Creating New Firefighting Tools With AI and 3D Point Cloud Annotation
Category: AI Insights
Published date: 06.09.2022
Read time: 6 min
One of the most dangerous aspects of the job of a firefighter is that there are dangers lurking around the corner that they cannot see. When they enter a burning building and look for survivors, there is so much information firefighters need to process about a scene that potential dangers become an afterthought. One such danger is a phenomenon called flashover.
In this article, we will talk about how AI is helping firefighters battle flashover, as well as the data annotation required to create such technologies. First, let’s get an understanding of what flashover is.
What is Flashover?
In a burning building, there are many flammable objects, such as furniture, electrical equipment, household items, and many other things. The flashover phenomenon occurs when almost all of these flammable objects ignite all of a sudden and is a leading cause of death for many firefighters. Thanks to new developments in AI, firefighters can be warned about objects that are about to ignite. Researchers at the National Institute of Standards and Technology at the Hong Kong Polytechnic University have created a Flashover Prediction Neural Network that can predict deadly events and give firefighters valuable time and information to help save their lives.
The system secured an accuracy of over 92% across more than a dozen common residential floor plans in the US and came out leading while competing with other AI-driven flashover predicting programs. To predict these events, existing tools generally relied on either constant streams of temperatures or leverage ML to fill in the missing data in events when heat detectors fail to perform under high temperatures. But this AI system is advanced and does not succumb to any external factors.
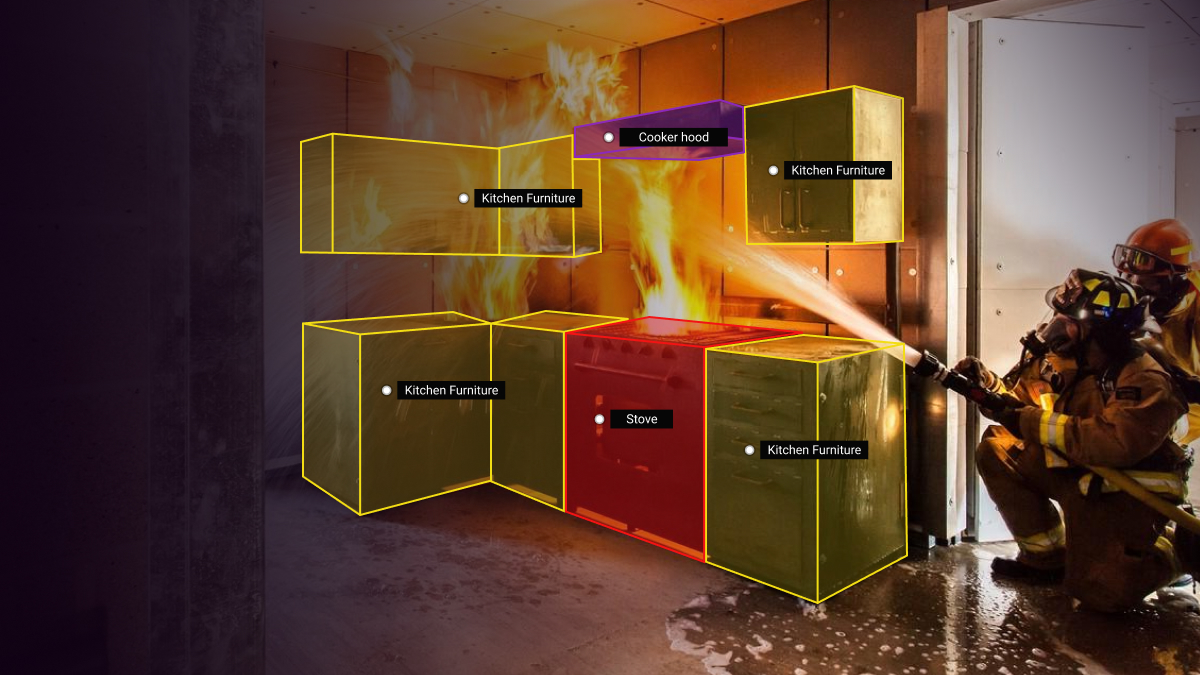
How Do Researchers Train Such AI Technology?
Training machine learning models of this nature requires a lot of test data and conducting lots of large-scale fire tests, which was just not feasible. This is why the team used virtual models of houses that they “burned” using the university’s Consolidated Model of Fire and Smoke Transport (CFAST), a fire modeling program validated by real fire experiments. Data from the simulation was fed into the algorithm, which included data from a three-bedroom, one-story ranch-style home since this is the most common type of home in most states.
In total, 5,041 simulations, with slight but critical variations between each. Different pieces of furniture throughout the house ignited with every run. Windows and bedroom doors were randomly configured to be open or closed. And the front door, which always started closed, opened up at some point to represent evacuating occupants. Heat detectors placed in the rooms produced temperature data until they were inevitably disabled by the intense heat.
To learn about the system’s ability to predict flashovers after heat detectors fail, the researchers split up the simulated temperature recordings, allowing the algorithm to learn from a set of 4,033 while keeping the others out of sight. Once P-Flash had wrapped up a study session, the team quizzed it on a set of 504 simulations, fine-tuned the model based on its grade, and repeated the process. After attaining the desired performance, the researchers put the system up against a final set of 504.
What Types of Data Annotation Were Required?
To better understand the types of data annotation required, let’s take a look at the image below:
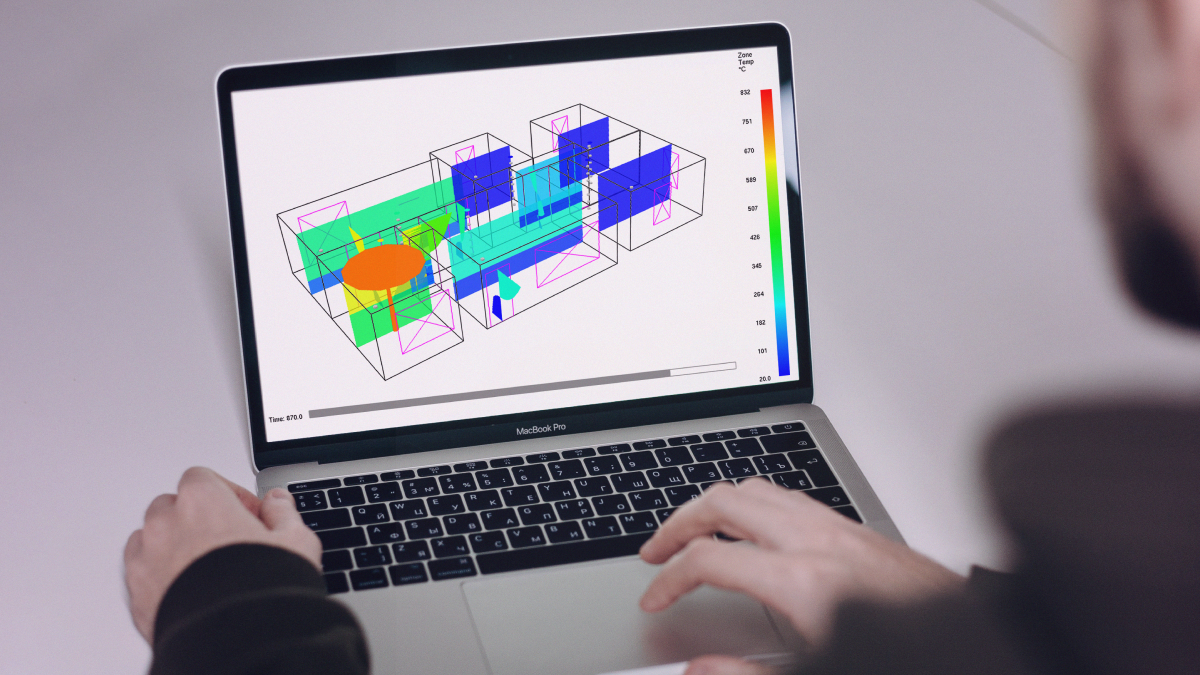
Here we see a virtual model of a house researchers use for simulating the fires. The first thing the data annotators need to do is a label where the windows and the doors are in each image, as well as whether or not they are open. Most likely, they would also need to label each piece of furniture in the house since this was one of the variables in the experiments. In addition to this, we see that the image is color-coded, where the areas with the highest temperatures are orange or red. The data annotators would need to use semantic segmentation to make sure that exactly the right parts of the images are covered with the corresponding color. With this type of 3D Point Cloud Annotation, accuracy and attention to detail are of critical importance since this will affect how the model operates.
This is why it is also important to have the needed QA processes and QA Team in place and also perform some data validation. Even if the data annotators were off by one pixel, this could cause big problems in the future.
Trust Mindy Support With All of Your 3D Point Cloud Annotation Needs
Mindy Support is a global company for data annotation and business process outsourcing, trusted by several Fortune 500 and GAFAM companies, as well as innovative startups. With nine years of experience under our belt and offices and representatives in Cyprus, Poland, Romania, The Netherlands, India, and Ukraine, Mindy Support’s team now stands strong with 2000+ professionals helping companies with their most advanced data annotation challenges.