Exploring the Future of Banking With AI
Category: AI insights
Published date: 13.11.2023
Read time: 6 min
Generative AI is already being used by banks with the goal of increasing profits and enhancing decision-making and risk management. However, banks will need to handle more risks, worries, and expenses as a result of this. Banks with effective AI implementations may gain capabilities and efficiency that provide them a competitive edge and point of differentiation. In the long run, this could affect our perceptions of creditworthiness in three main ways: risk management, financial performance, and business franchise.
In this article, we will take a look at how banks can utilize AI and the data annotation required to create these products.
Fraud Detection
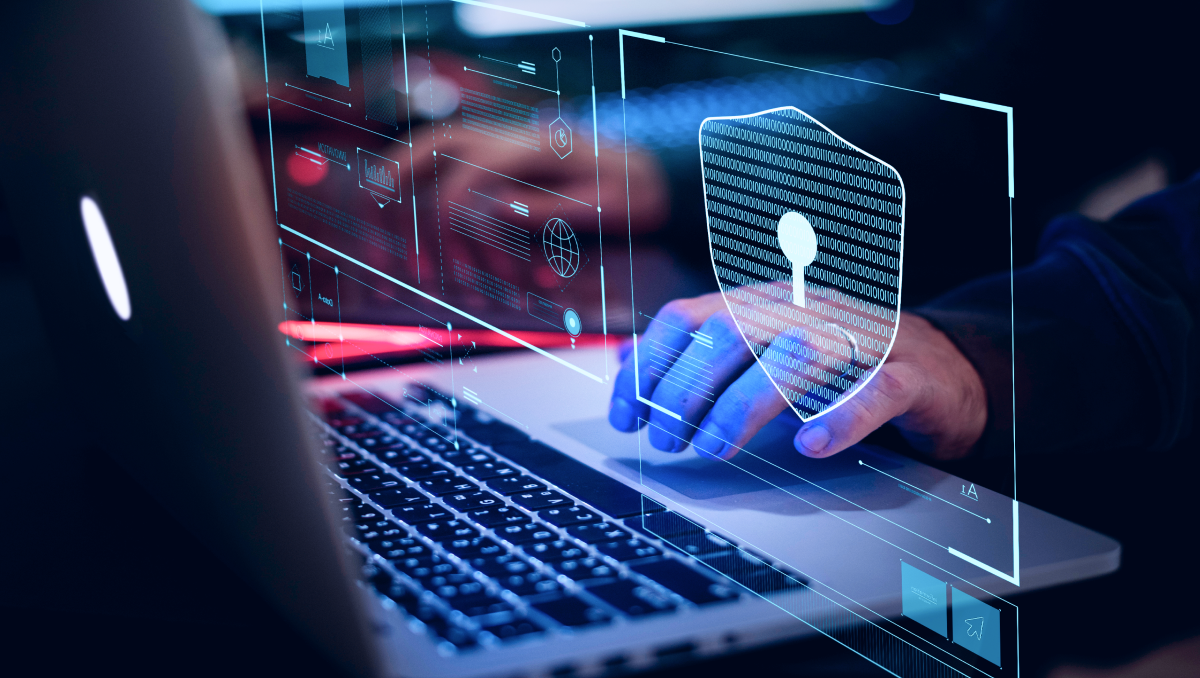
Conventional techniques for looking into financial fraud depend on human analysis and manual verification. Regretfully, these approaches frequently prove inadequate in addressing the ever-intricate and refined fraudulent schemes of today. Because of these drawbacks, it is essential to investigate how machine learning and artificial intelligence might revolutionize fraud investigations.
Investigators can examine relationships between things, spot suspicious trends, and visualize complex linkages by using AI algorithms and entity link analysis. Companies like Mastercard are already leveraging this technology to prevent criminals from scamming funds through a series of so-called mule accounts to disguise them. Mastercard has collaborated with UK banks for the previous five years to track the movement of money via these accounts and subsequently terminate them. With Mastercard’s AI solution, banks can intervene in real-time and stop a payment before funds are lost. This is done by overlaying specific analysis factors, such as account names, payment values, payer and payee history, and the payee’s links to accounts associated with scams, with the insights gained from this tracing activity.
Managing Risk
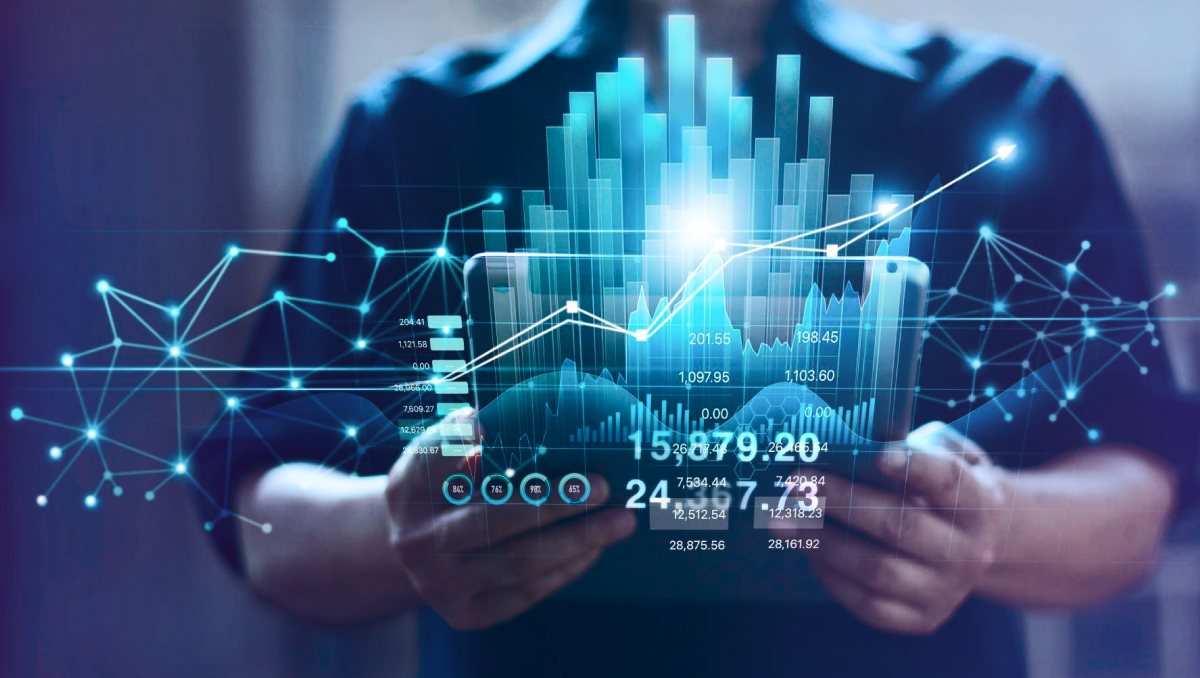
AI and ML are now synonymous with cost-effectively increasing productivity and efficiency in risk management. The capacity of the technologies to manage and analyze massive amounts of unstructured data at quicker rates with noticeably less human intervention has made this possible. In addition to lowering operational, regulatory, and compliance costs, technology has given banks and other financial institutions the ability to make correct credit decisions.
Since machine learning (ML) models are challenging to comprehend and difficult to verify for regulatory purposes, banks have historically used classical credit risk models to predict categorical, continuous, or binary outcome variables (default/non-default). However, they can still be applied to enhance the variable selection procedure and optimize parameters in current regulatory models. Despite having non-linear features, AI-based decision tree approaches can produce easily traceable and logical decision rules. While classification approaches like support vector machines can predict important credit risk features like PD or LGD for loans, unsupervised learning techniques can be utilized to examine the data for traditional credit risk modeling.
Streamlining the Credit Underwriting Process
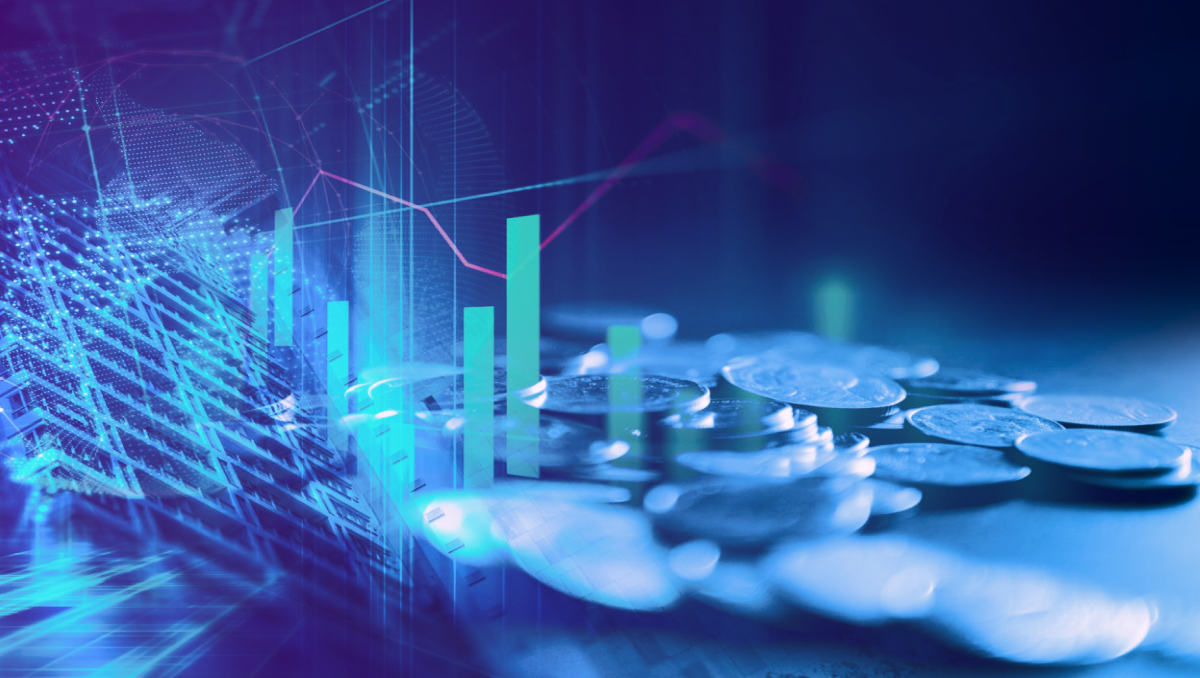
With larger and more complicated loan portfolios and a more difficult competitive landscape, underwriting has taken on a greater and greater significance. Underwriters have become more specialized in order to satisfy the demands of this dynamic market. They do this by using cutting-edge technologies and complex data analytics to increase the speed and precision with which they evaluate a potential buyer’s creditworthiness.
AI systems evaluate millions of data points from credit bureau sources to accurately forecast credit risk for any consumer, in contrast to traditional underwriting models that concentrate on a few credit criteria. It can significantly surpass standard scorecards in both consumer and small business financing by utilizing machine learning advancements. You may fully take advantage of artificial intelligence to improve your lending performance by developing models that take into account factors more than basic default and putting an emphasis on things like profitability and client lifetime value.
What Types of Data Annotation are necessary to Train These Technologies?
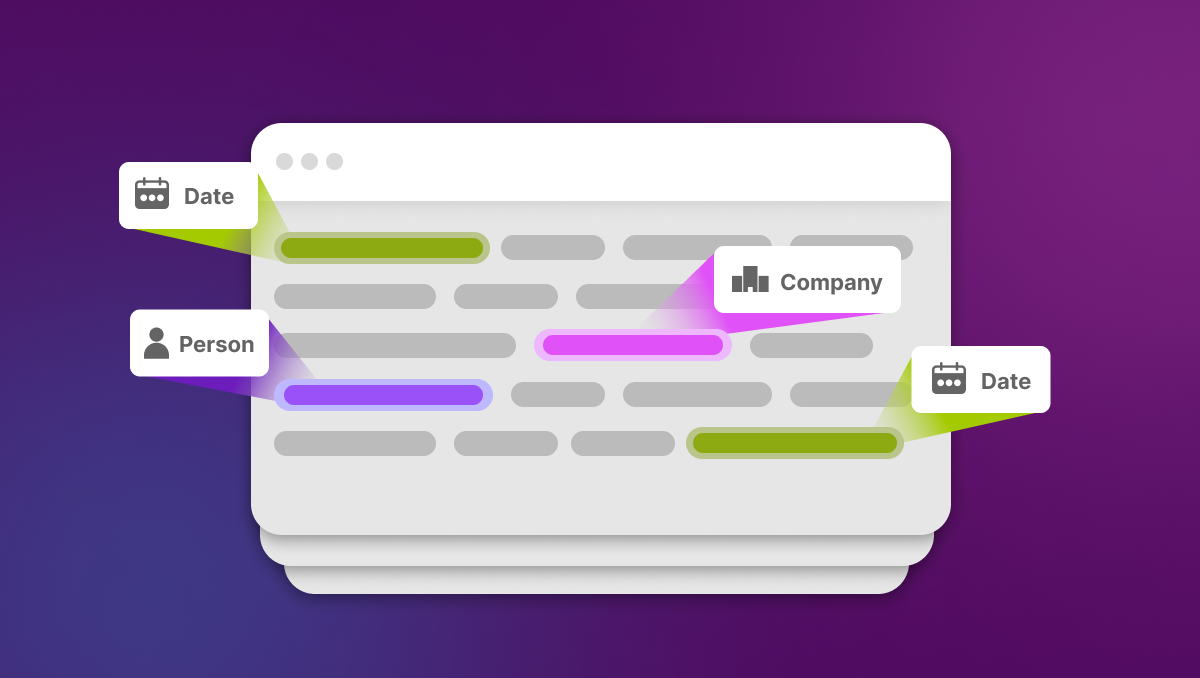
One of the main data annotation techniques used to train machine learning models is entity recognition and linking. This Is the process of locating, extracting, and tagging entities in text. AI models can recognize named entities, keyphrases, and elements of speech in texts by using entity annotation. Annotators must carefully read the text, identify the target entities, highlight them on the annotation platform, and select a label from a predefined list in order to complete this work. Entity annotation is frequently used in conjunction with entity linking to aid AI models in their further learning of named entities.
Consequently, the process of connecting those entities to more extensive stores of data about them is known as entity linking. Entity linking enhances user experience and search functionality. Linking labeled entities in a text to a URL containing more information about the entity is the responsibility assigned to annotators.
Trust Mindy Support With All of Your Data Annotation Needs
Mindy Support is a global provider of data annotation services and is trusted by Fortune 500 and GAFAM companies. With more than ten years of experience under our belt and offices and representatives in Cyprus, Poland, Romania, The Netherlands, India, OAE, and Ukraine, Mindy Support’s team now stands strong with 2000+ professionals helping companies with their most advanced data annotation challenges.