Applications of AI in Construction
Category: AI insights
Published date: 11.08.2023
Read time: 6 min
Construction companies at the forefront of innovation are already heavily utilizing machine learning and artificial intelligence-driven algorithms in their work, from determining the ideal concrete mix to building inspection. AI has shown promise as a technology that will revolutionize our sector. Understanding artificial intelligence and how it offers distinctive solutions to boost the productivity and performance of the construction sector is crucial as we move into this next phase of technology development.
In this article, we will take a look at some of the ways construction companies are using AI and the data annotation that’s required to create these technologies.
Improved Building Design
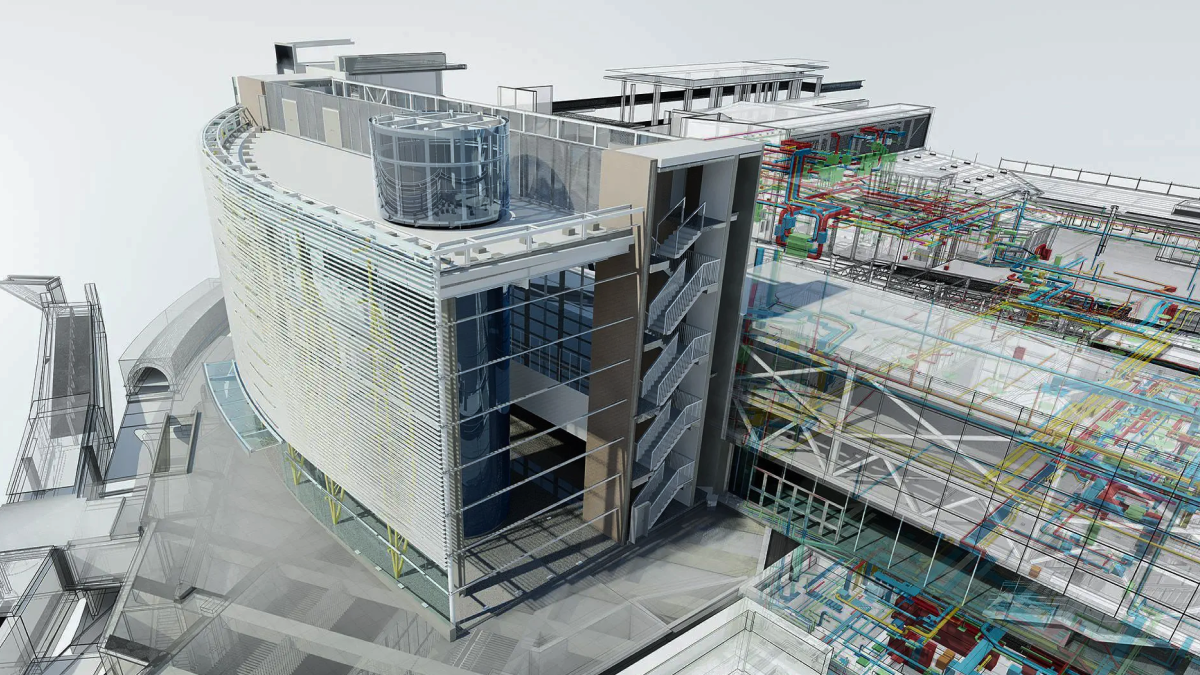
Building information modeling is a 3D model-based technique that provides architects, engineers, and construction workers with information they can use to effectively plan, design, build, and manage infrastructure and structures. The architecture, engineering, mechanical, electrical, and plumbing (MEP) plans, as well as the order of the various teams’ activities, must be considered in the 3D models when planning and designing a project’s construction. Making sure that the various models from the various sub-teams do not conflict with one another is the challenge.
To discover and resolve conflicts between the many models produced by the various teams, the industry leverages machine learning in the form of AI-powered generative design. This reduces the need for rework. There is software that explores every possible variation of a solution and generates design alternatives using machine learning algorithms. The generative design software develops 3D models suited for the limitations when the user enters their needs into the model. Iteratively creating models, it learns from each one until it finds the best one.
Enhancing Site Safety
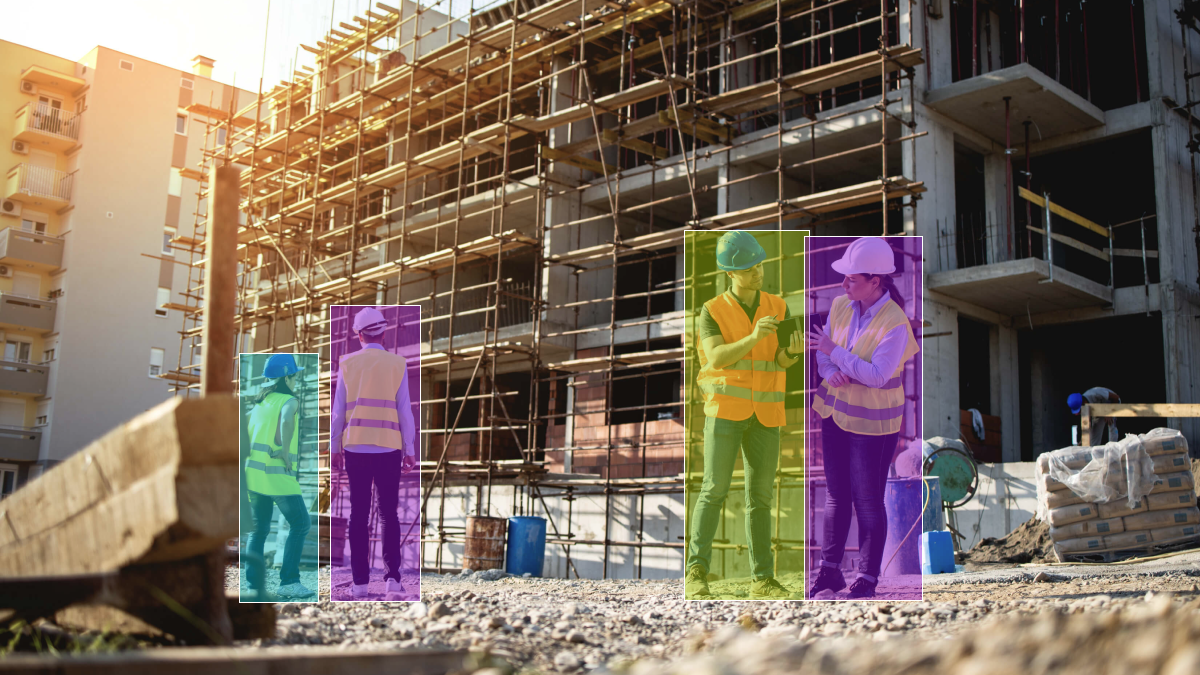
Even in conditions of high uncertainty, AI can track, detect, assess, and forecast potential hazards in terms of safety, quality, efficiency, and cost across teams and work areas. In order to capture the interdependencies of causes and accidents, measure the probability of failure, and assess the risk from both a qualitative and quantitative perspective, data from the construction site has been learned using a variety of AI methods, including probabilistic models, fuzzy theory, machine learning, neural networks, and others. They might get around the subjectivity and ambiguity of traditional risk assessments.
Using AI-based risk analysis, project managers can quickly prioritize potential risks and choose proactive rather than reactive actions for risk mitigation, such as streamlining job site operations, adjusting staffing levels, and maintaining projects on schedule and within budget. In complex procedures, AI provides early troubleshooting to prevent failure and mistakes. To lessen the number of people in danger on building sites, robots can do hazardous activities.
Monitoring the Pace of Construction
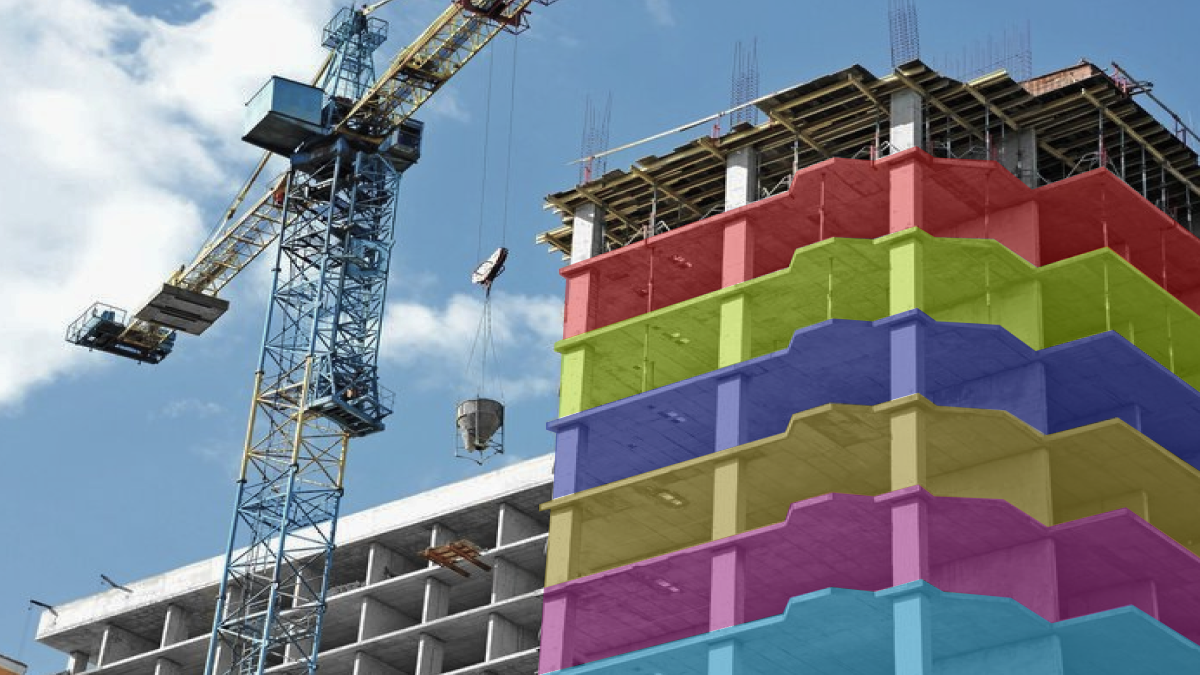
All construction stakeholders can follow construction projects and continuously evaluate their quality and progress using AI. Robots with computer vision cameras can independently traverse construction sites to collect 3D point clouds. After a digital model is complete, companies use a neural network to compare project data to BIM and bill of materials data. Project managers can better oversee large-scale projects with thousands of components thanks to the information gathered. The amount owed or if the budget is in jeopardy, the punctuality of projects, and the early identification of quality flaws that allow for repair and mitigation are some examples of these insights.
What Types of Data Annotation are Needed to Train These Technologies?
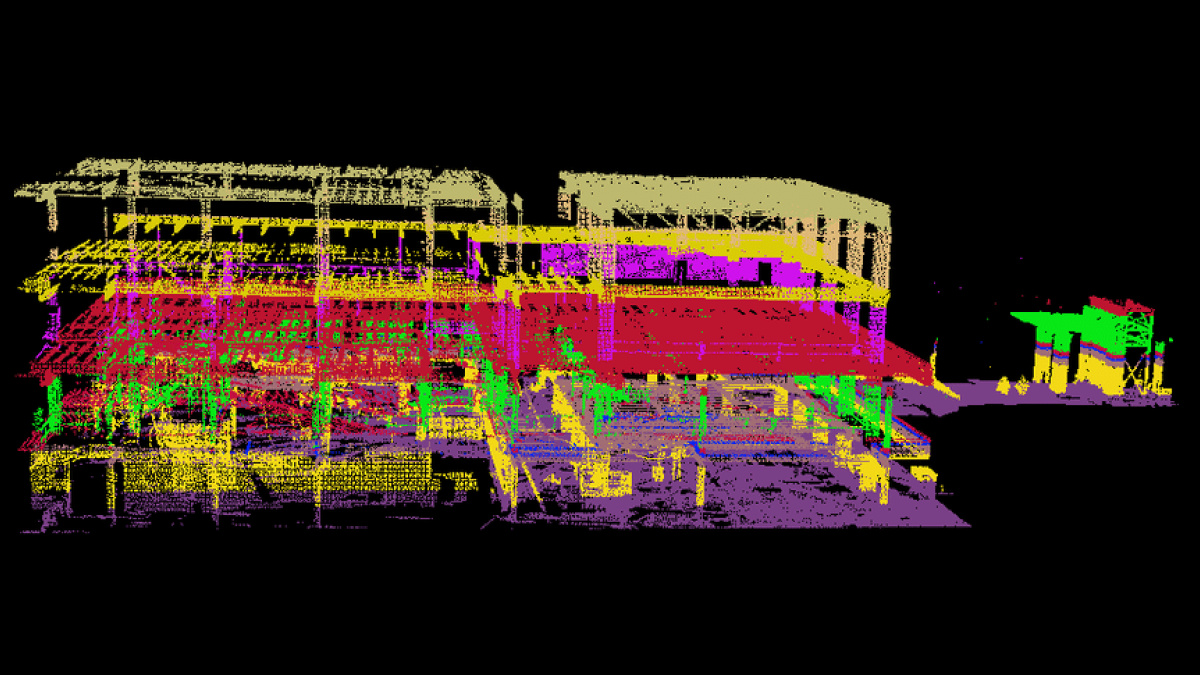
In order to create technologies to monitor construction projects and can improve building design, 3D Point Cloud annotation is needed. As 3D annotation allows for depth and volume perception, it can be used in a variety of industries. It is much more difficult to annotate than 2D data, but it provides much better insights when 2D visual data is insufficient. Data annotation types such as cuboids for object recognition provide a way to identify the size, shape, and location of objects in the point cloud data. Also, semantic segmentation is a computer vision task in which the goal is to categorize each pixel in an image into a class or object. The goal is to produce a dense pixel-wise segmentation map of an image, where each pixel is assigned to a specific class or object.
Data annotation for computer vision is also necessary for site safety technology. This covers topics like instance segmentation, where models learn to recognize objects, pinpoint each one’s precise placement inside the frame, and estimate its number of pixels. These models can be helpful if you require more accuracy and more exact pixel estimations for object interactions.
Trust Mindy Support With All of Your Data Annotation Needs
Mindy Support is a global provider of data annotation services and is trusted by Fortune 500 and GAFAM companies. With more than ten years of experience under our belt and offices and representatives in Cyprus, Poland, Romania, The Netherlands, India, OAE, and Ukraine, Mindy Support’s team now stands strong with 2000+ professionals helping companies with their most advanced data annotation challenges.