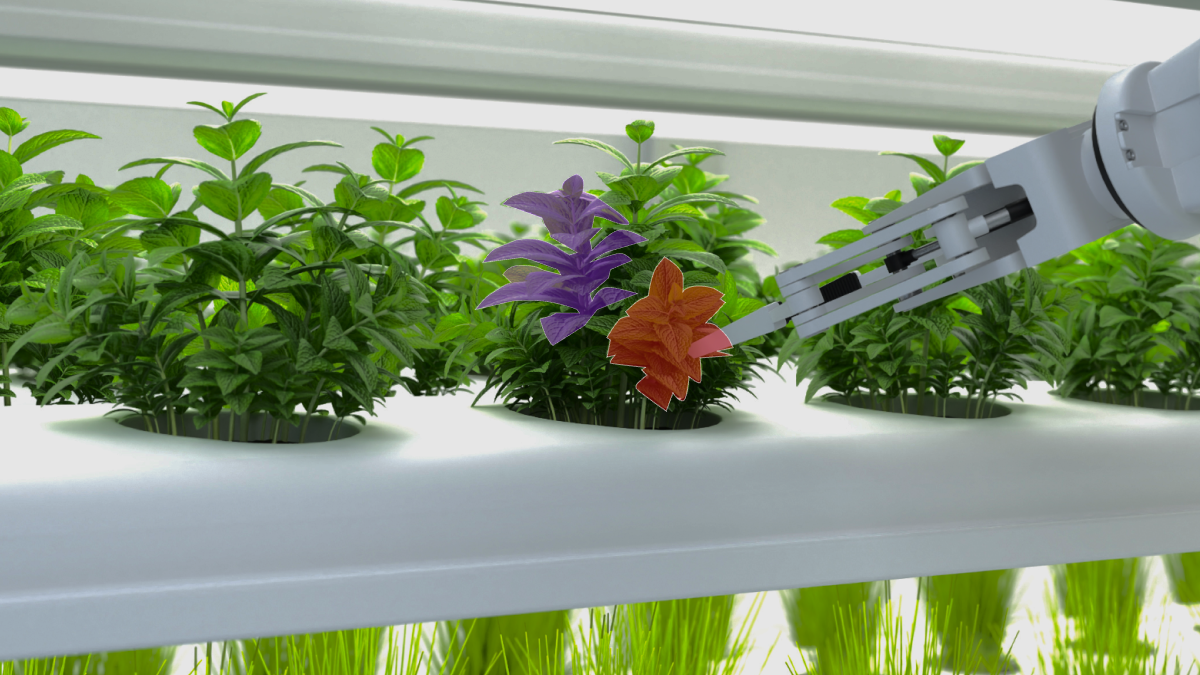
The AI technology used in drones to fly over farmlands and take aerial images relies on computer vision to identify things like healthy and unhealthy plants, drought areas, pests, and many other things. The data annotation techniques needed here could range from bounding boxes to more complex types, like image classification. The latter is where the models are trained to predict if a defined object will show in an image or video, but they are unable to determine where it will appear or how frequently. These models use classification or multi-classification labels that are applied to the entire image, signaling if the frame contains a specific class.
Robots that need to pick fruits and vegetables need more in-depth data annotation to not only determine one crop for another, but also to determine if the crop is ripe for picking. This means annotation types like object recognition will be needed, which is the accurate labeling of the existence, location, and number of one or more items in an image is the goal of this type of picture annotation. It can also be used to pinpoint a specific object. You may teach a machine learning model to recognize the items in unlabeled photographs on its own by repeating this technique with various images.
More detailed types of image annotation, like semantic segmentation, are also needed because this is what helps the AI system establish boundaries between related things and assign the same identity to all of them. When you wish to comprehend the existence, position, and, occasionally, the size and shape of items, you employ this strategy.
Trust Mindy Support With All of Your Data Annotation Needs
Mindy Support is a global provider of data annotation services and is trusted by Fortune 500 and GAFAM companies. With more than ten years of experience under our belt and offices and representatives in Cyprus, Poland, Romania, The Netherlands, India, OAE, and Ukraine, Mindy Support’s team now stands strong with 2000+ professionals helping companies with their most advanced data annotation challenges.