HD Mapping is Improving the Accuracy of Self-Driving Cars
Category: AI Insights
Published date: 10.05.2023
Read time: 6 min
HD mapping is flying under the radar even though it’s becoming an important part of autonomous vehicles’ infrastructure. In the same way, engines need infrastructure like gas or charging stations to operate at scale, and self-driving features require HD maps to precisely localize themselves and to navigate accurately on a lane-level basis. In this article, we will take a closer look at HD mapping, how it can be used in the automotive industry, and the data annotation required to make them useful for training self-driving vehicles.
What is HD Mapping?
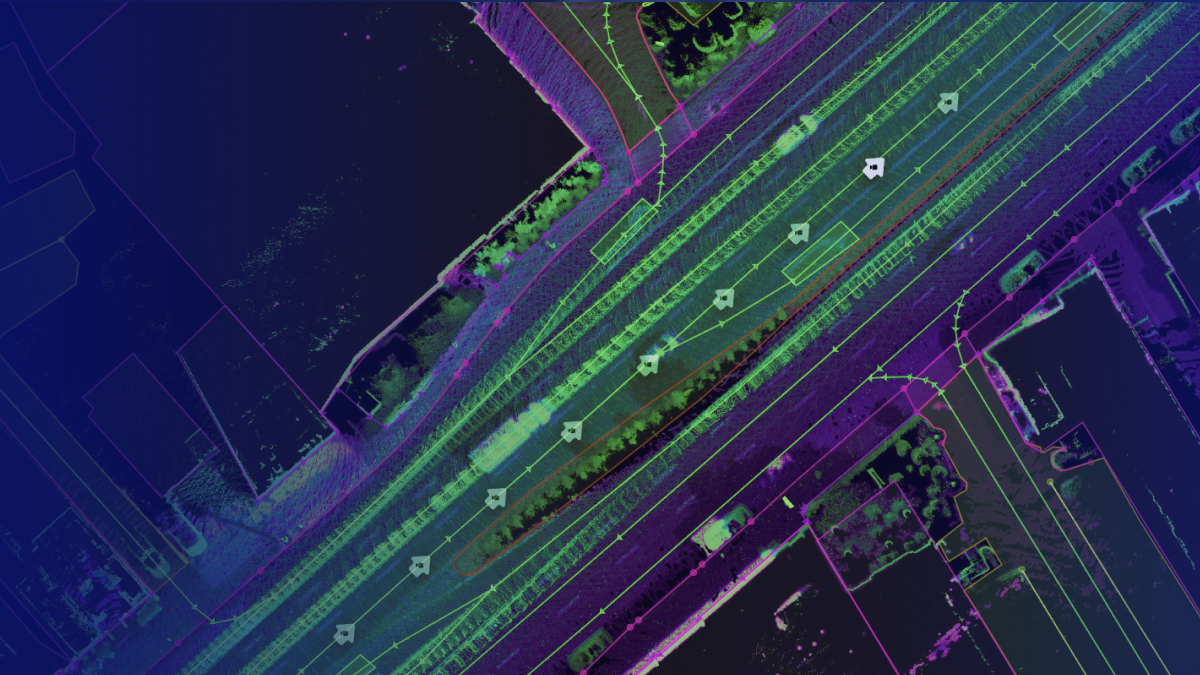
HD maps (high-definition maps, also called 3D maps) are roadmaps with inch-perfect accuracy and high environmental fidelity – they provide more precise information about the location of pedestrian crossings, traffic lights/signs, barriers, and more. While a human driver won’t crash because their map is a few meters off since we simply understand what the map refers to in the scene we see through the windshield. However, autonomous vehicles cannot compensate for map errors the same way humans can when using their GPS.
It can be beneficial to think of HD maps as the difference between knowing a route by heart and taking it for the first time. This helps to explain the choice between riding in a car with a seasoned driver or one who is utterly unfamiliar with the area.
How is HD Maps Created?
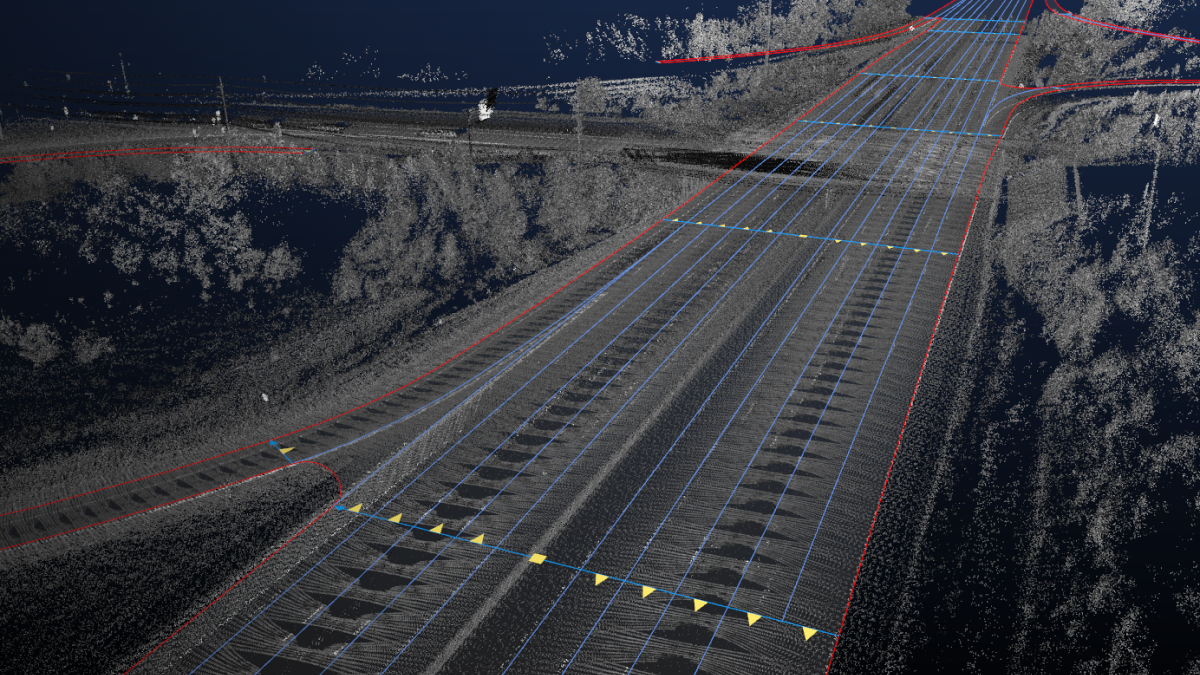
There are several ways of creating HD maps. One way is through LiDAR. Basically, the way this works is that companies working on HD Maps collect the data by driving LiDAR-mounted cars and generating point cloud datasets. Engineers, in a time-consuming process, review images and label the object. These labels can be Roads, lanes, traffic signals, and signs.
Another way is through aerial imagery. Every pixel in the millions of photographs that satellites take each year reveals useful information that AI and ML can use to recognize various index items for HD Maps. To fill in the information gap between well-known map features, like streets, and empty spaces, such as parking lots, high-resolution photography is used.
HD Maps vs. Traditional Maps: What’s the Difference?
HD maps are far more detailed than regular ones. Usually, they are displayed on a centimeter scale. HD maps provide data on lane placement, road boundaries, the severity of curves, the gradient of the road surface, and more, in addition to simply identifying the positions of certain locations or the distance between two destinations.
This level of information is necessary due to the wide disparity in road conditions. For instance, the width of bike lanes can vary depending on whether they are designated or not. Plus, in areas with very high traffic volumes, some municipal planners might decide to make such lanes significantly wider than the minimum.
If a self-driving car assumes that all bike lanes are the same width, the car could maneuver in a that puts the rider at risk. Traditional maps are meant to be read by humans. When continually analyzing their environment and making sure they don’t run off the road or divert into another lane, people might use factors like experience and depth perception. Autonomous vehicles require maps that complement their capabilities because they lack human-level skills. HD maps offer details that enable driverless cars to navigate from one place to another without making mistakes.
What Types of Data Annotation are Required to Prepare HD Maps to Train AI Systems?
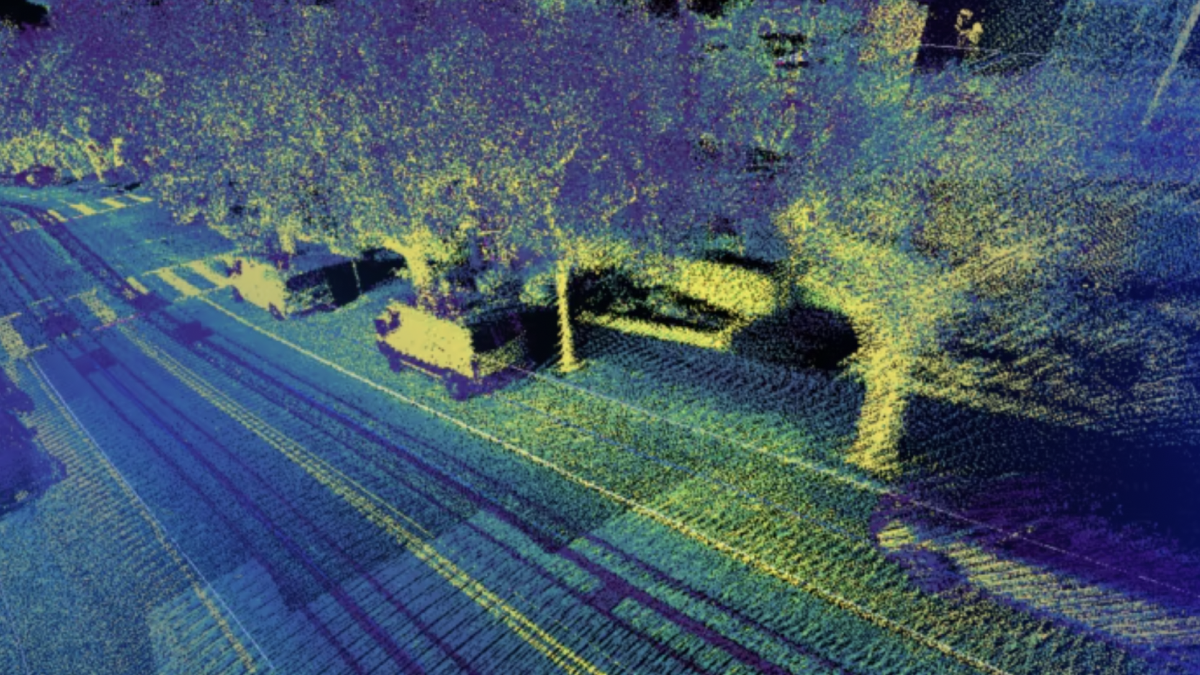
Earlier, we talked about how LiDAR is one of the ways of creating HD maps. LiDAR creates a 3D Point Cloud, which needs to be annotated with things like cuboids to capture objects in the image. For irregular or complex shapes, polygon annotation can be used since it captures more lines and more angles than a regular bounding box. More detailed types of annotation, such as polyline annotation, will also be necessary since this is what helps the vehicle identify all of the various road markings and curbs.
For aerial images, techniques like semantic segmentation will be used, which treats multiple objects of a particular category as one collective entity. Objects shown in an image are grouped together based on defined categories. In addition to this, instance segmentation can help provide greater granularity because it detects the instances of each category. It identifies individual objects within these categories. Categories like “vehicles” are split into “cars,” “motorcycles,” “buses,” and so on.
Trust Mindy Support With All of Your Data Annotation Needs
Mindy Support is a global provider of data annotation services and is trusted by Fortune 500 and GAFAM companies. With more than ten years of experience under our belt and offices and representatives in Cyprus, Poland, Romania, The Netherlands, India, OAE, and Ukraine, Mindy Support’s team now stands strong with 2000+ professionals helping companies with their most advanced data annotation challenges.