Computer Vision Drones are Improving Crops Yields and Sustainability
Artificial intelligence-enabled drones are becoming widely used for smart farming, helping farmers with a variety of tasks from planning and analysis to planting crops and then monitoring the fields to ensure health and growth. When combined with drone technology, artificial intelligence can help farmers with all aspects of farming along the value chain. By analyzing aerial photos in greater detail, AI can provide farmers with information that can reduce planting costs, use less water and fertilizer, and monitor crop health.
In this article, we will take a look at how AI-powered drones are assisting farmers with a wide variety of tasks and the data annotation needed to train them.
Increasing Crop Yields
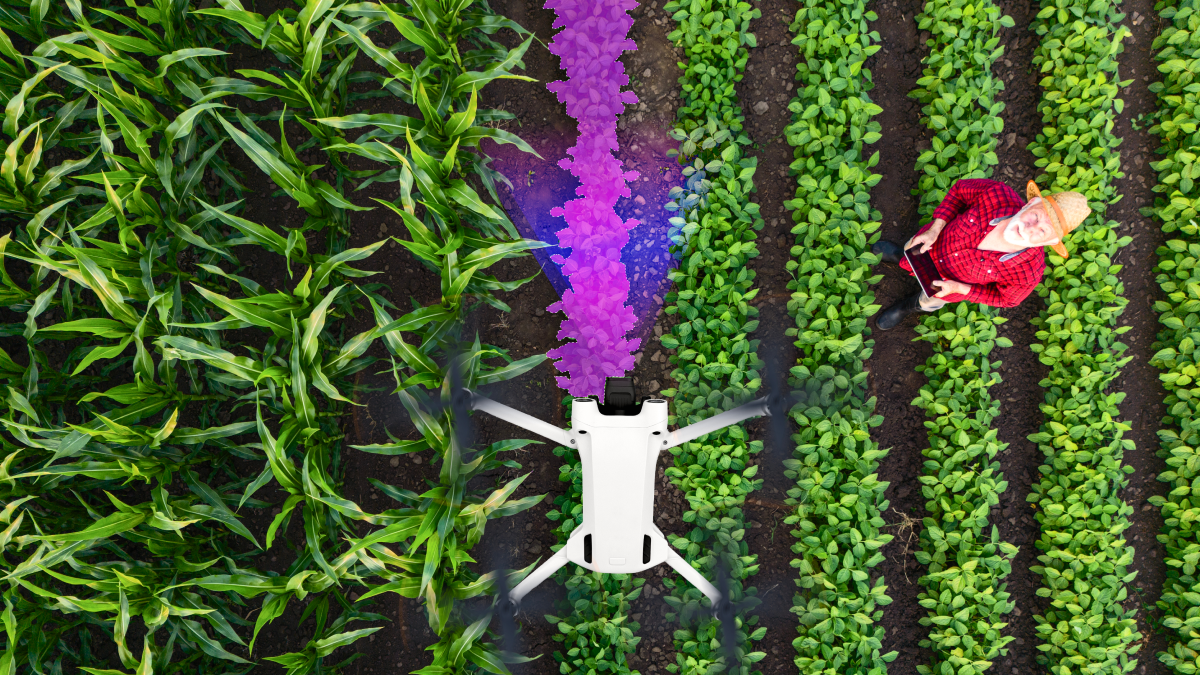
Farmers are constantly looking to increase the amount of marketable crop yields they can produce, both for financial and food security reasons. There will inevitably be differences in the size and quality of individual crops at harvest time due to the uneven growth of plants. Therefore, one of the top priorities for farmers is to determine when to harvest. A new method that heavily relies on artificial intelligence and drones improves this estimation in a measurable way by closely and accurately analyzing each crop to determine its likely growth characteristics.
The system requires minimal labor costs because the drones perform the imaging process repeatedly without the need for human intervention. Every plant in the field is identified and cataloged by drones, and the imaging data they collect powers a deep learning model that provides farmers with comprehensible visual data. The aspects of deep learning and image analysis present one of the major obstacles here. The process of gathering the image data is rather simple, but because of the way plants move in the wind and how the light varies with the seasons and over time, there is a lot of variation in the data that is difficult for machines to account for.
This is where high-quality data annotation can be of great value to help the AI system better understand the image data. We will go into greater detail about the types of data annotation needed for this type of AI in a later section.
Improving Sustainability
One of the ways AI drones are improving sustainability efforts is through a more efficient way of battling weeds. The standard approach would be to use a tractor that would spray herbicides throughout the entire farmland to zap weeds that are often tiny and scattered about. In addition to its detrimental effects on the environment, the traditional “spray it all” approach causes enormous financial waste. Today, more than ever, the issue of controlling weeds sustainably is critical. According to scientists, certain weeds that compete with crops for nutrients are growing faster as a result of global warming, endangering food security. However, each herbicide drop that farmers use has an adverse effect on the environment, contaminating drinking water and soil and contributing to the disastrous loss of biodiversity.
AI drones, powered with computer vision cameras, can distinguish between staple crops such as corn, wheat, soybeans, and weeds. The drone’s AI detects weeds with 96% accuracy and can “see” anything larger than half a sesame seed; it will only spray the targeted area.
What Types of Data Annotation are Needed to Train AI Drones?
AI-powered drones rely on computer vision to scan and distinguish all the various crops in the field. This means data annotation techniques like object detection will be necessary. This enables the learning of object detection and location estimation within a frame by models. The counting and tracking of objects in pictures or videos is a common application for these models. Rectangle labels, commonly referred to as bounding boxes, are typically needed by object detection models in order to annotate objects inside the frames.
In addition to this instance, segmentation will be needed, which is where the model gains the ability to recognize objects, locate each one in the frame, and calculate the precise pixel count of each one. If you require higher accuracy and more accurate pixel estimates for object interactions, these models may be helpful. Polygon labels are needed to annotate the distinct pixels that belong to an object in these kinds of models. AI-powered tools can be particularly useful in the tedious and time-consuming task of manually labeling polygons.
Trust Mindy Support With All of Your Data Annotation Needs
Mindy Support is a global provider of data annotation services and is trusted by Fortune 500 and GAFAM companies. With more than ten years of experience under our belt and offices and representatives in Cyprus, Poland, Romania, The Netherlands, India, OAE, and Ukraine, Mindy Support’s team now stands strong with 2000+ professionals helping companies with their most advanced data annotation challenges.